A Spiking Recurrent Neural Network with Phase Change Memory Synapses for Decision Making
ISCAS(2020)
摘要
Neuronal activity of recurrent neural networks (RNNs) experimentally observed in the hippocampus is widely believed to play a key role for mammalian ability to associate concepts and make decisions. For this reason, RNNs have rapidly gained strong interest as computational enabler of brain-inspired cognitive functions in hardware. From the technology viewpoint, nonvolatile memory devices such as phase change memory (PCM) and resistive switching memory (RRAM) have become a key asset to allow for high synaptic density and biorealistic cognitive functionality. In this work, we demonstrate for the first time associative learning and decision making in a hardware Hopfield RNN with 6 spiking neurons and PCM synapses via storage, recall and competition of attractor states. We also experimentally demonstrate the solution of a constraint satisfaction problem (CSP) namely a Sudoku with size 2x2 in hardware and 9x9 in simulation. These results support spiking RNNs with PCM devices for the implementation of decision making capabilities in hardware neuromorphic systems.
更多查看译文
关键词
phase change memory (PCM),Hebbian learning,spiking recurrent neural network (RNN),associative memory,decision making,constraint satisfaction problems (CSPs)
AI 理解论文
溯源树
样例
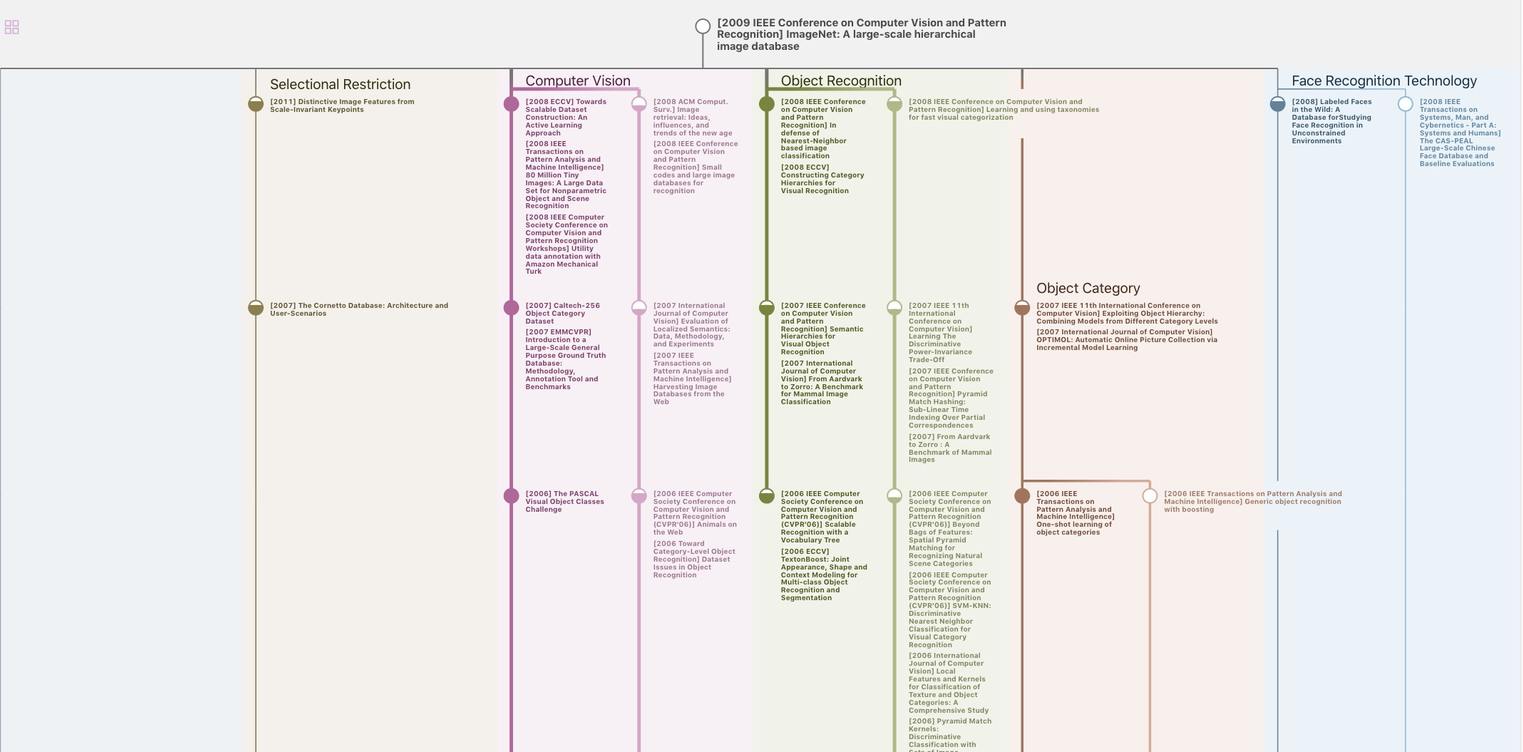
生成溯源树,研究论文发展脉络
Chat Paper
正在生成论文摘要