Remote Sensing Image Classification Based on a Cross-Attention Mechanism and Graph Convolution
IEEE GEOSCIENCE AND REMOTE SENSING LETTERS(2022)
Abstract
An attention mechanism assigns different weights to different features to help a model select the features most valuable for accurate classification. However, the traditional attention mechanism algorithm often allocates weights in a one-way fashion, which can result in a loss of feature information. To obtain better hyperspectral data classification results, a novel cross-attention mechanism and graph convolution integration algorithm are proposed in this letter. First, principal component analysis is used to reduce the dimensionality of hyperspectral images to obtain low-dimensional features that are more expressive. Second, the model uses a cross (horizontal and vertical directions) attention algorithm to allocate weights jointly based on its two strategies; then, it adopts a graph convolution algorithm to generate the directional relationships between the features. Finally, the generated deep features and the relationship between the deep features are used to complete the prediction of hyperspectral data. Experiments on three well-known hyperspectral data setsx2014;Indian Pines, the University of Pavia, and Salinasx2014;show that the proposed algorithm achieves better performances than do other well-known algorithms using different methods of training set division.
MoreTranslated text
Key words
Convolution, Hyperspectral imaging, Training, Feature extraction, Principal component analysis, Cross-attention mechanism (CAM), deep learning, graph convolution
AI Read Science
Must-Reading Tree
Example
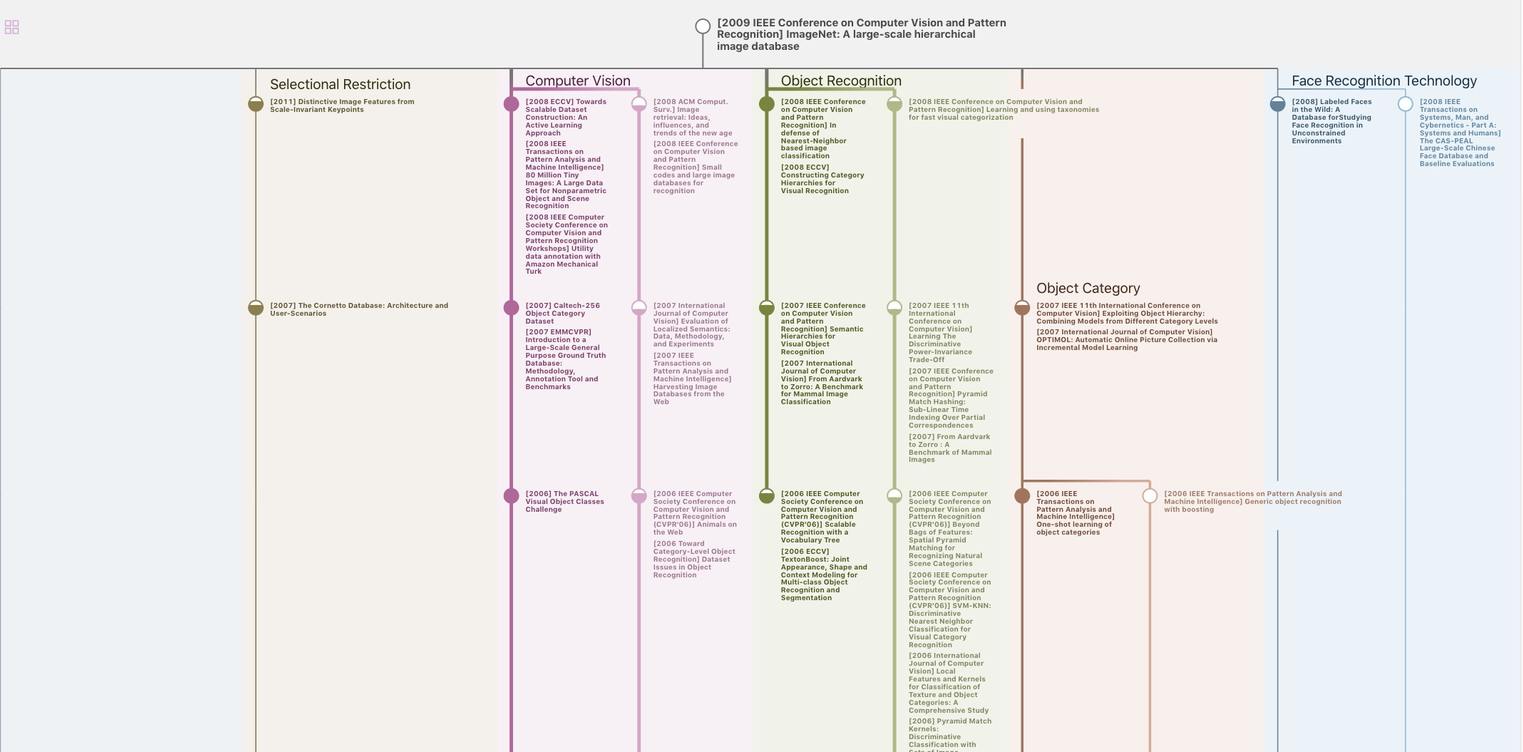
Generate MRT to find the research sequence of this paper
Chat Paper
Summary is being generated by the instructions you defined