Residue Buildup Predictive Modeling For Stencil Cleaning Profile Decision-Making Using Recurrent Neural Network
ROBOTICS AND COMPUTER-INTEGRATED MANUFACTURING(2021)
摘要
This research proposes a novel framework to control the stencil cleaning profile selection in the stencil printing process (SPP). The SPP is a major contributor to yield loss in surface mount technology (SMT). Enhancement in SPP performance is critical to improving the printed circuit board (PCB) assembly line. The selection of a solvent-based or a dry-based cleaning profile is challenging, but the choice determines the effectiveness and efficiency of the stencil cleaning operation. The amount of residue buildup under the stencil is the main criterion used to decide the appropriate cleaning profile in SPP. In this research, a multi-dimensional temporal recurrent neural network (RNN) approach is used to accurately predict the amount of residue buildup on the underneath surface of the stencil in real-time. Specifically, the long short-term memory (LSTM) architecture is trained using actual residue buildup data. The proposed LSTM prediction model is compared with other state-of-the-art regression models such as multilayer perceptron (MLP) and ensemble learning models. Experimental results show the proposed LSTM model outperforms the state-of-the-art regression models and accurately predicts the stencil status. The proposed research aids decision-makers in the SPP line to select the appropriate stencil cleaning profile adaptively and in real-time. As a result, the overall SPP performance is improved.
更多查看译文
关键词
Stencil printing process, Cleaning profile selection, Recurrent neural network (RNN), Long short-term memory (LSTM)
AI 理解论文
溯源树
样例
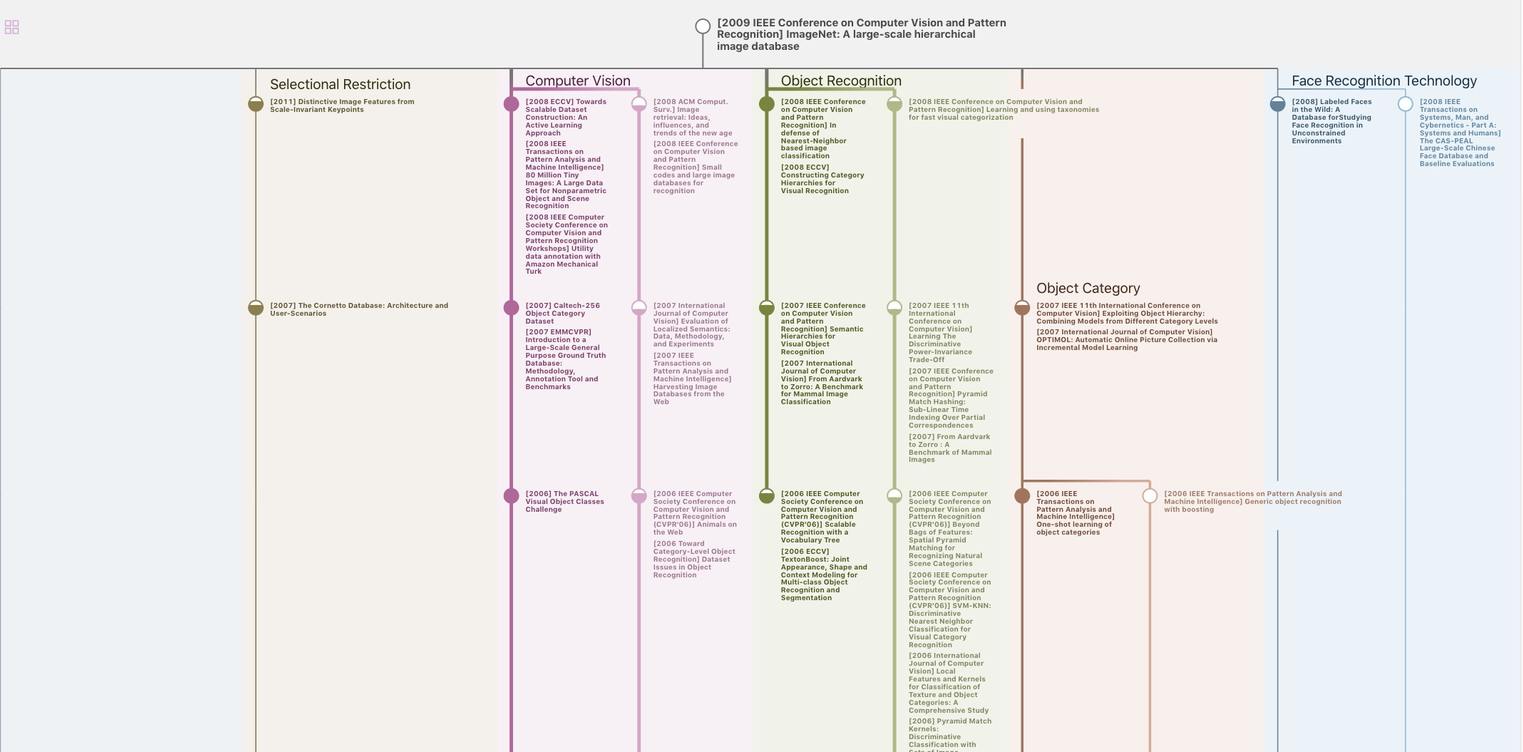
生成溯源树,研究论文发展脉络
Chat Paper
正在生成论文摘要