Modeling Charged-Particle Multiplicity Distributions At Lhc
MODERN PHYSICS LETTERS A(2020)
摘要
With many applications in high-energy physics, Deep Learning or Deep Neural Network (DNN) has become noticeable and practical in recent years. In this article, a new technique is presented for modeling the charged particles multiplicity distribution Pn of Proton-Proton (PP) collisions using an efficient DNN model. The charged particles multiplicity n, the total center of mass energy root s, and the pseudorapidity eta used as input in DNN model and the desired output is P-n. DNN was trained to build a function, which studies the relationship between P-n (n, root s, eta). The DNN model showed a high degree of consistency in matching the data distributions. The DNN model is used to predict with P-n not included in the training set. The expected P-n had effectively merged the experimental data and the values expected indicate a strong agreement with Large Hadron Collider (LHC) for ATLAS measurement at root s = 0.9, 7 and 8 TeV.
更多查看译文
关键词
Charged particles multiplicity distribution, charged particles multiplicity, the total center of mass energy, and the pseudorapidity, deep neural network
AI 理解论文
溯源树
样例
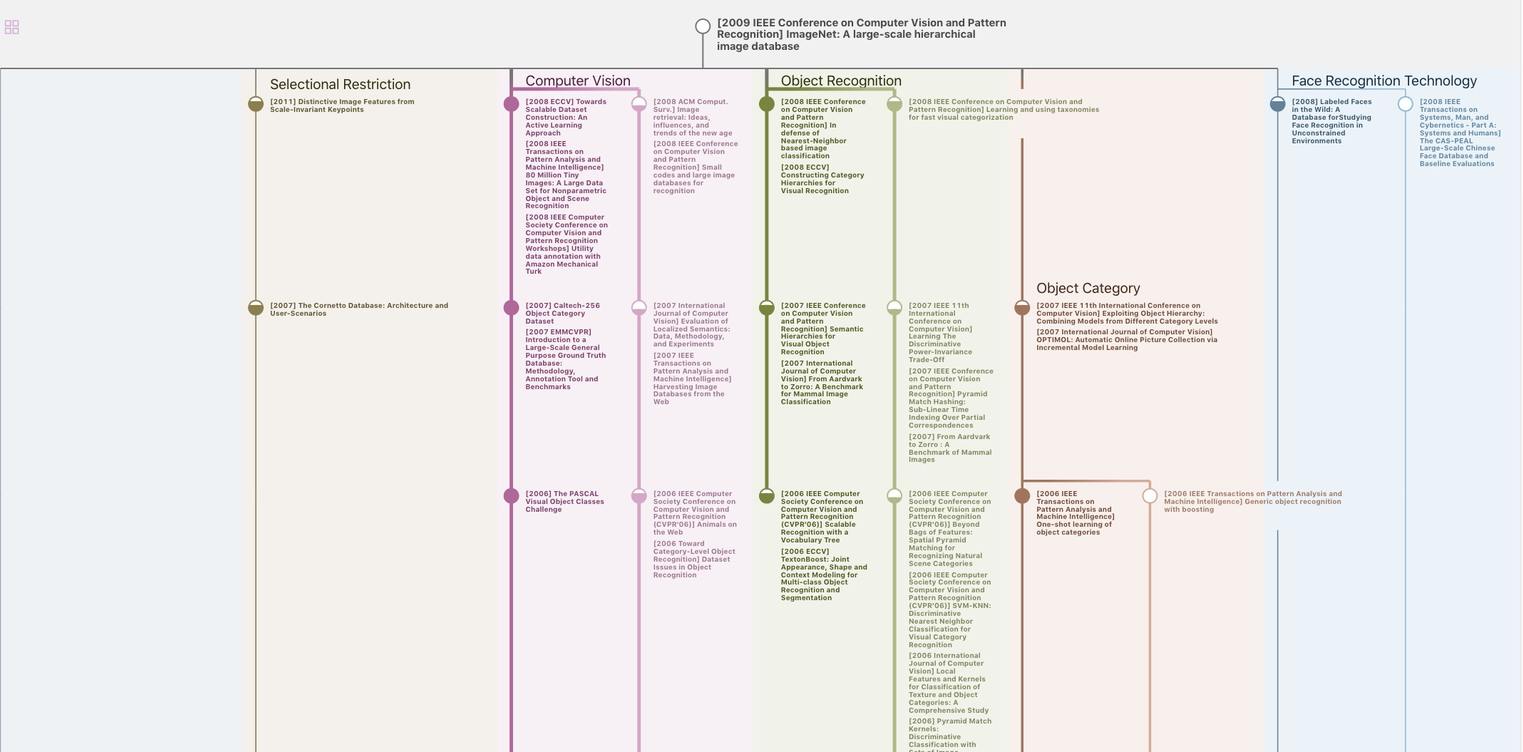
生成溯源树,研究论文发展脉络
Chat Paper
正在生成论文摘要