Adaptive Magnetic Anomaly Detection Method Using Support Vector Machine
IEEE GEOSCIENCE AND REMOTE SENSING LETTERS(2022)
Abstract
Magnetic anomaly detection (MAD) has been widely used for detecting the magnetic targets in many areas. In low signal-to-noise ratio (SNR) situations, the magnetic anomaly is usually buried in the magnetic noise, which leads to a decrease in the detection performance of traditional MAD methods. In order to improve the detection probability of MAD in low SNR, we propose an adaptive method of MAD using support vector machine (SVM). First, we design a detection model using SVM with radial basis function (RBF) kernel function. Meanwhile, we analyze the magnetic anomaly signal and determined the orthonormal basis function (OBF) energy and magnetic entropy as the features of the magnetic anomaly, which are as the input of the model. Then, the model is trained based on the data set with the magnetic signals. Finally, the trained model is applied to the raw magnetic signal to detect the anomaly. Compared to the traditional methods, the proposed method has a better performance and adaptive ability. The proposed method is validated by the real magnetic noise. The results show that the proposed method can reduce the case of false alarm and improve the detection performance in low SNR.
MoreTranslated text
Key words
Magnetometers, Entropy, Magnetic resonance, Support vector machines, Signal to noise ratio, Adaptation models, Magnetic noise, Features selection, magnetic anomaly detection (MAD), magnetic entropy, orthonormal basis function (OBF) energy, support vector machine (SVM)
AI Read Science
Must-Reading Tree
Example
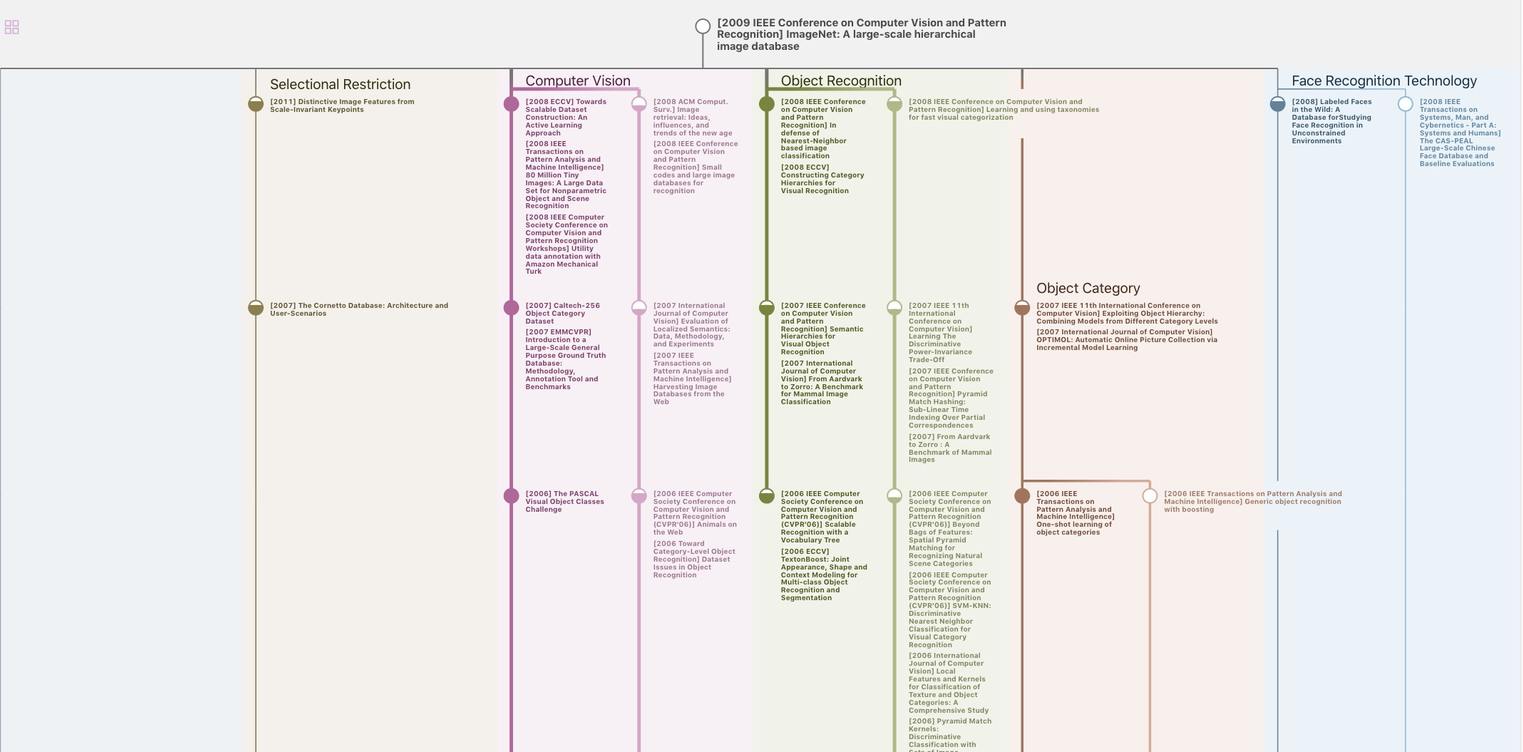
Generate MRT to find the research sequence of this paper
Chat Paper
Summary is being generated by the instructions you defined