Multimodal Multiobject Tracking By Fusing Deep Appearance Features And Motion Information
COMPLEXITY(2020)
Abstract
Multiobject Tracking (MOT) is one of the most important abilities of autonomous driving systems. However, most of the existing MOT methods only use a single sensor, such as a camera, which has the problem of insufficient reliability. In this paper, we propose a novel Multiobject Tracking method by fusing deep appearance features and motion information of objects. In this method, the locations of objects are first determined based on a 2D object detector and a 3D object detector. We use the Nonmaximum Suppression (NMS) algorithm to combine the detection results of the two detectors to ensure the detection accuracy in complex scenes. After that, we use Convolutional Neural Network (CNN) to learn the deep appearance features of objects and employ Kalman Filter to obtain the motion information of objects. Finally, the MOT task is achieved by associating the motion information and deep appearance features. A successful match indicates that the object was tracked successfully. A set of experiments on the KITTI Tracking Benchmark shows that the proposed MOT method can effectively perform the MOT task. The Multiobject Tracking Accuracy (MOTA) is up to 76.40% and the Multiobject Tracking Precision (MOTP) is up to 83.50%.
MoreTranslated text
Key words
Multiple Object Tracking,Object Tracking,Object Detection,Motion Detection,Visual Tracking
AI Read Science
Must-Reading Tree
Example
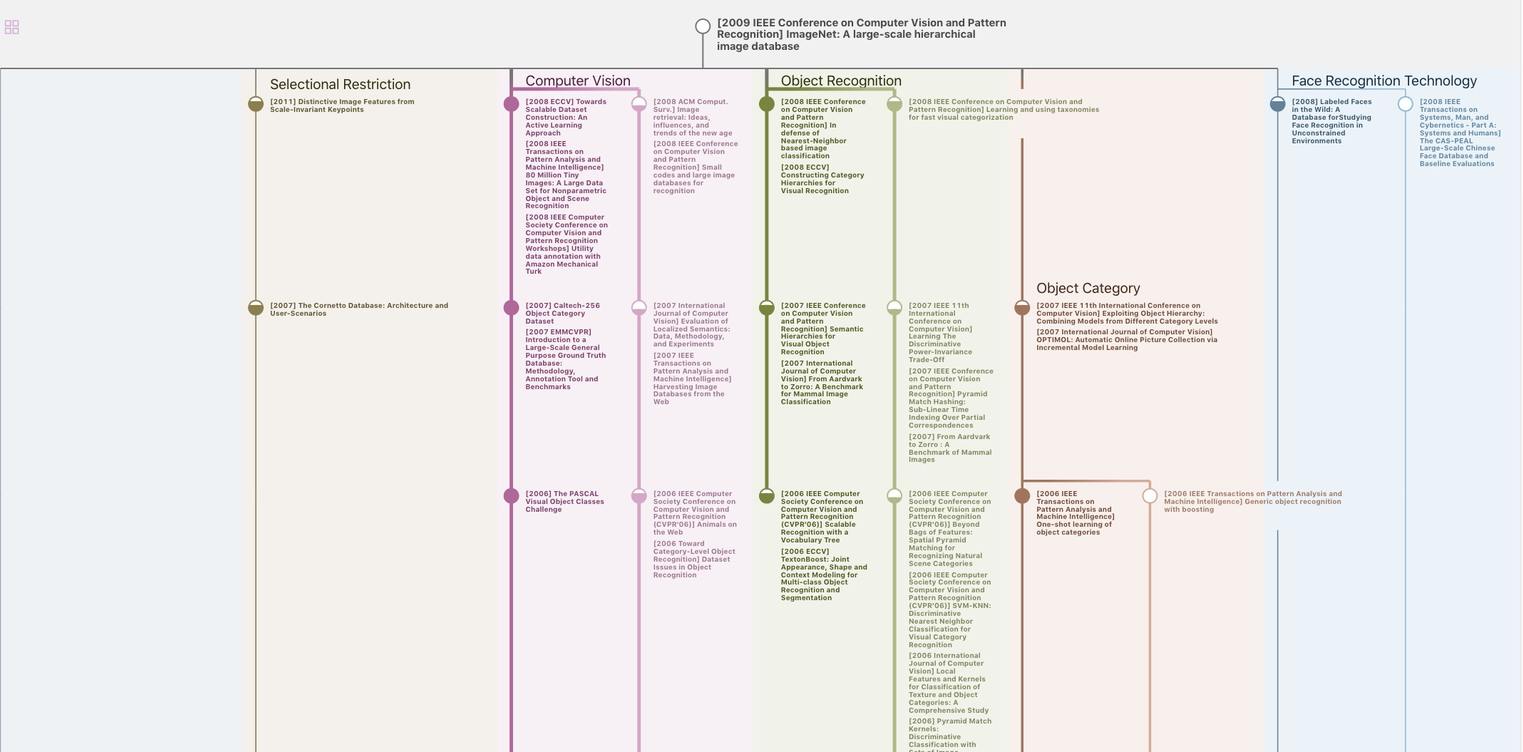
Generate MRT to find the research sequence of this paper
Chat Paper
Summary is being generated by the instructions you defined