Joint Representation Learning and Clustering: A Framework for Grouping Partial Multiview Data
IEEE Transactions on Knowledge and Data Engineering(2022)
摘要
Partial multi-view clustering has attracted various attentions from diverse fields. Most existing methods adopt separate steps to obtain unified representations and extract clustering indicators. This separate manner prevents two learning processes to negotiate to achieve optimal performance. In this paper, we propose the Joint Representation Learning and Clustering (JRLC) framework to address this issue. The JRLC framework employs representation matrices to extract view-specific clustering information directly from the presence of partial similarity matrices, and rotates them to learn a common probability label matrix simultaneously, which connects representation learning and clustering seamlessly to achieve better clustering performance. Under the guidance of JRLC framework, several new incomplete multi-view clustering methods can be developed by extending existing single-view graph-based representation learning methods. For illustration, within the framework, we propose two specific methods, JRLC with spectral embedding (JRLC-SE) and JRLC via integrating nonnegative embedding and spectral embedding (JRLC-NS). Two iterative algorithms with guaranteed convergence are designed to solve the resultant optimization problems of JRLC-SE and JRLC-NS. Experimental results on various datasets and news topic clustering application demonstrate the effectiveness of the proposed algorithms.
更多查看译文
关键词
Representation learning,clustering,partial multi-view data,graph
AI 理解论文
溯源树
样例
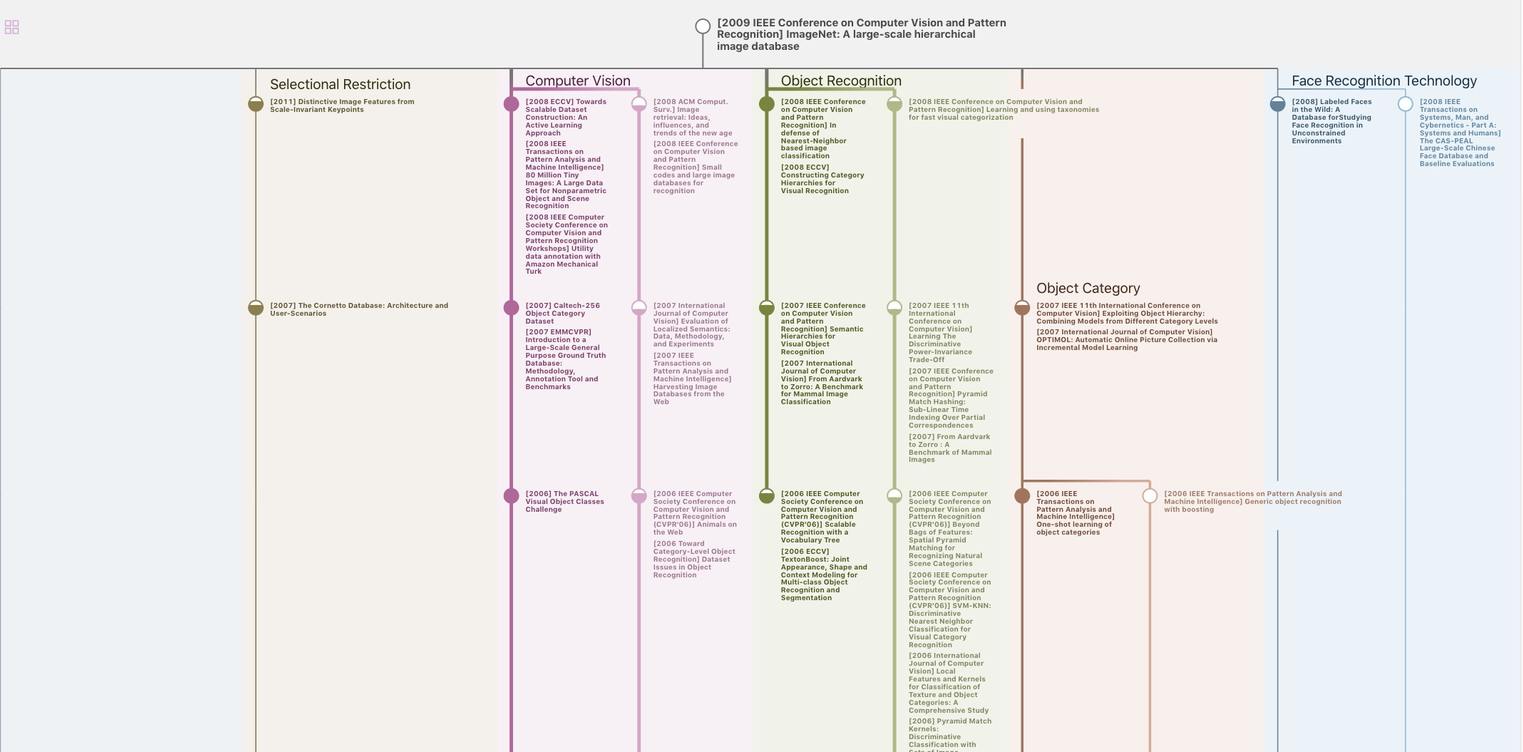
生成溯源树,研究论文发展脉络
Chat Paper
正在生成论文摘要