Road extraction from aerial image data via multiple features integrated with convolution long short time memory unit network
REMOTE SENSING LETTERS(2020)
摘要
Semantic segmentation models based on deep learning have shown remarkable performance in road extraction from high-resolution aerial images. However, it is still a difficult task to segment multiscale roads with high completeness and accuracy from complex backgrounds. To deal with this problem, this letter proposes an end to end network named Multiple features integrated with convolutional long-short time memory unit network (MFI-CLSTMN). First, in MFI-CLSTMN, the ConvLSTM unit is designed to explore and integrate the sequential correlations among features, which can alleviate the feature loss caused by the max-pooling operation. Second, the structure of dense concatenation and multiscale up-sampling combines detailed features with semantic information to preserve road details. At last, at the optimization stage, a self-adaptive composite loss function is added to handle class imbalance, such that MFI-CLSTMN can effectively train hard examples and avoids local optimum. Experiments demonstrate that MFI-CLSTMN has higher segmentation accuracy and lower computational complexity than four comparative state-of-the-art models in a consistent environment. Moreover, MFI-CLSTMN can especially protect road segmentation from netsplit and brokenness, which is hard for other models to achieve.
更多查看译文
AI 理解论文
溯源树
样例
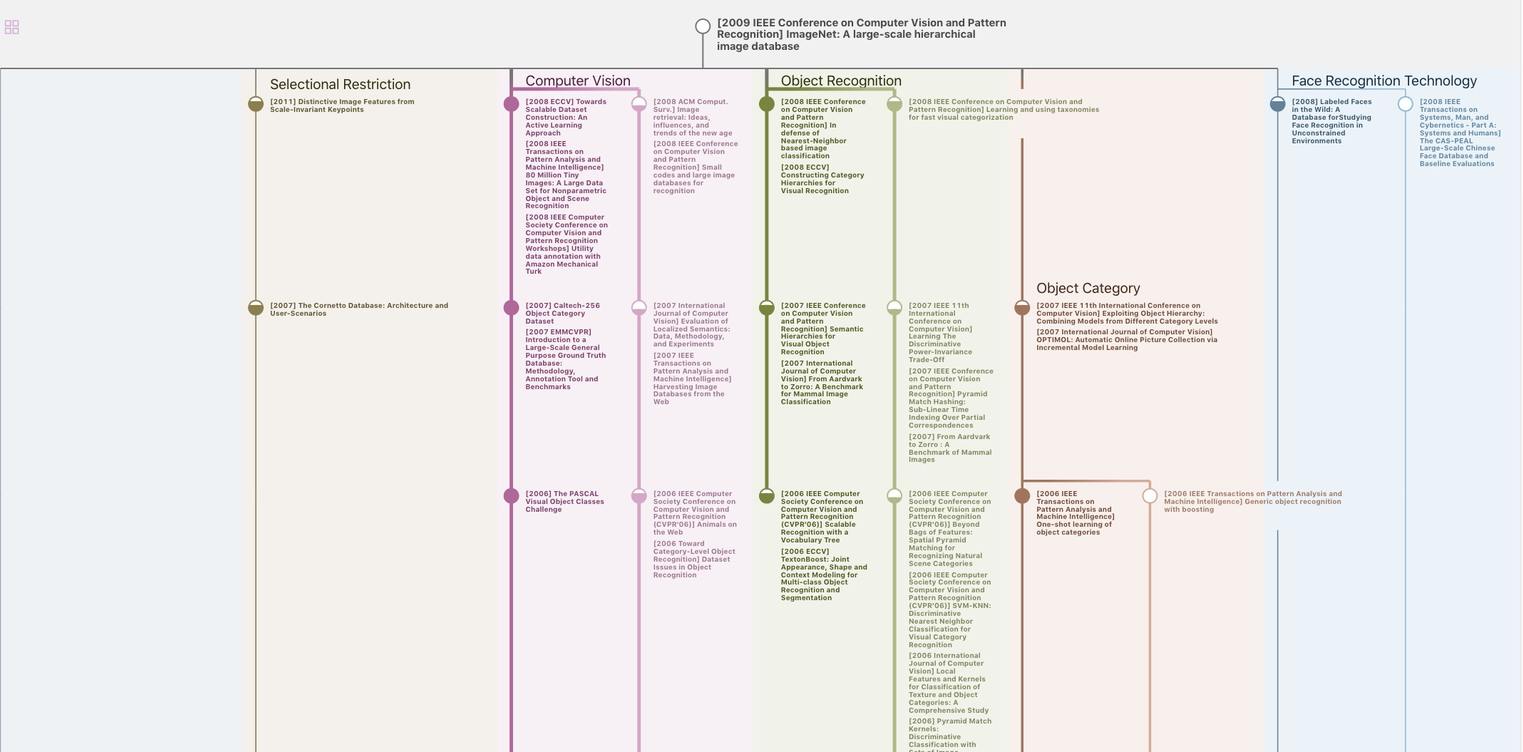
生成溯源树,研究论文发展脉络
Chat Paper
正在生成论文摘要