Multiplication Method For Fine-Tuning Regularization Parameter Of A Sparse Modeling Technique Tentatively Optimized Via Cross Validation
JOURNAL OF THE PHYSICAL SOCIETY OF JAPAN(2020)
Abstract
Sparse modeling is generally applied to data analysis in the field of experimental physics, using statistical regularization methods such as the least absolute shrinkage and selection operator (LASSO). Therefore, it is essential to adjust the regularization parameter to minimize the estimation error. We focused on the cross validation error and analyzed the relation between the cross validation and estimation errors, by the replica method. From the results of our analysis, we propose a method to fine-tune the regularization parameter of LASSO tentatively optimized via cross validation. On the basis of a numerical experiment, we argue that the proposed method outperforms a heuristic method known as the one-standard-error rule and discuss the characteristics of the proposed method.
MoreTranslated text
Key words
sparse modeling technique tentatively,regularization,fine-tuning
AI Read Science
Must-Reading Tree
Example
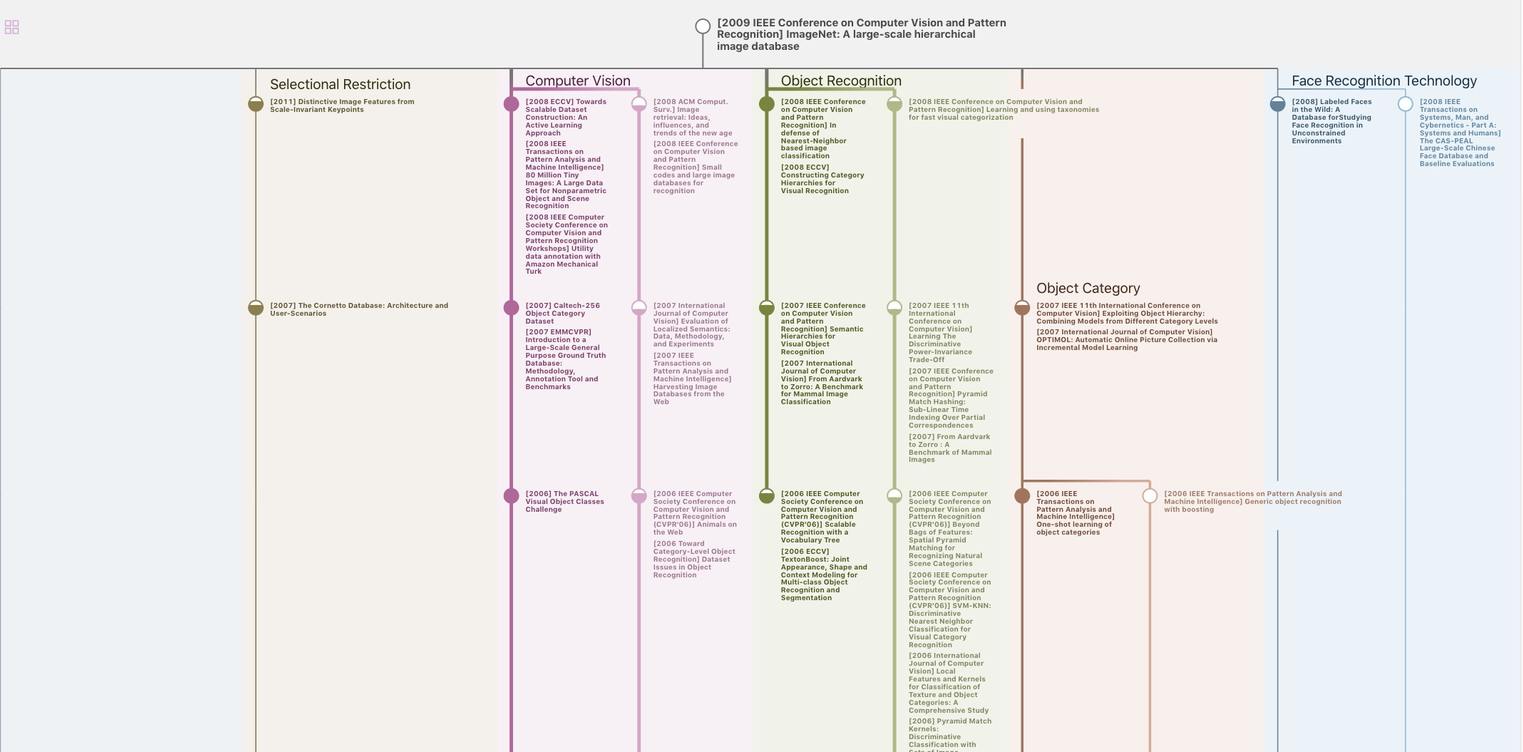
Generate MRT to find the research sequence of this paper
Chat Paper
Summary is being generated by the instructions you defined