Delta Prognosis: A Novel Clinical Outcome Based On Automated Analysis Of Unstructured Cancer Ehr Data
CANCER RESEARCH(2020)
Abstract
Introduction: Efforts such as AACR Project GENIE pool genomic data across institutions to facilitate understanding of the clinical impact of genomic biomarkers in cancer. However, correlating genomic data with treatment outcomes is challenging, since outside clinical trials, outcomes such as improving or worsening cancer may be recorded only in electronic health record (EHR) free text. Automated methods could accelerate discovery by reliably characterizing treatment outcomes from unstructured EHR data. Methods: We analyzed unstructured imaging reports for patients with solid tumors (any stage) who participated in an institutional next generation sequencing study from 2013-2018 and received palliative-intent systemic therapy. A recurrent neural network was trained to predict overall survival (OS) following each report, using text from the report and the sequence of prior reports for the patient. Model performance was measured using the concordance (c)-index in a 10% held-out validation set. Next, the ‘delta prognosis, (ΔP)9 was measured for each report. ‘Delta prognosis9 (ΔP) captures the change in the number of months a patient is predicted to be alive out of the 12 months following each report, compared to the exponentially weighted moving average of prior prognostic predictions for that patient. Finally, a second neural network was trained to predict ΔP using each report. Ten-fold cross validation was used to annotate each report so ΔP measures for each patient would not be based on that patient9s actual survival time. Using joint modeling of ΔP, biomarker status, and report frequency, associations were measured between ΔP and previously described prognostic biomarkers for cancer types in which prolonged survival with advanced disease is common, including BRAF mutations in colorectal cancer and BRCA1/2 mutations in ovarian cancer. Results: Model training was performed using 78,371 imaging reports for 4,512 patients with 273 cancer histologies. In a validation set of 9,306 reports for 595 patients, the c-index for survival prediction was 0.76. Within the first 6 months of palliative-intent therapy, among 597 patients with colorectal cancer, BRAF mutations were associated with worse mean ΔP (-0.33 months per report; 95% CI, -0.55 to -0.11 months; p = 0.003); among 395 patients with ovarian cancer, BRCA1/2 mutations were associated with better mean ΔP (+0.39 months per report; 95% CI, 0.17-0.61 months; p Conclusion: Neural networks trained to identify shifts in prognosis using EHR text may enable ascertainment of improving and worsening cancer with no manual labeling, even if overall survival data are unavailable or immature at inference time. This technique could be relevant to any analysis of cancer outcomes using EHR data. Citation Format: Kenneth L. Kehl, Haitham A. Elmarakeby, Michael J. Hassett, Eliezer M. Van Allen, Deb Schrag. Delta prognosis: A novel clinical outcome based on automated analysis of unstructured cancer EHR data [abstract]. In: Proceedings of the Annual Meeting of the American Association for Cancer Research 2020; 2020 Apr 27-28 and Jun 22-24. Philadelphia (PA): AACR; Cancer Res 2020;80(16 Suppl):Abstract nr 2062.
MoreTranslated text
Key words
unstructured cancer ehr data,delta prognosis,novel clinical outcome
AI Read Science
Must-Reading Tree
Example
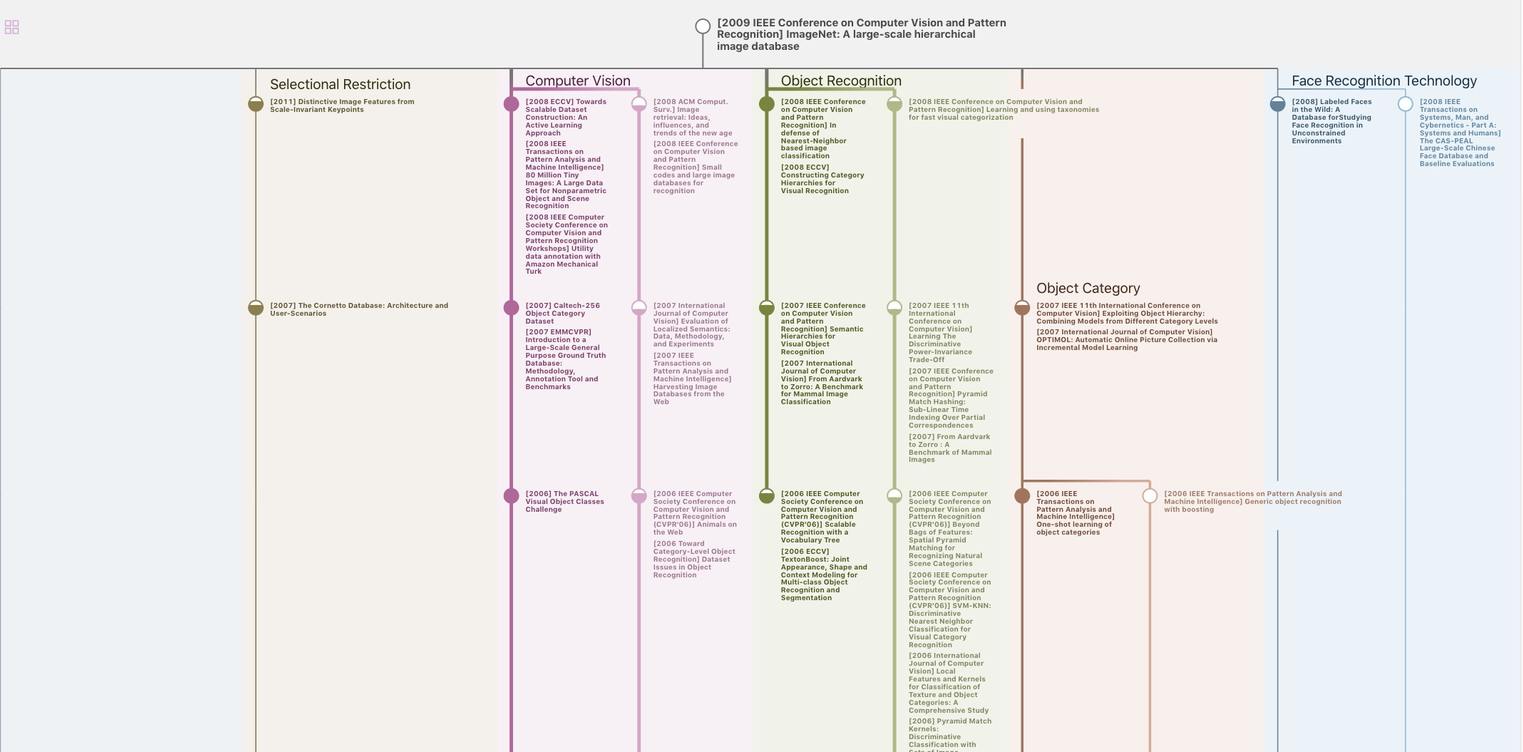
Generate MRT to find the research sequence of this paper
Chat Paper
Summary is being generated by the instructions you defined