Detecting Statistically Significant Events in Large Heterogeneous Attribute Graphs via Densest Subgraphs.
KSEM (1)(2020)
摘要
With the widespread of social platforms, event detection is becoming an important problem in social media. Yet, the large amount of content accumulated on social platforms brings great challenges. Moreover, the content usually is informal, lacks of semantics and rapidly spreads in dynamic networks, which makes the situation even worse. Existing approaches, including content-based detection and network structure-based detection, only use limited and single information of social platforms that limits the accuracy and integrity of event detection. In this paper, (1) we propose to model the entire social platform as a heterogeneous attribute graph (HAG), including types, entities, relations and their attributes; (2) we exploit non-parametric scan statistics to measure the statistical significance of subgraphs in HAG by considering historical information; (3) we transform the event detection in HAG into a densest subgraph discovery problem in statistical weighted network. Due to its NP-hardness, we propose an efficient approximate method to find the densest subgraphs based on (k, \\(\\varPsi \\))-core, and simultaneously the statistical significance is guaranteed. In experiments, we conduct comprehensive empirical evaluations on Weibo data to demonstrate the effectiveness and efficiency of our proposed approaches.
更多查看译文
关键词
large heterogeneous attribute graphs,significant events
AI 理解论文
溯源树
样例
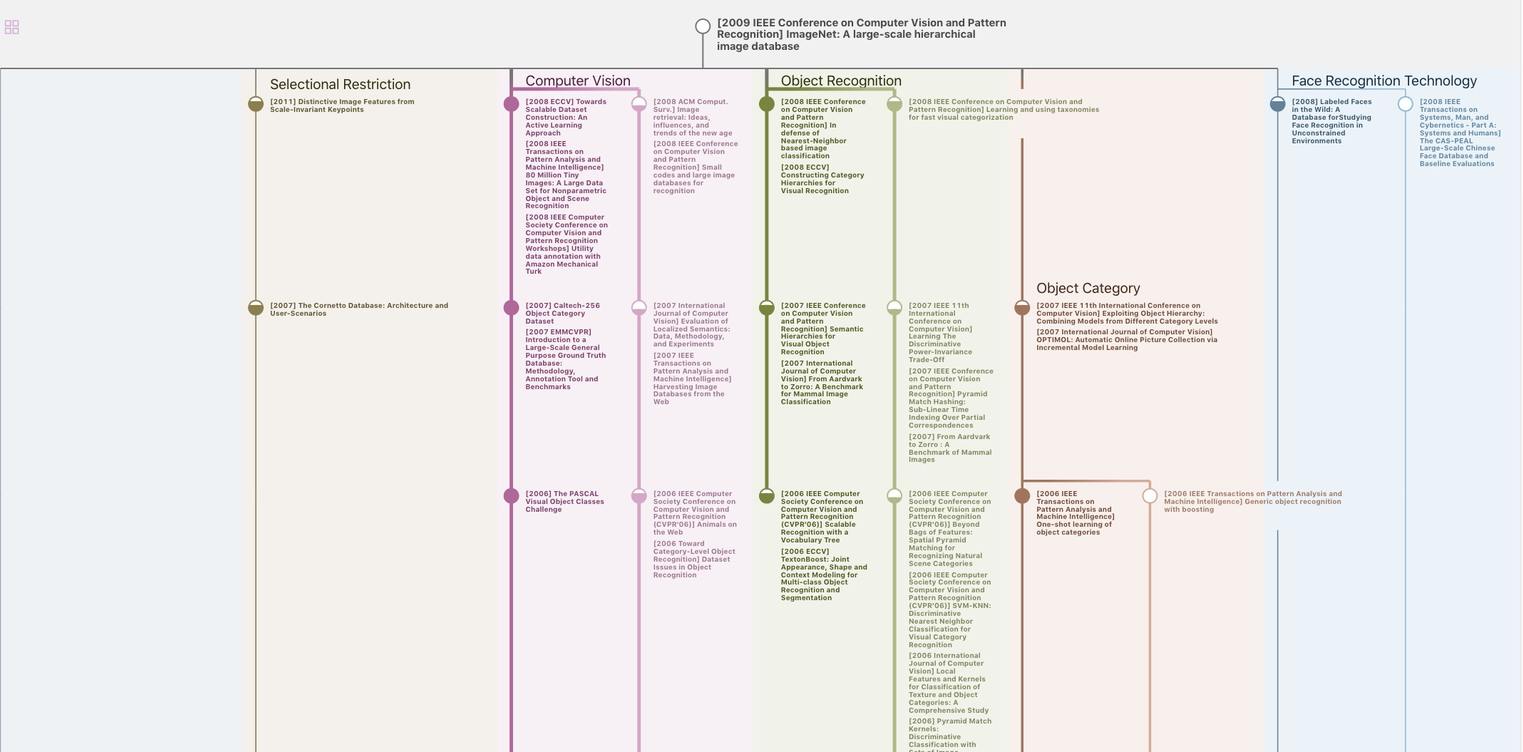
生成溯源树,研究论文发展脉络
Chat Paper
正在生成论文摘要