Emotion Recognition Using Three-Dimensional Feature And Convolutional Neural Network From Multichannel Eeg Signals
IEEE SENSORS JOURNAL(2021)
摘要
Using electroencephalogram (EEG) signal to recognize emotional states has become a research hotspot of affective computing. Previous emotion recognition methods almost ignored the correlation and interaction among multichannel EEG signals, which may provide salient information related to emotional states. This article proposes a novel approach based on rearranged EEG features and deep learning algorithm. In particular, each channel EEG signal is first processed in time domain to get time-domain features. Then, features of all channels are treated as a three-dimensional (3D) feature matrix, according to positions of electrode sensors. This makes the features closer to the real response of the cerebral cortex. Subsequently, an advanced convolutional neural network (CNN) designed with univariate convolution layer and multivariate convolution layer is employed to deal with the 3D feature matrix for emotion recognition. A benchmark dataset for emotion analysis using physiological signal is employed to evaluate this method. The experimental results proved that the 3D feature matrix can effectively represent the emotion-related features in multichannel EEG signals and the proposed CNN can efficaciously mine the unique features of each channel and the correlation among channels for emotion recognition.
更多查看译文
关键词
Electroencephalography, Feature extraction, Emotion recognition, Time-domain analysis, Three-dimensional displays, Electrodes, Machine learning, Emotion recognition, multichannel EEG signal, three-dimensional feature, CNN, deep learning
AI 理解论文
溯源树
样例
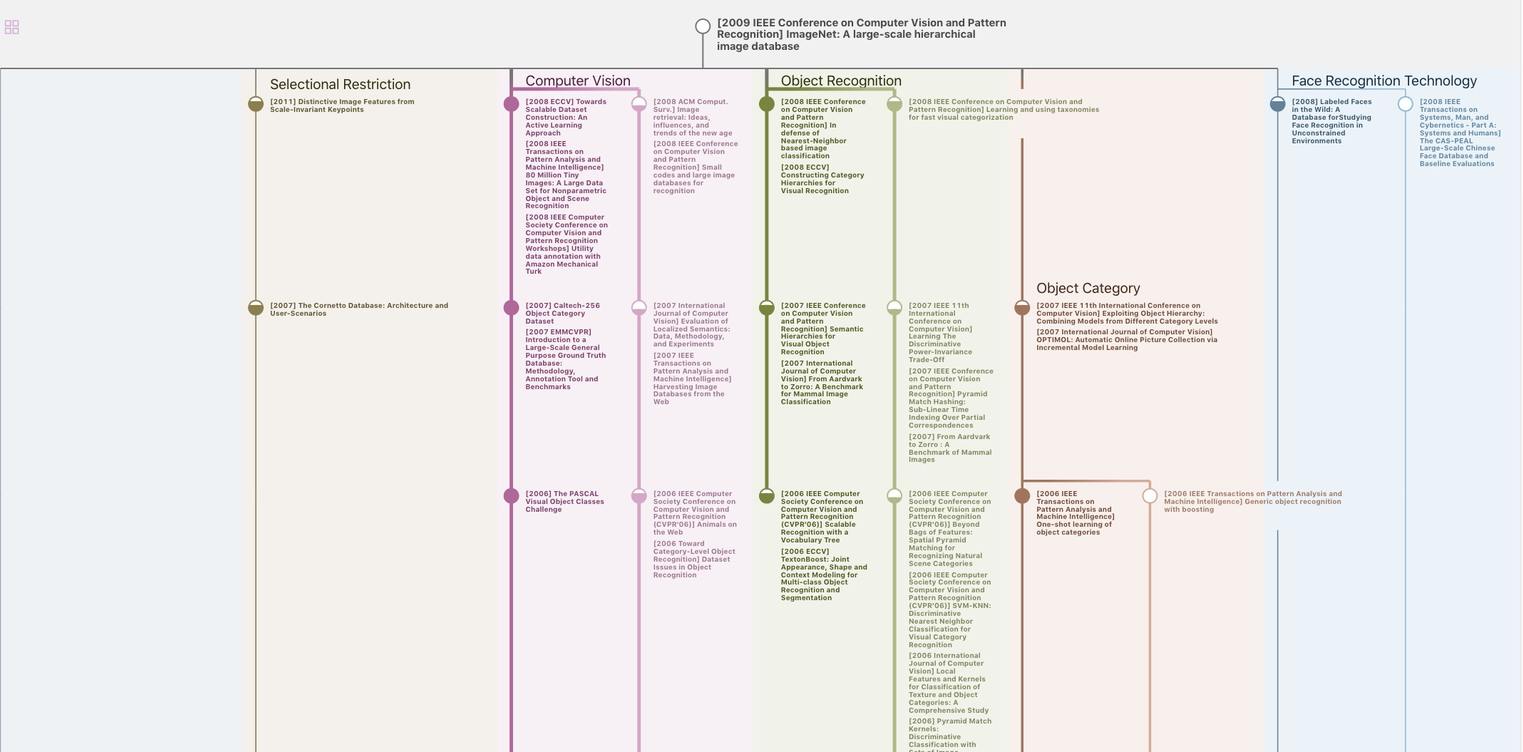
生成溯源树,研究论文发展脉络
Chat Paper
正在生成论文摘要