A robust surrogate model of a solid oxide cell based on an adaptive polynomial approximation method
International Journal of Hydrogen Energy(2020)
摘要
Surrogate models that predict the behaviors of solid oxide cells (SOCs) accurately at low computational cost are crucial to the control and optimization of SOC plants. Lumped physical models of SOCs, while widely used in such applications, lack accuracy because of neglected physical details. Data-driven models are the other options of surrogate models, which are proved to be more accurate because these models are identified directly from experiments or numerical simulations. However, due to the time cost of experiments and numerical simulations of SOCs, it is hoped that data-driven models can be constructed from a minimum amount of data. Also, the trained data-driven models should be robust, in other words, insensitive to the data set as well as the initial settings. These requirements are hard to be achieved by existing data-driven models of SOCs, such as lookup tables and artificial neural networks (ANNs). Aiming to preserve robustness and reduce the required amount of data, this paper introduces an adaptive polynomial approximation (APA) method, which is derived from the latest findings of numerical computation science, to the surrogate modeling of SOCs. The obtained models by the APA method are validated by both experiments and simulations. By analyzing the models, the coupling relationship among operating parameters of SOCs is revealed. The physical interpretability makes the APA method distinctive from common data-driven modeling methods. Performance comparison shows that the APA method is more accurate and robust than the existing ones with similar sampling costs. Additionally, the APA method can control the accuracy of the model by setting an error criterion in the algorithm iteration, endowing the APA method with an error control ability as per different accuracy requirements for SOC modeling.
更多查看译文
关键词
Solid oxide cell,Modeling,Adaptive polynomial approximation,Experiments,Data-driven
AI 理解论文
溯源树
样例
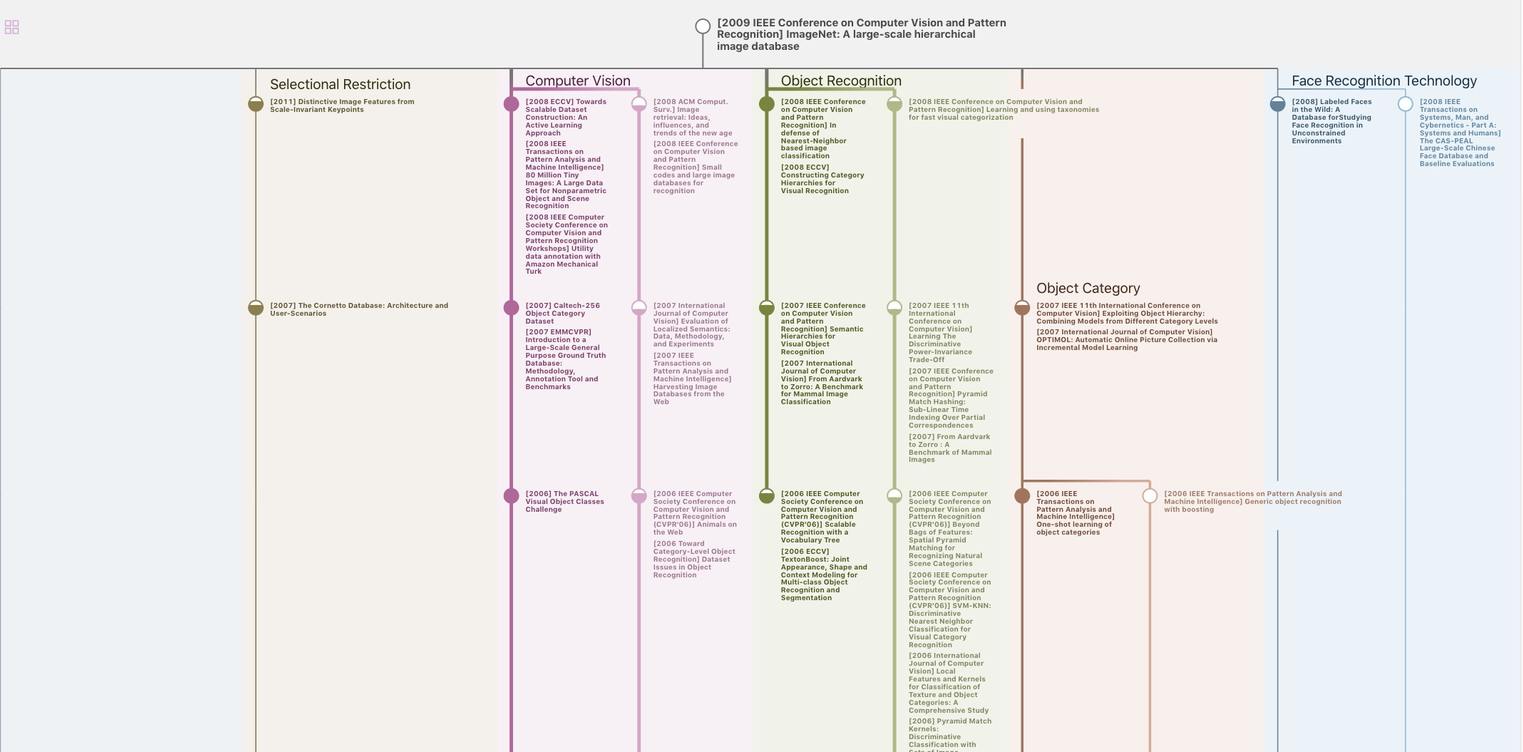
生成溯源树,研究论文发展脉络
Chat Paper
正在生成论文摘要