Estimation of daily maize transpiration using support vector machines, extreme gradient boosting, artificial and deep neural networks models
Agricultural Water Management(2021)
摘要
Accurate measurement or estimation of plant transpiration (T) is of great significance for understanding crop water use, predicting crop yield and designing irrigation schedule in agricultural production. Nevertheless, direct measurement of T is difficult, expensive, destructive and time-consuming. This study explored the applicability of support vector machines (SVM), extreme gradient boosting (XGBoost), single-layer artificial neural networks (ANN) and deep neural networks (DNN) models for estimating daily T of summer maize in Northwest China. Four input combinations of meteorological (Tmax, Tmin, RH, U and Rs), soil (SWC) and crop (LAI) variables were employed to explore the effects of various variables on daily T estimation. The whole dataset during the four maize growing seasons of 2015–2018 were randomly divided into two subsets: 80% for model training and 20% for model testing. The results showed that the performances of the four machine learning models improved by 10.1–13.1%, 15.7–23.8% and 36.1–46.2% in terms of RMSE during testing when SWC (0.556–0.675 mm d−1), LAI (0.477–0.621 mm d−1) and both of them (0.344–0.482 mm d−1) were further incorporated respectively, compared to models with only meteorological variables as inputs (0.621–0.754 mm d−1). DNN models (R2 = 0.816–954, RMSE = 0.344–0.621 d−1, MAE = 0.256–0.445 mm d−1 and NSE = 0.902–0.972) slightly outperformed SVM models (R2 = 0.731–0.948, RMSE = 0.370–0.688 mm d−1, MAE = 0.286–0.500 mm d−1 and NSE = 0.880–0.967) during testing, followed by XGBoost models (R2 = 0.739–0.929, RMSE = 0.455–0.721 mm d−1, MAE = 0.355–0.519 mm;d−1 and NSE = 0.872–0.947) and ANN models (R2 = 0.683–0.913, RMSE = 0.482–0.754 mm d−1, MAE = 0.368–0.545 mm d−1 and NSE = 0.840–0.936) under the four input combinations. The incorporation of SWC or/and LAI in the machine learning models is highly recommended for accurate daily maize T estimation. The DNN model is more effective for daily maize T estimation due to its advantage in modeling high-order complex relationships between T and its driving variables through multiple levels of feature abstraction.
更多查看译文
关键词
Transpiration,Support vector machines,Extreme gradient boosting,Artificial neural networks,Deep neural networks
AI 理解论文
溯源树
样例
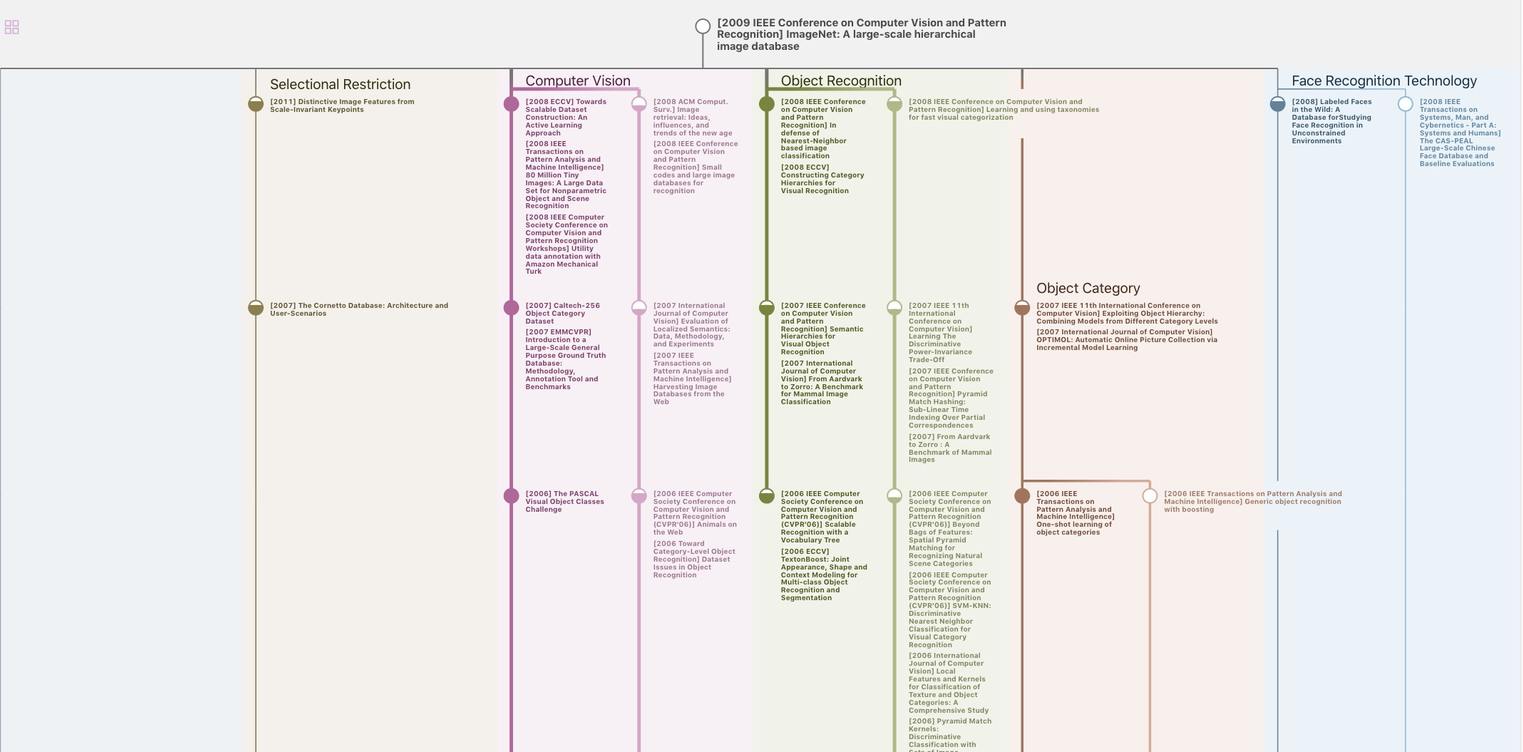
生成溯源树,研究论文发展脉络
Chat Paper
正在生成论文摘要