Improved Generative Adversarial Networks for VHR Remote Sensing Image Classification
IEEE GEOSCIENCE AND REMOTE SENSING LETTERS(2022)
摘要
With increasing spatial resolution of remote sensing images, accurate classification of land classes depends more on the number of labeled samples. However, the acquisition of labeled samples is difficult and time-consuming. Hence, generative adversarial networks (GANs) have become a new method for collecting training samples for very-high-resolution (VHR) remote sensing image classification. A traditional GAN generates new samples with the same distribution as the labeled samples. However, the generated samples have features close to their class center, and the network cannot obtain effective discriminative ability for the samples close to the decision boundary. This letter presents an improved GAN (IGAN) for VHR remote sensing image classification. In the proposed framework, the generator aims to generate synthetic samples close to the classification boundary, and the discriminator aims to constrain the labels of the synthetic samples. The obtained synthetic samples can effectively improve the classification accuracy of the classification boundary. Experiments are conducted on two VHR remote sensing images, and the results show that the proposed method performs better than several state-of-the-art methods.
更多查看译文
关键词
Training, Remote sensing, Gallium nitride, Generators, Generative adversarial networks, Sensors, Spatial resolution, Generative adversarial network (GAN), remote sensing image classification, semisupervised learning
AI 理解论文
溯源树
样例
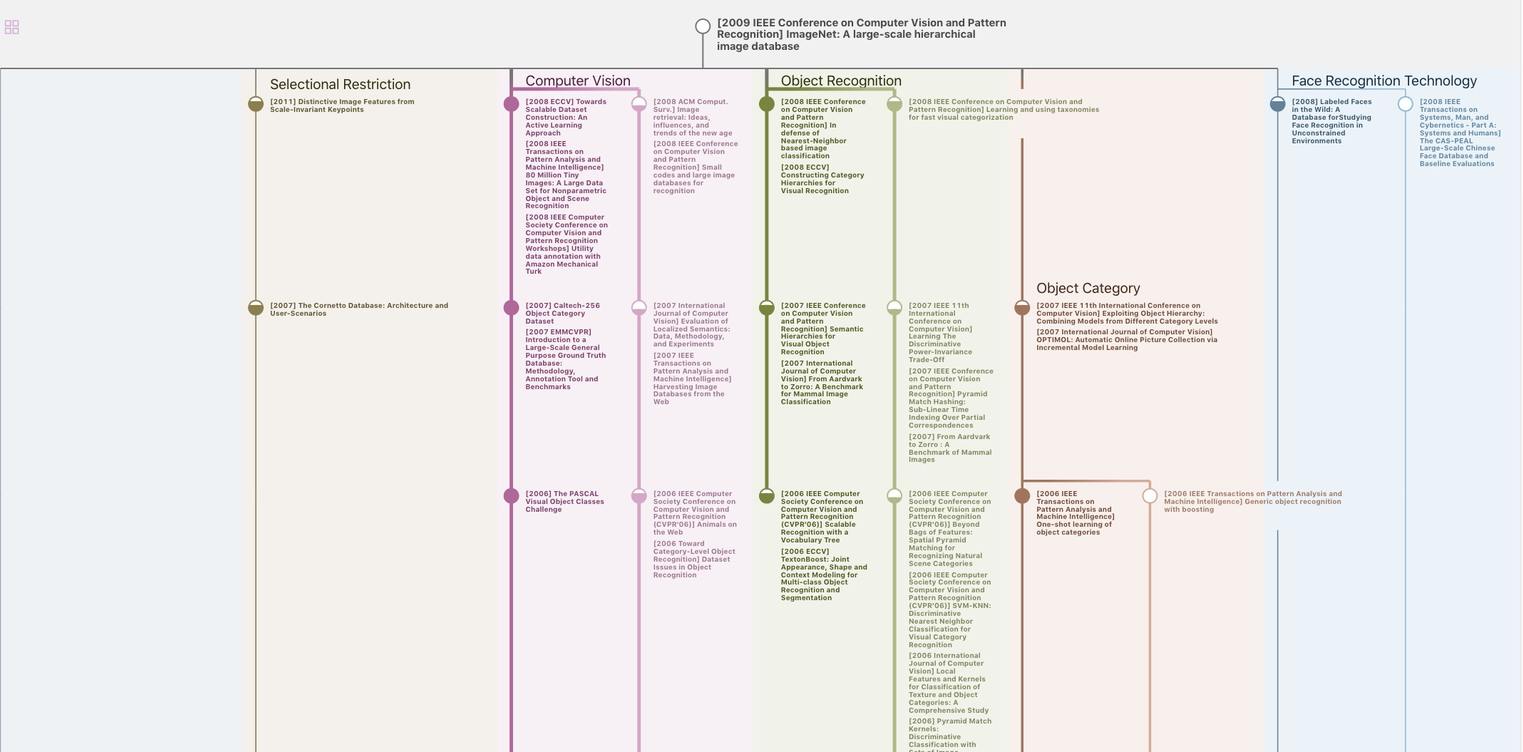
生成溯源树,研究论文发展脉络
Chat Paper
正在生成论文摘要