Enhanced Graph Isomorphism Network For Molecular Admet Properties Prediction
IEEE ACCESS(2020)
摘要
The evaluation of absorption, distribution, metabolism, exclusion, and toxicity (ADMET) properties plays a key role in a variety of domains including industrial chemicals, agrochemicals, cosmetics, environmental science, food chemistry, and particularly drug development. Since molecules are often intrinsically described as molecular graphs, graph neural networks have recently been studied to improve the prediction of ADMET properties. Among many graph neural networks published in recent years, Graph Isomorphism Network (GIN) is a relatively recent and very promising one. In this paper, we propose an enhanced GIN, called MolGIN, via exploiting the bond features and differences influence of the atom neighbors to end-to-end predict ADMET properties. Based on GIN, MolGIN concatenates the bond feature together with node feature in the feature aggregator and applies a gate unit to adjust the atomic neighborhood weights to map the differences in the interaction strength between the central atom and its neighbors, such that more meaningful structural patterns of molecules can be explored toward better molecular modeling. Extensive experiments were conducted on seven public datasets to evaluate MolGIN against four baseline models with benchmark metrics. Experimental results of MolGIN were also compared with state-of-the-art results published in the last three years on each dataset. Experimental results in terms of RMSE and AUC show that MolGIN significantly boosts the prediction performance of GIN and markedly outperforms the baseline models, and achieves comparable or superior performance to state-of-the-art results.
更多查看译文
关键词
Predictive models,Drugs,Neural networks,Compounds,Machine learning,Chemicals,Logic gates,ADMET prediction,graph convolutional network,graph isomorphism network,molecular property,quantitative structure-property relationship
AI 理解论文
溯源树
样例
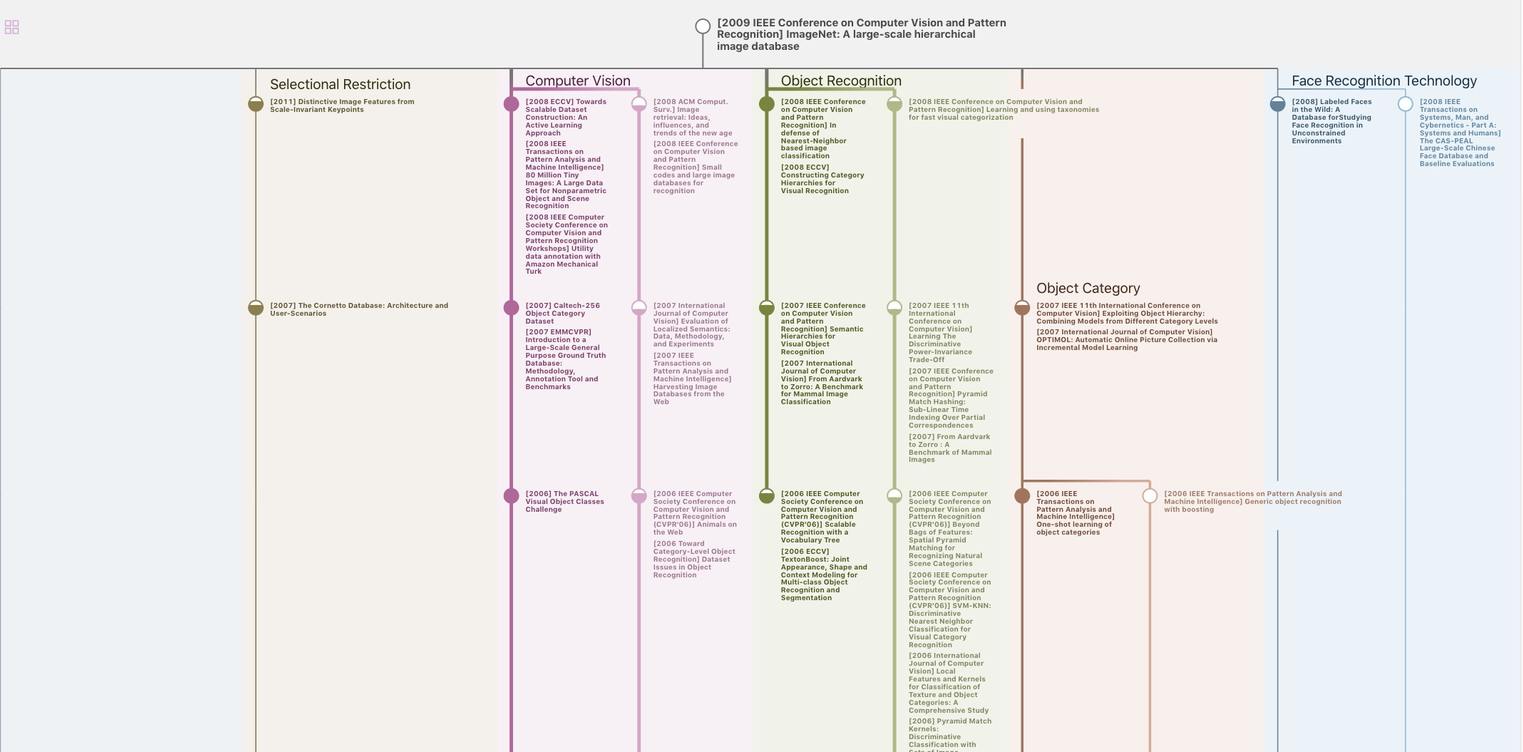
生成溯源树,研究论文发展脉络
Chat Paper
正在生成论文摘要