Identification of High Leverage Points in Linear Functional Relationship Model
PAKISTAN JOURNAL OF STATISTICS AND OPERATION RESEARCH(2020)
Abstract
In a standard linear regression model the explanatory variables, X, are considered to be fixed and hence assumed to be free from errors. But in reality, they are variables and consequently can be subjected to errors. In the regression literature there is a clear distinction between outlier in the Y- space or errors and the outlier in the X-space. The later one is popularly known as high leverage points. If the explanatory variables are subjected to gross error or any unusual pattern we call these observations as outliers in the X- space or high leverage points. High leverage points often exert too much influence and consequently become responsible for misleading conclusion about the fitting of a regression model, causing multicollinearity problems, masking and/or swamping of outliers etc. Although a good number of works has been done on the identification of high leverage points in linear regression model, this is still a new and unsolved problem in linear functional relationship model. In this paper, we suggest a procedure for the identification of high leverage points based on deletion of a group of observations. The usefulness of the proposed method for the detection of multiple high leverage points is studied by a well-known data set and Monte Carlo simulations.
MoreTranslated text
Key words
Errors in variable,Leverages,Masking,Swamping,Monte Carlo simulation
AI Read Science
Must-Reading Tree
Example
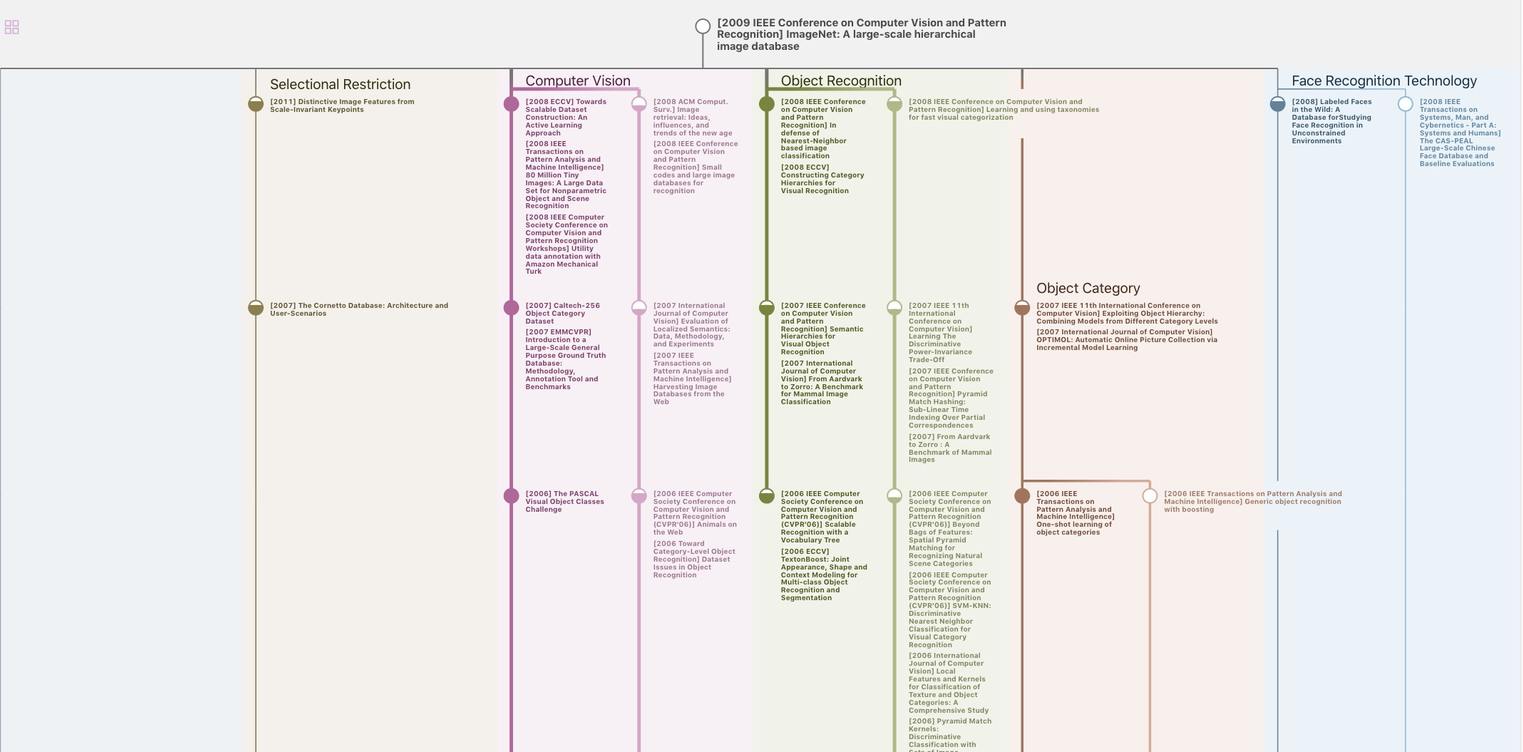
Generate MRT to find the research sequence of this paper
Chat Paper
Summary is being generated by the instructions you defined