A Feature-Learning-Based Method For The Disease-Gene Prediction Problem
INTERNATIONAL JOURNAL OF DATA MINING AND BIOINFORMATICS(2020)
摘要
We predict disease-genes relations on the human interactome network using a methodology that jointly learns functional and connectivity patterns surrounding proteins. Contrary to other data structures, the interactome is characterised by high incompleteness and absence of explicit negative knowledge, which makes predictive tasks particularly challenging. To exploit at best latent information in the network, we propose an extended version of random walks, named Random Watcher-Walker (RW2), which is shown to perform better than other state-of-the-art algorithms. We also show that the performance of RW2 and other compared state-of-the-art algorithms is extremely sensitive to the interactome used, and to the adopted disease categorisations, since this influences the ability to capture regularities in presence of sparsity and incompleteness.
更多查看译文
关键词
network medicine, disease gene prediction, disease gene prioritisation, node embedding, random walks, graph-based methods, biological networks, complex diseases
AI 理解论文
溯源树
样例
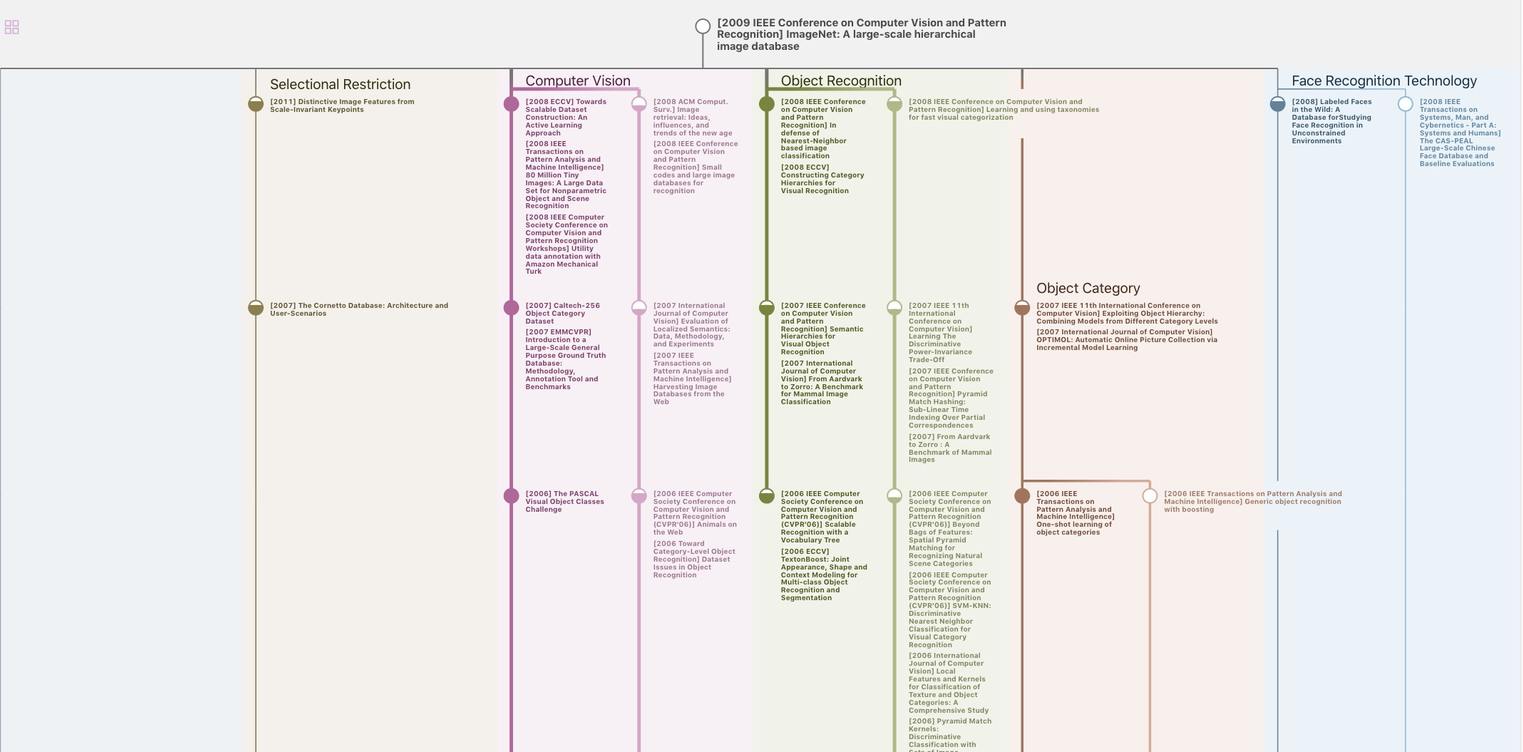
生成溯源树,研究论文发展脉络
Chat Paper
正在生成论文摘要