Gesture Recognition from Bio-signals Using Hybrid Deep Neural Networks
international conference on artificial intelligence(2020)
摘要
Surface electromyogram (sEMG) provides a promising means to develop a non-invasive prosthesis control system. In the context of transradial amputees, it allows a limited but functionally useful return of hand function that can significantly improve patients\u0027 quality of life. In order to predict users\u0027 motion intention, the ability to process multichannel sEMG signals generated by muscle is required. We propose an attention-based Bidirectional Convolutional Gated Recurrent Unit (Bi-CGRU) deep neural network to analyse sEMG signals. The two key novel aspects of our work include: firstly, novel use of a bi-directional sequential GRU to focus on the inter-channel relationship between both the prior time steps and the posterior signals. This enhances the intra-channel features extracted by an initial one-dimensional CNN. Secondly, an attention component is employed at each GRU layer. This mechanism learns different intra-attention weights, enabling focus on vital parts and corresponding dependencies of the signal. This increases robustness to feature noise to further improve accuracy. The attention-based Bi-CGRU is evaluated on the Ninapro benchmark dataset of sEMG hand gestures. The electromyogram signals of 17 hand gestures from 10 subjects from the database are tested. The average accuracy achieved 88.73%, outperforming the state-of-the-art approaches on the same database. This demonstrates that the proposed attention based Bi-CGRU model provides a promising bio-control solution for robotic prostheses.
更多查看译文
关键词
surface electromyogram,myoelectric control,gesture and movement recognition,deep learning,attention mechanism,gated recurrent unit,signal processing
AI 理解论文
溯源树
样例
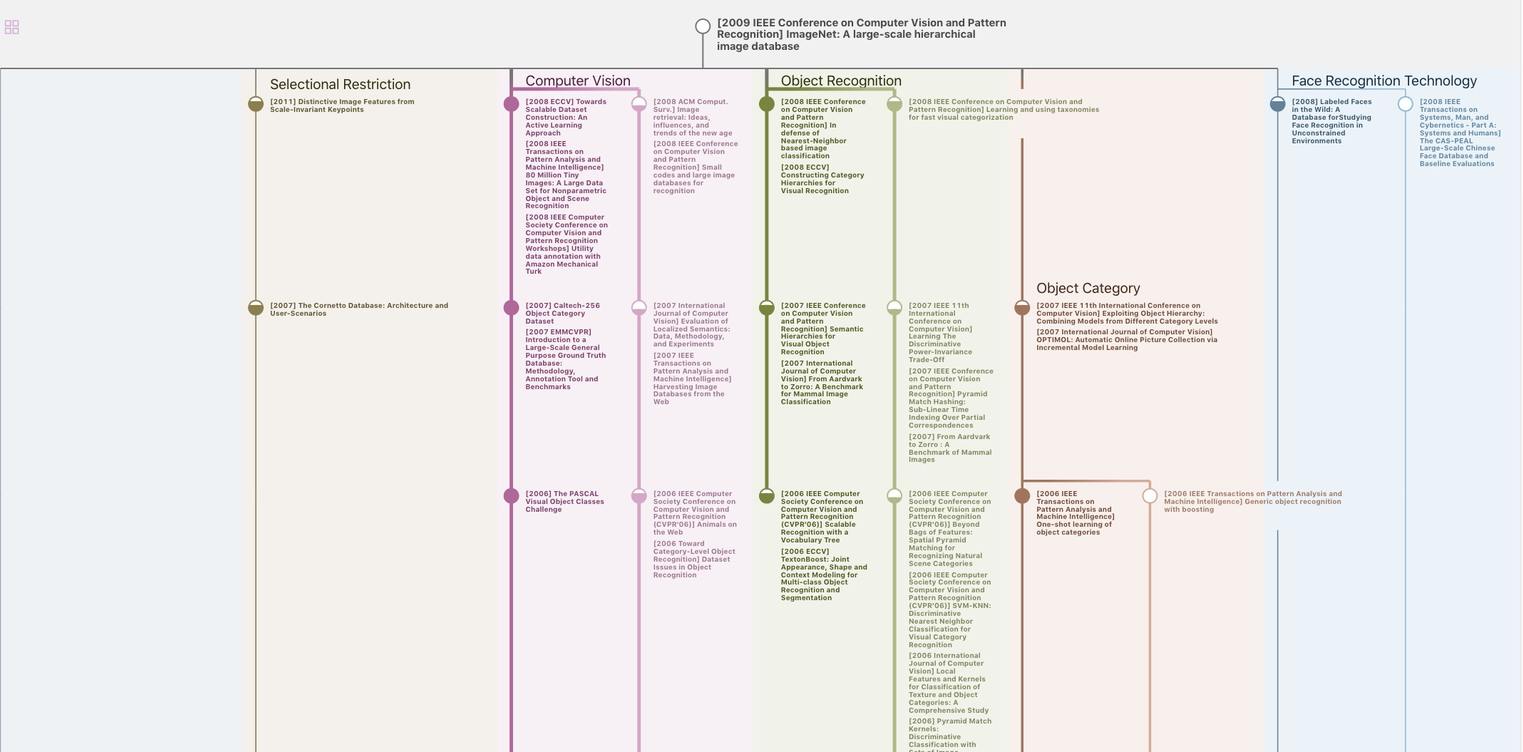
生成溯源树,研究论文发展脉络
Chat Paper
正在生成论文摘要