Photovoltaic power forecasting based on a support vector machine with improved ant colony optimization
JOURNAL OF CLEANER PRODUCTION(2020)
Abstract
Accurate prediction of photovoltaic (PV) power for an ultra-short term can improve the usage of grid-connected PV power. In this study, data preprocessing based on an ultra-short-term PV model is explored. A support vector machine (SVM) is constructed based on the processed data, and the parameters of the SVM are optimized using ant colony optimization (ACO). A series of improvements are introduced to optimize the ACO. The results indicate that the regression coefficient (R-2) of the model can be increased by 6.8% through reasonable data preprocessing. However, smoothing is not suitable for the preprocessing of PV models with large datasets. The R-2 of the hybrid model reaches up to 0.997. In particular, the forecasting accuracies for peak power and nighttime are significantly improved, thereby improving the model's full-time grid-connected generation abilities. (C) 2020 Elsevier Ltd. All rights reserved.
MoreTranslated text
Key words
Photovoltaic power prediction,Data processing,Support vector machine,Parameter optimization,Improved ant colony optimization
AI Read Science
Must-Reading Tree
Example
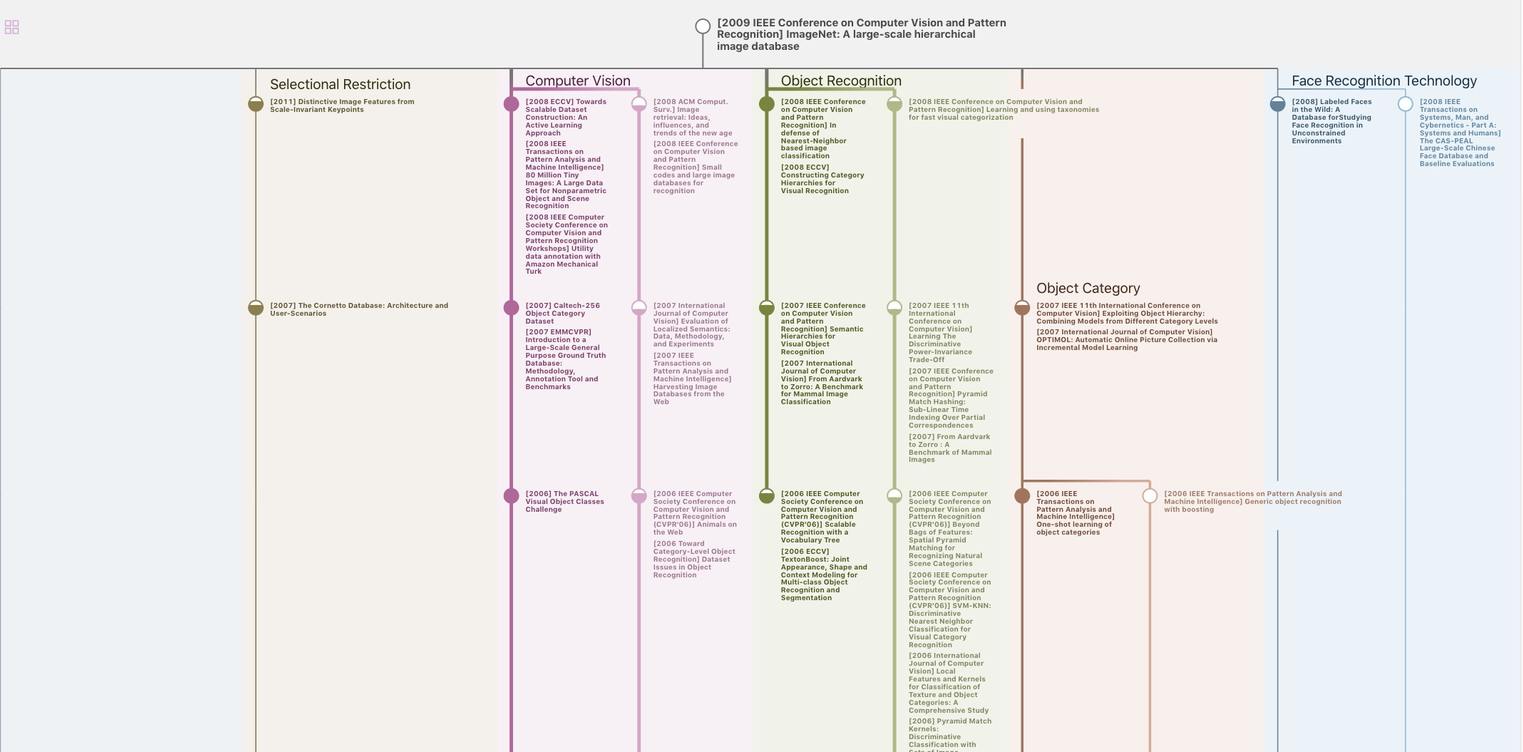
Generate MRT to find the research sequence of this paper
Chat Paper
Summary is being generated by the instructions you defined