Using schema.org annotations for training and maintaining product matchers
WIMS(2020)
摘要
Product matching is a central task within e-commerce applications such as price comparison portals and online market places. State-of-the-art product matching methods achieve F1 scores above 0.90 using deep learning techniques combined with huge amounts of training data (e.g \u003e 100K pairs of offers). Gathering and maintaining such large training corpora is costly, as it implies labeling pairs of offers as matches or non-matches. Acquiring the ability to be good at product matching thus means a major investment for an e-commerce company. This paper shows that the manual labeling of training data for product matching can be replaced by relying exclusively on schema.org annotations gathered from the public Web. We show that using only schema.org data for training, we are able to achieve F1 scores between 0.92 and 0.95 depending on the product category. As new products appear everyday, it is important that matching models can be maintained with justifiable effort. In order to give practical advice on how to maintain matching models, we compare the performance of deep learning and traditional matching models on unseen products and experiment with different fine-tuning and re-training strategies for model maintenance, again using only schema.org annotations as training data. Finally, as using the public Web as distant supervision carries inherent noise, we evaluate deep learning and traditional matching models with regards to their label-noise resistance and show that deep learning is able to deal with the amounts of identifier-noise found in schema.org annotations.
更多查看译文
关键词
annotations
AI 理解论文
溯源树
样例
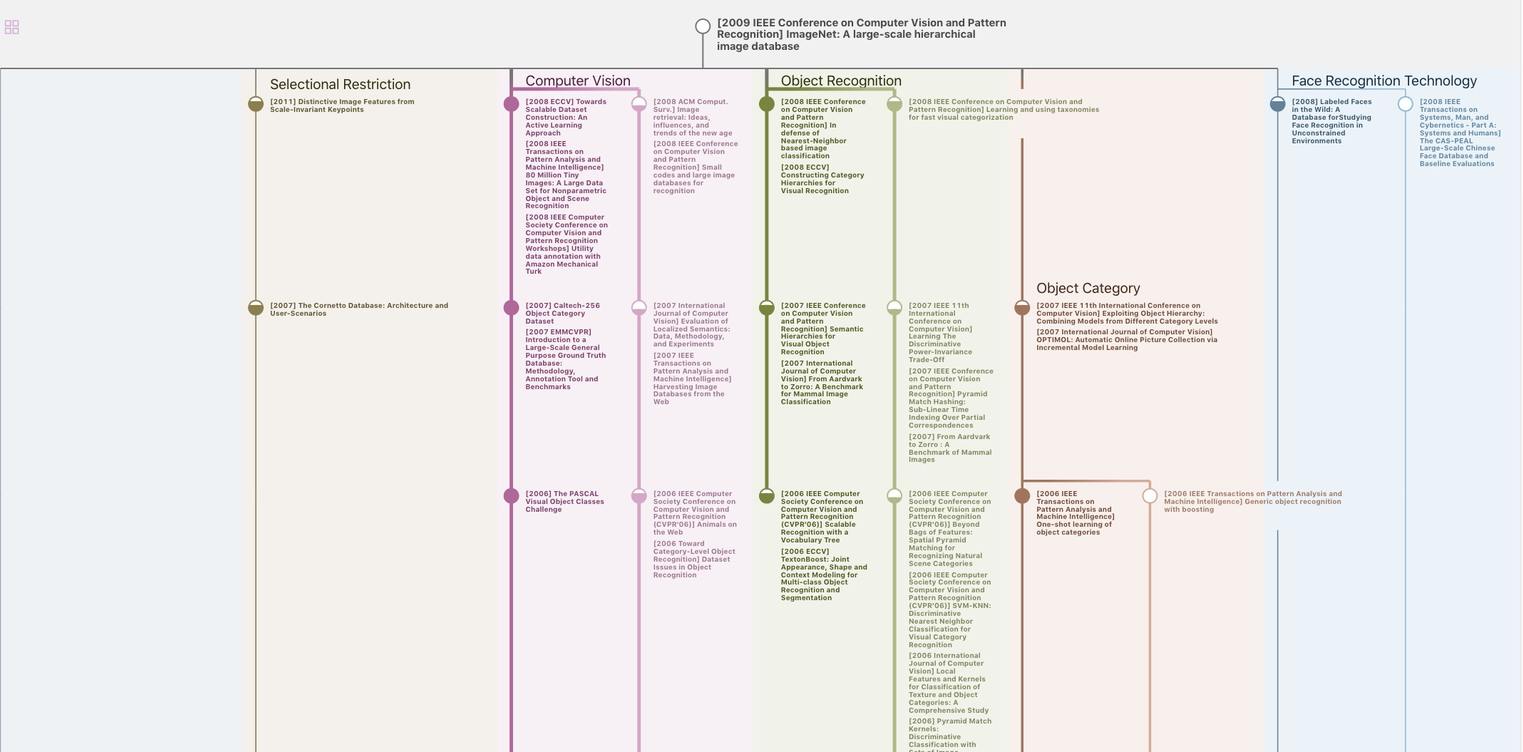
生成溯源树,研究论文发展脉络
Chat Paper
正在生成论文摘要