Wind Speed Forecasting Based On Extreme Gradient Boosting
IEEE ACCESS(2020)
摘要
As the integration of wind power into electrical energy network increasing, accurate forecast of wind speed becomes highly important in the case of large-scale wind power connected into the grid. In order to improve the accuracy of wind speed forecast and the generalization ability of the model, Extreme Gradient Boosting (XGBoost) as an improvement from gradient boosting decision tree (GBDT) is trained and deployed in the cheaper central processing unit (CPU) devices instead of graphics processing unit (GPU) devices, thus, a wind speed forecast model based on Extreme Gradient Boosting is proposed in this paper. Firstly, the historical data is taken as a part of the input vectors for the model. Moreover, considering the monthly change of wind speed characteristics, the dataset of wind power is divided into four parts by month so that the models are constructed in different complexity by month. Finally, compared with back propagation neural networks (BPNN) and linear regression (LR) models, the experimental results show that the improved XGBoost model can promote the forecast accuracy effectively.
更多查看译文
关键词
Predictive models, Wind speed, Data models, Forecasting, Autoregressive processes, Wind forecasting, Machine learning, Short-term wind speed forecasting, XGBoost, time series, historical characteristics, power grid
AI 理解论文
溯源树
样例
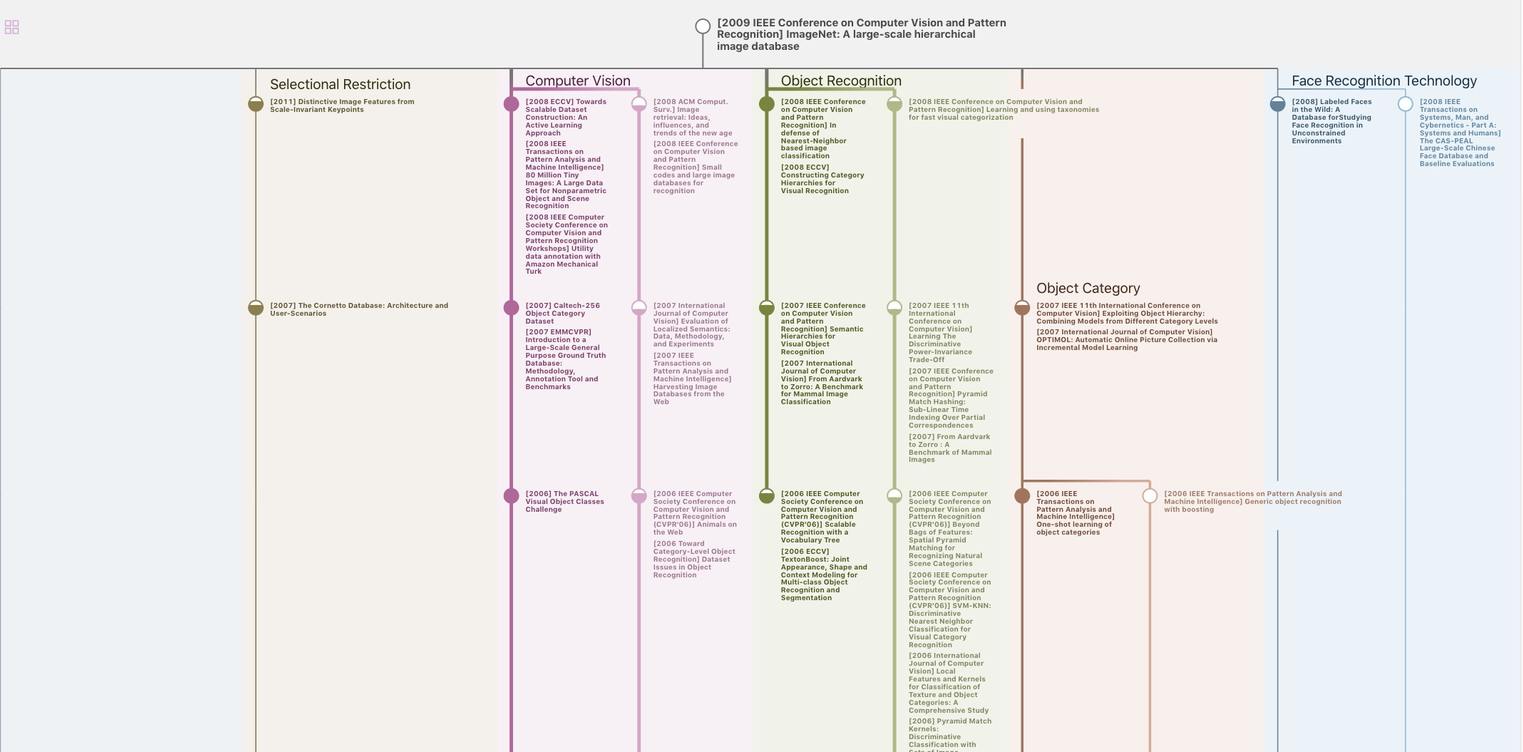
生成溯源树,研究论文发展脉络
Chat Paper
正在生成论文摘要