An experimental approach to evaluate machine learning models for the estimation of load distribution on suspension bridge using FBG sensors and IoT
Computational Intelligence(2022)
Abstract
Most of the tragedies on any bridge structure have been the cause of high-density crowd behavior as a response to trampling as well as the crushing scenario. Therefore, it is most important to monitor such unforeseen situations by sensing the load imposed on the bridge structures. This scenario may arise where crowd movement is huge on these types of bridges. Similarly, the fiber Bragg grating (FBG) is a promising technology for structural health monitoring applications. In this work, an Internet of Things based FBG optical sensing scheme is proposed to monitor real-time strain distribution throughout the bridge structures and localization of load imposed on the structure from a central control room. A suspension bridge model is designed by referring to a real bridge scenario and these FBG sensors are deployed to validate the proposed machine learning models. In this article, the performances of two machine learning strategies are discussed for the accurate estimation of load and its position by acquiring high sensitive FBG sensors signals at a very high data rate. The algorithms include K-nearest neighbor (KNN) and random forest (RF); which are applied on each sensing data source, and then validated using a prototype suspension bridge model integrated with three FBG sensors (1532 nm, 1538 nm, and1541 nm) on a single optical fiber cable.
MoreTranslated text
Key words
FBG Sensor, Internet of Things, K-Nearest Neighbor, Random Forest, Structural Health Monitoring
AI Read Science
Must-Reading Tree
Example
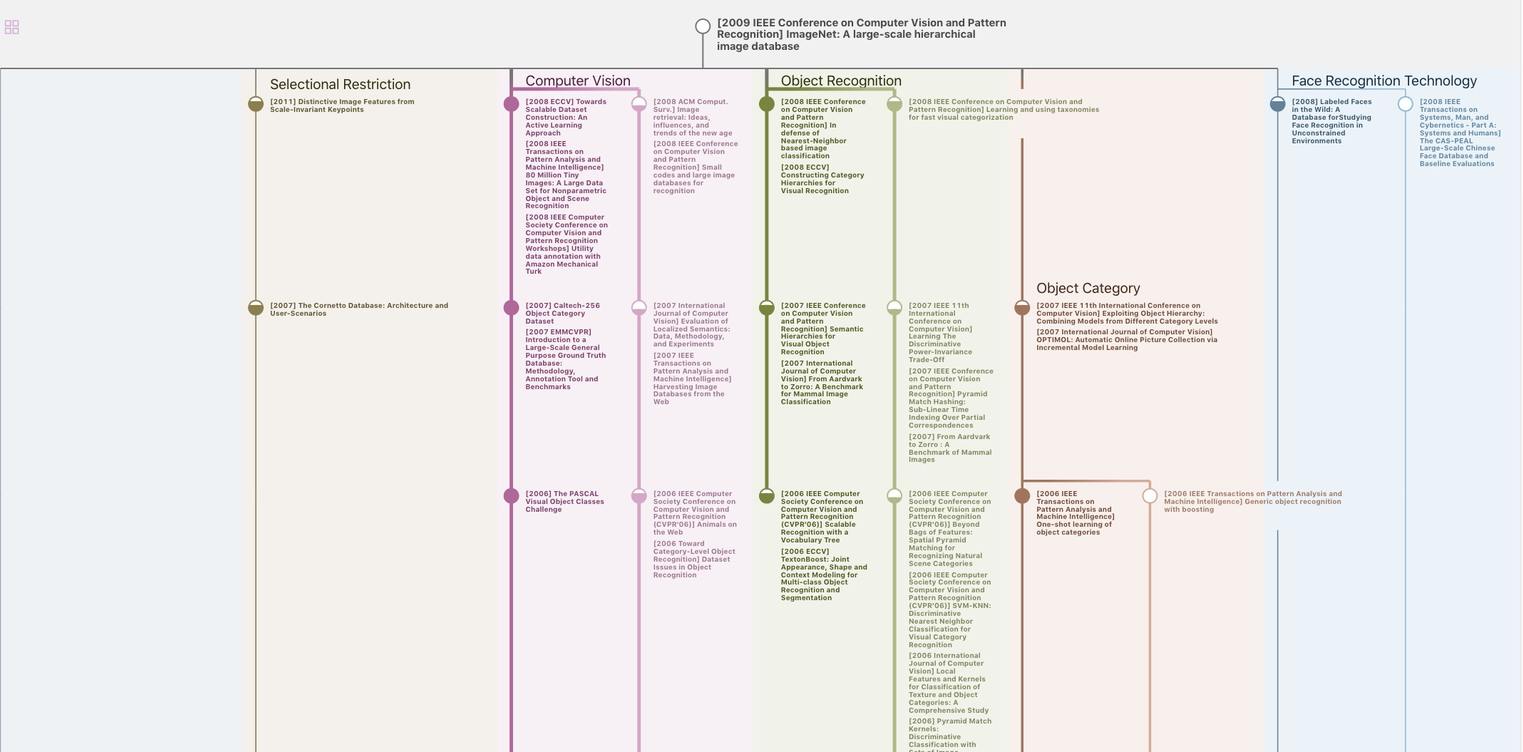
Generate MRT to find the research sequence of this paper
Chat Paper
Summary is being generated by the instructions you defined