Statistics manifold learning approach and its application to non-Gaussian process monitoring
chinese control conference(2020)
摘要
Feature extraction plays a key role in the data-driven process monitoring. Recently, manifold learning approaches have shown good effectiveness of preserving manifold structure features of process data. However, conventional algorithms only focus on low-order statistics while ignore high-order statistics, which makes them unable to extract non-Gaussian features of data effectively. Moreover, process data may not strictly follow Gaussian distribution in the complex modern industrial processes. In this paper, to address the above issues, a new manifold learning method named statistics local and nonlocal embedding (SLNLE) is proposed for non-Gaussian process monitoring. Firstly, both low-order and high-order process statistics are conducted by statistics pattern analysis (SPA) algorithm under given time window. Then, local and nonlocal embedding (LNLE) is adopted to preserve manifold structure information of them. Compared with locality preserving projections (LPP) algorithm only retaining local structure features, LNLE implements global and local feature extraction simultaneously, which can preserve non-Gaussian features more effectively. Lastly, a SLNLE-based monitoring model is developed and kernel density estimation (KDE) is applied to obtain more accurate control limits for better process monitoring performance. The feasibility and superiority of the proposed approach are tested on the Tennessee Eastman (TE) process.
更多查看译文
关键词
Non-Gaussian process monitoring,Statistics pattern analysis,Manifold learning,Feature extraction,Fault detection
AI 理解论文
溯源树
样例
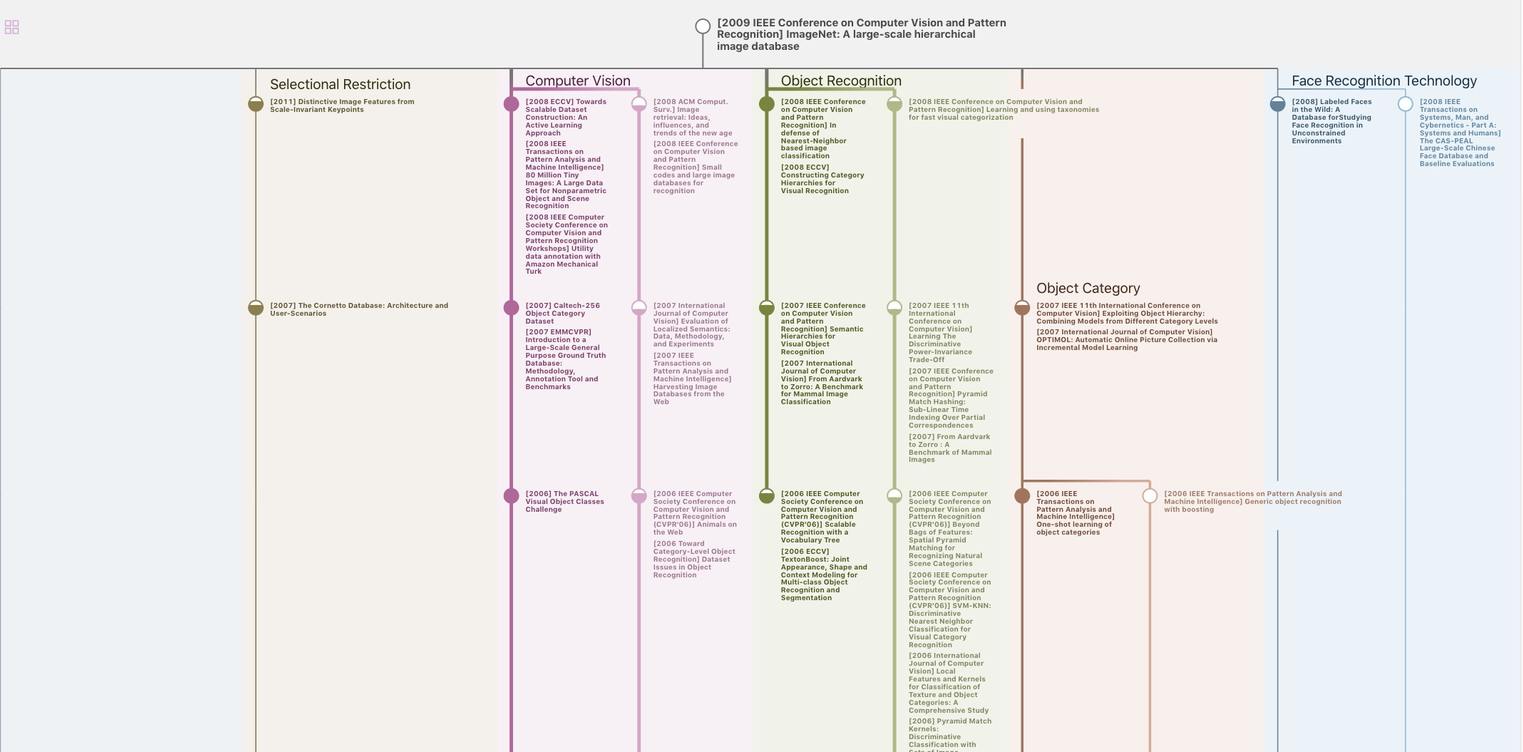
生成溯源树,研究论文发展脉络
Chat Paper
正在生成论文摘要