Survey on RNN and CRF models for de-identification of medical free text
Journal of Big Data(2020)
摘要
The increasing reliance on electronic health record (EHR) in areas such as medical research should be addressed by using ample safeguards for patient privacy. These records often tend to be big data, and given that a significant portion is stored as free (unstructured) text, we decided to examine relevant work on automated free text de-identification with recurrent neural network (RNN) and conditional random field (CRF) approaches. Both methods involve machine learning and are widely used for the removal of protected health information (PHI) from free text. The outcome of our survey work produced several informative findings. Firstly, RNN models, particularly long short-term memory (LSTM) algorithms, generally outperformed CRF models and also other systems, namely rule-based algorithms. Secondly, hybrid or ensemble systems containing joint LSTM-CRF models showed no advantage over individual LSTM and CRF models. Thirdly, overfitting may be an issue when customized de-identification datasets are used during model training. Finally, statistical validation of performance scores and diversity during experimentation were largely ignored. In our comprehensive survey, we also identify major research gaps that should be considered for future work.
更多查看译文
关键词
De-identification, Big Data, Recurrent neural network, Conditional random field, Machine learning
AI 理解论文
溯源树
样例
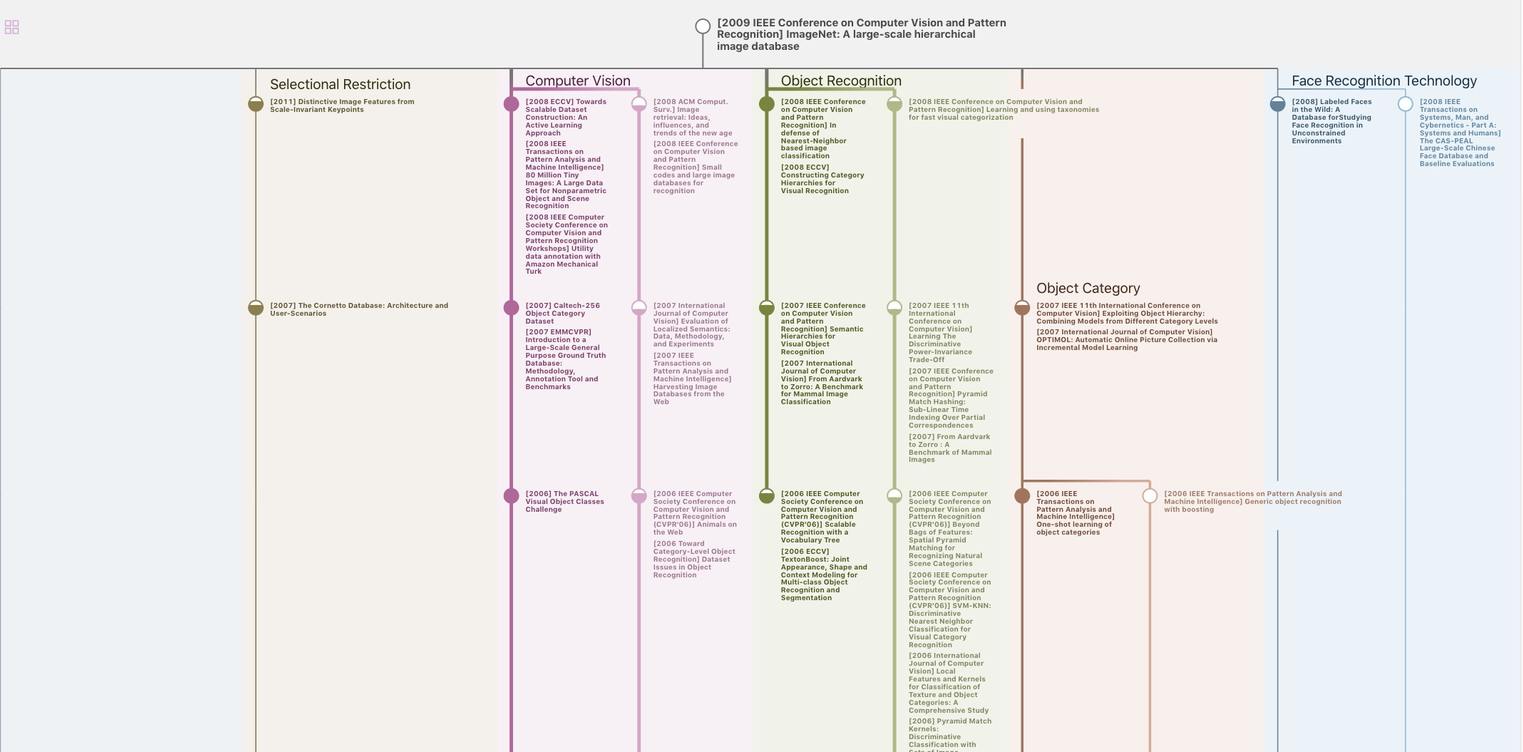
生成溯源树,研究论文发展脉络
Chat Paper
正在生成论文摘要