Indirect tool monitoring in drilling based on gap sensor signal and multilayer perceptron feed forward neural network
JOURNAL OF INTELLIGENT MANUFACTURING(2020)
摘要
In this study, an indirect tool monitoring was developed based on the installation of a gap sensor in measuring the signal related to the tool behavior during the drilling process. Eleven types of twist drills with different tool conditions were utilized to differentiate the sensorial signals based on the tool states. A statistical analysis was conducted in the signal processing, by extracting the gap sensor signal associates from each tool condition, using the skewness and kurtosis features. Multi-class classification was conducted using the multilayer perceptron (MLP) feed forward neural network (FF-NN) model to classify and predict the tool condition based on the skewness and kurtosis data. The architectures of the MLP FF-NN models were varied to optimize the classification accuracy. This study found that the tool condition was correlated to the displacement of the drill machine spindle because the runout occurred when the sensor signal displayed fluctuation and irregularity trends. The peak intensity of the gap sensor signals increased with increasing wear severity of the twist drill. An ideal MLP FF-NN structure was achieved when the classification performance was optimized to be consistent with the learning curve.
更多查看译文
关键词
Indirect tool monitoring, Drilling, Supervised learning, Multilayer perceptron feed forward neural network, Statistical analysis
AI 理解论文
溯源树
样例
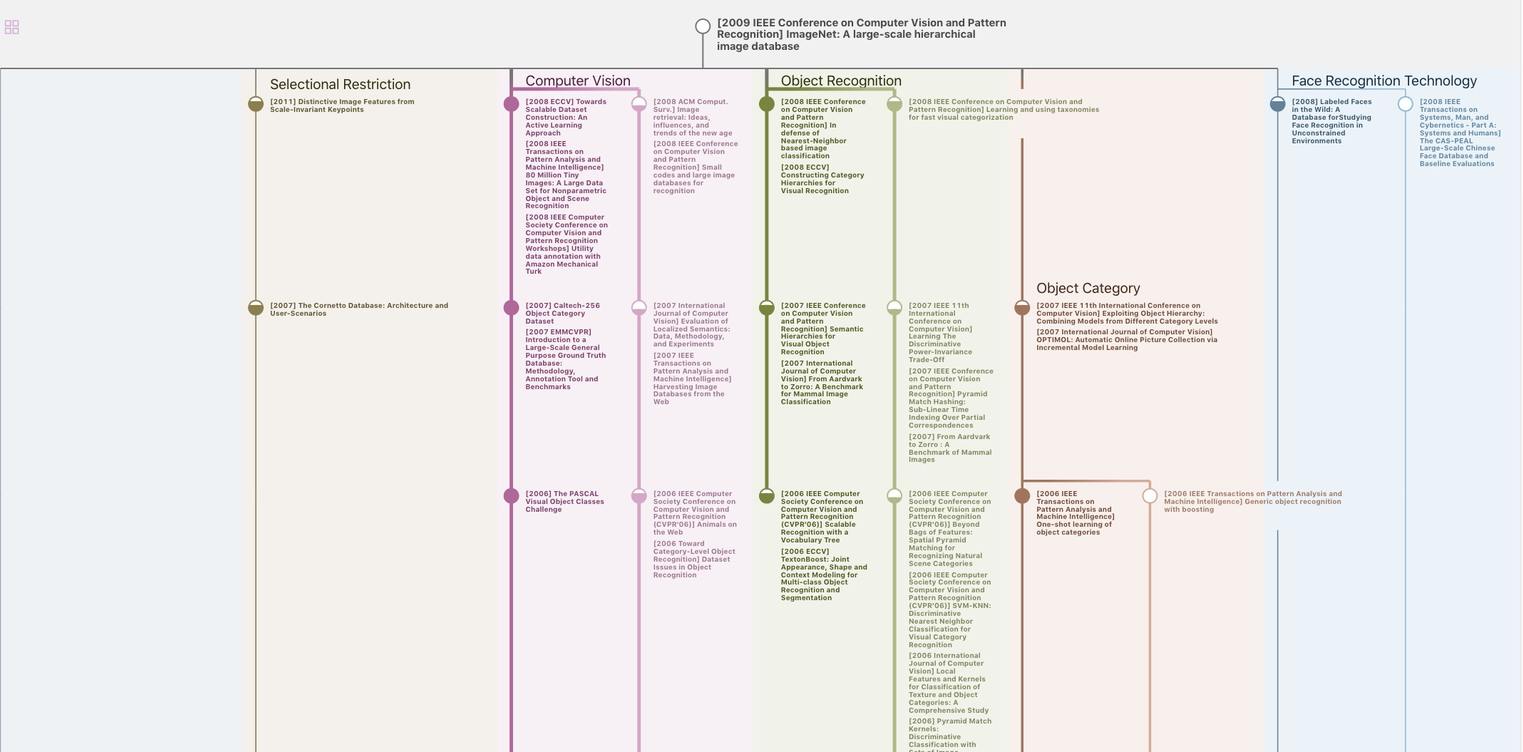
生成溯源树,研究论文发展脉络
Chat Paper
正在生成论文摘要