Efficient Multiple Imputation for Sensitivity Analysis of Recurrent Events Data With Informative Censoring
STATISTICS IN BIOPHARMACEUTICAL RESEARCH(2022)
摘要
Missing data are commonly encountered in clinical trials due to dropout or nonadherence to study procedures. In trials in which recurrent events are of interest, the observed count can be an undercount of the events if a patient drops out before the end of the study. In many applications, the data are not necessarily missing at random (MAR) and it is often not possible to test the MAR assumption. Consequently, it is critical to conduct sensitivity analysis. We develop a control-based multiple imputation method for recurrent events data, where patients who drop out of the study are assumed to have a similar response profile to those in the control group after dropping out. Specifically, we consider the copy reference approach and the jump to reference approach. We model the recurrent event data using a semiparametric proportional intensity frailty model with the baseline hazard function completely unspecified. We develop nonparametric maximum likelihood estimation and inference procedures. We then impute the missing data based on the large sample distribution of the resulting estimators. The variance estimation is corrected by a bootstrap procedure. Simulation studies demonstrate the proposed method performs well in practical settings. We provide applications to two clinical trials.
更多查看译文
关键词
Bootstrap method, Clinical trials, Missing data, Nonparametric maximum likelihood estimation
AI 理解论文
溯源树
样例
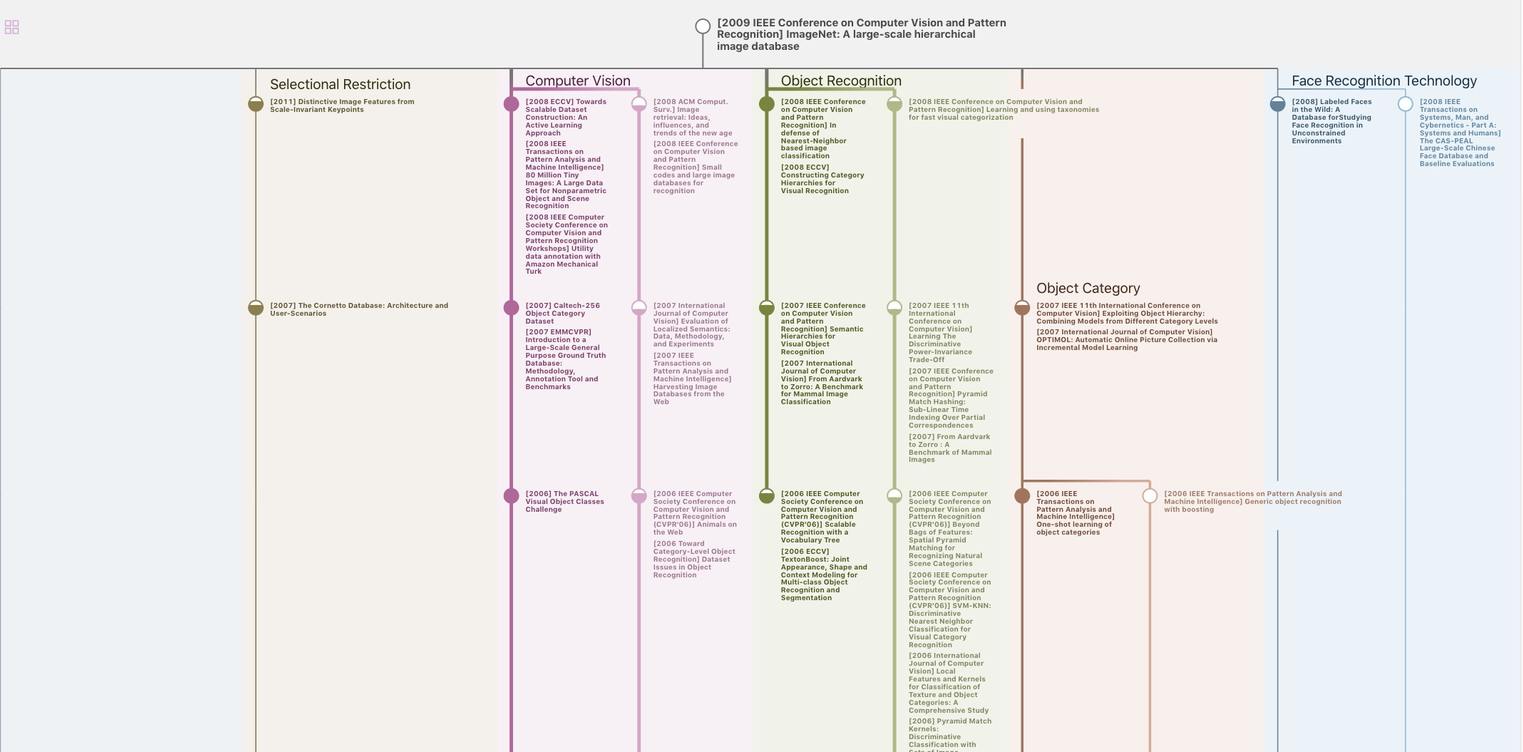
生成溯源树,研究论文发展脉络
Chat Paper
正在生成论文摘要