Deep Learning Approach with Rotate-Shift Invariant Input to Predict Protein Homodimer Structure
ISBRA(2020)
Abstract
The ability to predict protein complexes is important for applications in drug design and generating models of high accuracy in the cell. Recently deep learning techniques showed a significant success in protein structure prediction, but a protein docking problem is unsolved yet. We developed a two-staged approach which consists of deep convolutional neural network to predict protein contact map for homodimers and optimization procedure based on gradient descent to build the homodimer structure from the contact map. Neural network uses the distance map calculated as all pairwise Euclidian distances between CB atoms of protein 3D structure as input, which is invariant to rotation and translation. The network has a large receptive filed to capture patterns in contacts between residues. The suggested approach could be generalized to heterodimers because it does not depend on symmetry features inherent in homodimers. The presented algorithm could be also used for scoring protein homodimers models in docking.
MoreTranslated text
Key words
Protein docking, Protein homodimers, Deep learning
AI Read Science
Must-Reading Tree
Example
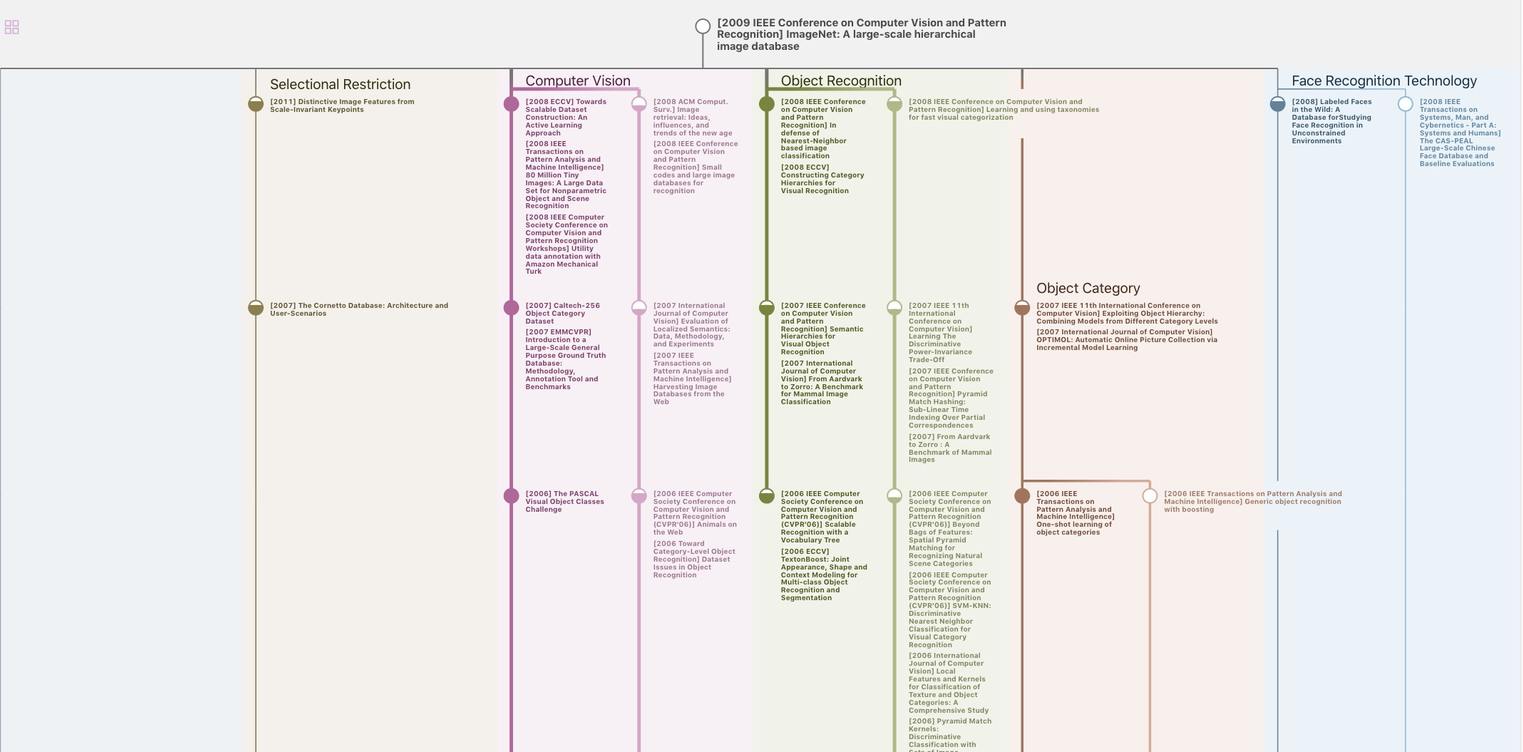
Generate MRT to find the research sequence of this paper
Chat Paper
Summary is being generated by the instructions you defined