Moderately Clipped Lasso For The High-Dimensional Generalized Linear Model
COMMUNICATIONS FOR STATISTICAL APPLICATIONS AND METHODS(2020)
摘要
The least absolute shrinkage and selection operator (LASSO) is a popular method for a high-dimensional regression model. LASSO has high prediction accuracy; however, it also selects many irrelevant variables. In this paper, we consider the moderately clipped LASSO (MCL) for the high-dimensional generalized linear model which is a hybrid method of the LASSO and minimax concave penalty (MCP). The MCL preserves advantages of the LASSO and MCP since it shows high prediction accuracy and successfully selects relevant variables. We prove that the MCL achieves the oracle property under some regularity conditions, even when the number of parameters is larger than the sample size. An efficient algorithm is also provided. Various numerical studies confirm that the MCL can be a better alternative to other competitors.
更多查看译文
关键词
generalized linear model, moderately clipped LASSO, oracle property, variable selection
AI 理解论文
溯源树
样例
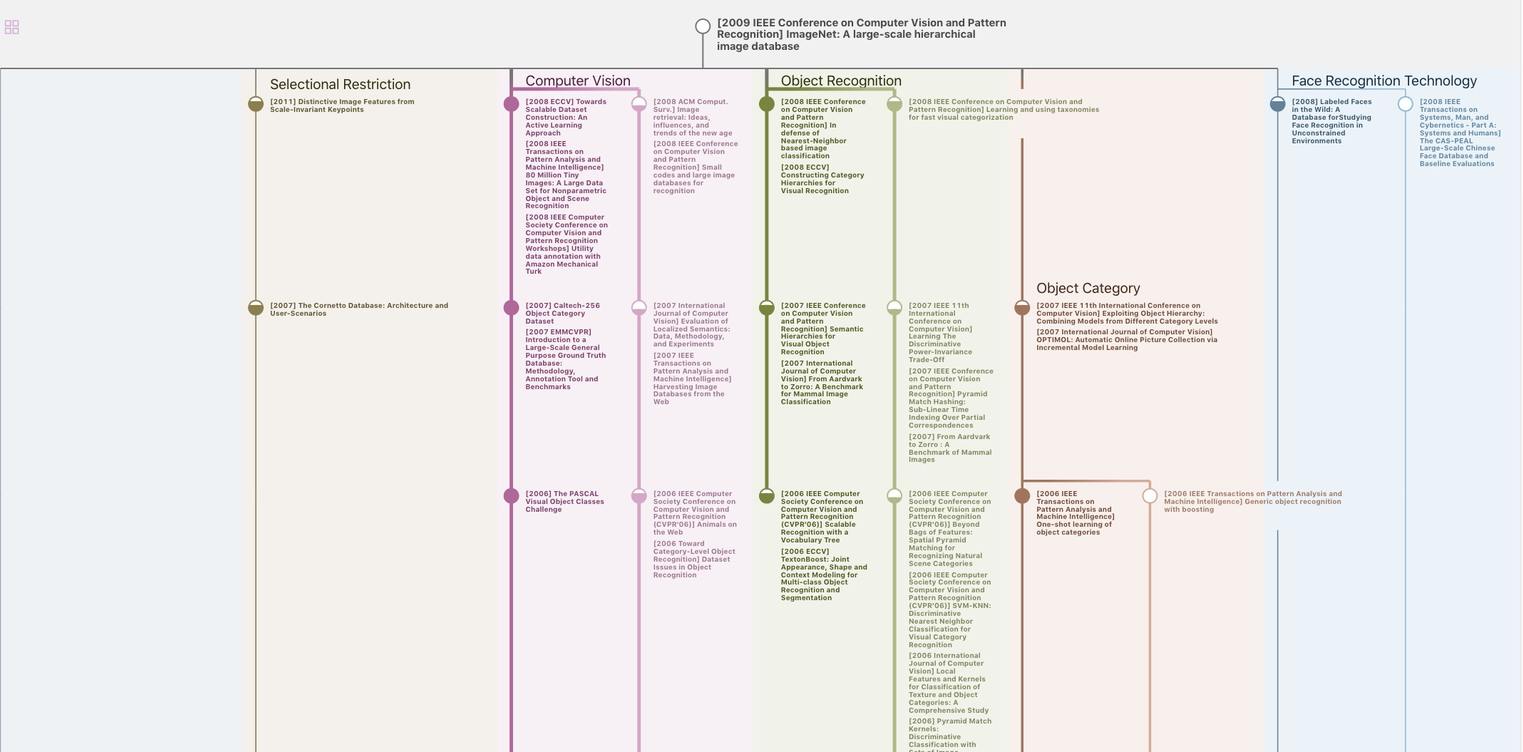
生成溯源树,研究论文发展脉络
Chat Paper
正在生成论文摘要