Improving Cloud Type Classification of Ground-Based Images Using Region Covariance Descriptors
Atmospheric Measurement Techniques Discussions(2020)
摘要
Abstract. Cloud types are important indicators of cloud characteristics and short-term weather forecasting. The meteorological researchers can benefit from the automatic cloud type recognition of massive images captured by the ground-based imagers. However, by far it is still of huge challenge to design a powerful discriminative classifier for cloud categorization. To tackle this difficulty, in this paper, we present an improved method with region covariance descriptors (RCovDs) and Riemannian Bag-of-Feature (BoF). RCovDs model the correlations among different dimensional features, that allows for a more discriminative representation. BoF is extended from Euclidean space to Riemannian manifold by k-Means clustering, in which Stein divergence is adopted as a similarity metric. The histogram feature is extracted by encoding RCovDs of the cloud image blocks with BoF-based codebook. The multi-class support vector machine (SVM) is utilized for the recognition of cloud types. The experiments on the ground-based cloud image datasets validate the proposed method and exhibit the competitive performance against state-of-the-art methods.
更多查看译文
AI 理解论文
溯源树
样例
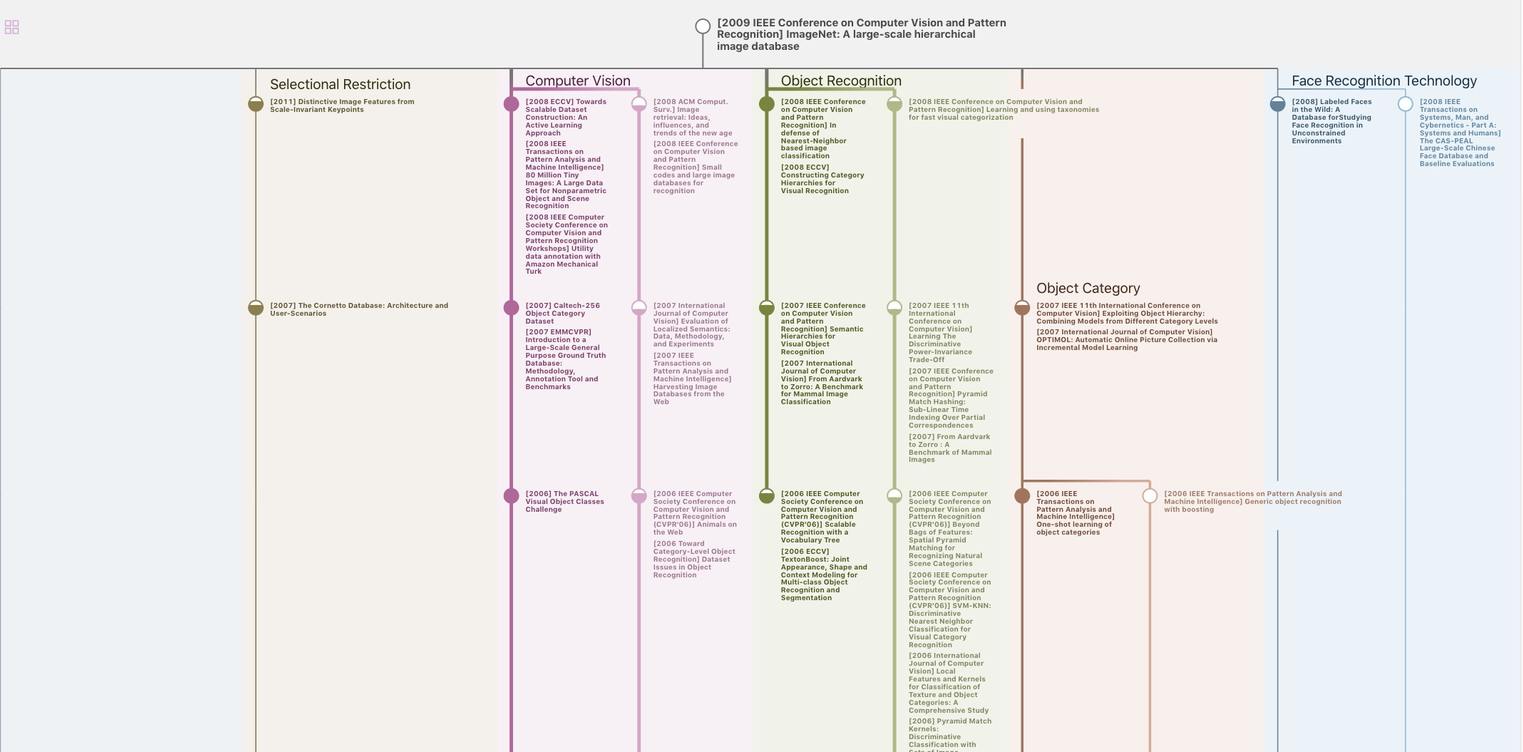
生成溯源树,研究论文发展脉络
Chat Paper
正在生成论文摘要