Superresolution Land Cover Mapping Using a Generative Adversarial Network
IEEE GEOSCIENCE AND REMOTE SENSING LETTERS(2022)
摘要
Superresolution mapping (SRM) is a commonly used method to cope with the problem of mixed pixels when predicting the spatial distribution within low-resolution pixels. Central to the popular SRM method is the spatial pattern model, which is utilized to represent the land cover spatial distribution within mixed pixels. The use of an inappropriate spatial pattern model limits such SRM analyses. Alternative approaches, such as deep-learning-based algorithms, which learn the spatial pattern from training data through a convolutional neural network, have been shown to have considerable potential. Deep learning methods, however, are limited by issues such as the way the fraction images are utilized. Here, a novel SRM model based on a generative adversarial network (GAN), GAN-SRM, is proposed that uses an end-to-end network to address the main limitations of existing SRM methods. The potential of the proposed GAN-SRM model was assessed using four land cover subsets and compared to hard classification and several popular SRM methods. The experimental results show that of the set of methods explored, the GAN-SRM model was able to generate the most accurate high-resolution land cover maps.
更多查看译文
关键词
Generative adversarial networks, Training data, Layout, Spatial resolution, Training, Gallium nitride, Deep learning, generative adversarial network (GAN), super-resolution mapping (SRM)
AI 理解论文
溯源树
样例
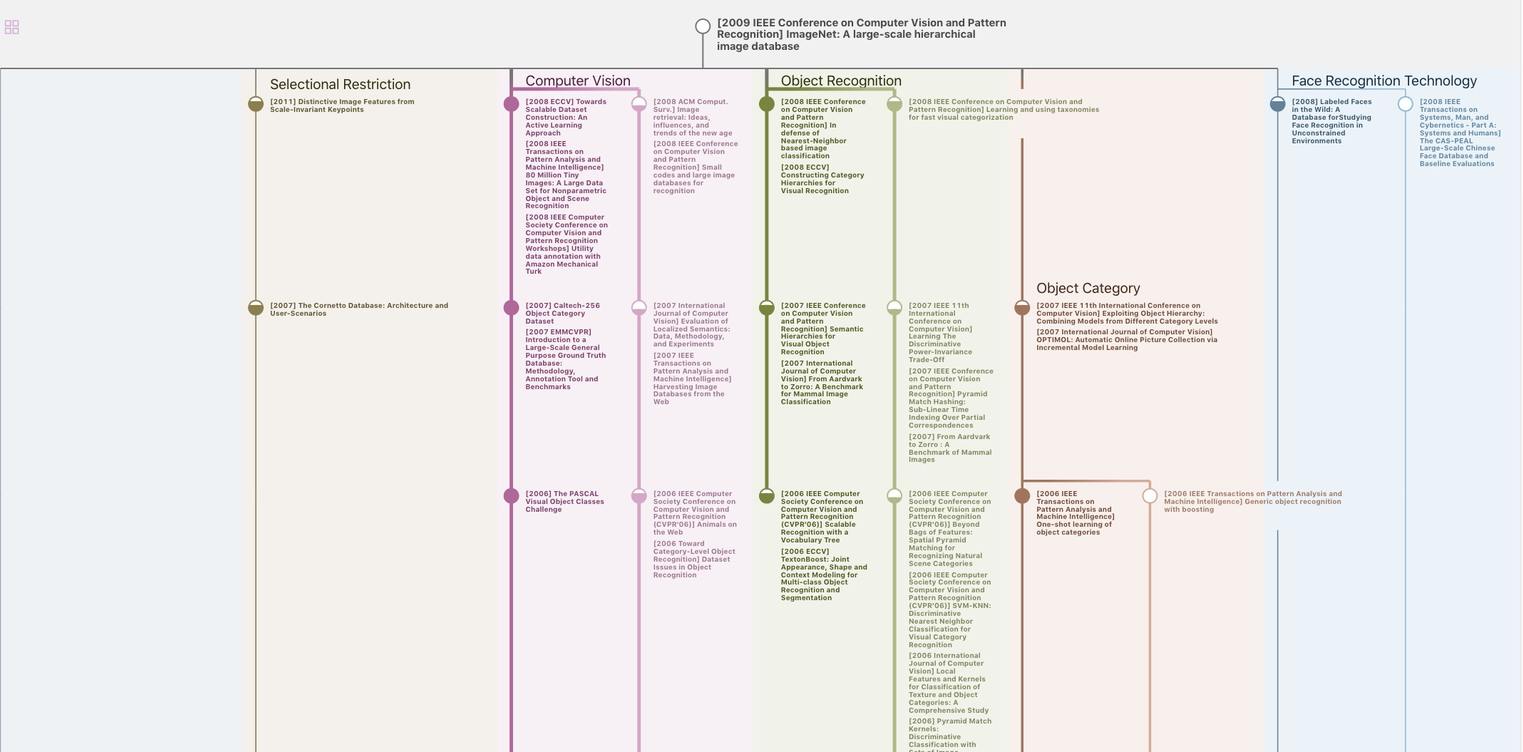
生成溯源树,研究论文发展脉络
Chat Paper
正在生成论文摘要