Classification Algorithms for Unbalanced High-Dimensional Data with Hyperbox Vertex Over-Sampling Iterative Support Vector Machine Approach
PROCEEDINGS OF THE 32ND 2020 CHINESE CONTROL AND DECISION CONFERENCE (CCDC 2020)(2020)
Abstract
This paper is written for addressing the bisectional classification problem for big and high-dimensional data sets with unbalanced characteristics distribution, whenever the support vector machine (SVM) algorithm is used. As is well known in conventional SVM, calculating large-scale covariance matrices and biased support vectors due to unbalanced characteristics are thorny issues that severely affect accuracy and efficiency of SVM classification algorithm in general. To surmount these problems, a class of modified SVM classification algorithm (hyperbox vertex over-sampling iterative SVM: HVS-ISVM) based on hyperbox vertex over-sampling data compensating and active iterative learning are innovatively proposed. The algorithm procedures are explained briefly and the main features are summarized, which are conducive to implementing and revealing deeper data indices. Numerical simulation results show that the proposed HVS-ISVM algorithm have higher calculation speed and better classification accuracy, and the obtained iterative support vectors and their corresponding hyperplanes are also robust against numerical noise due to over-sampling.
MoreTranslated text
Key words
hyperbox, vertex over-sampling, interval number, support vector machine, actively learning, unbalanced data
AI Read Science
Must-Reading Tree
Example
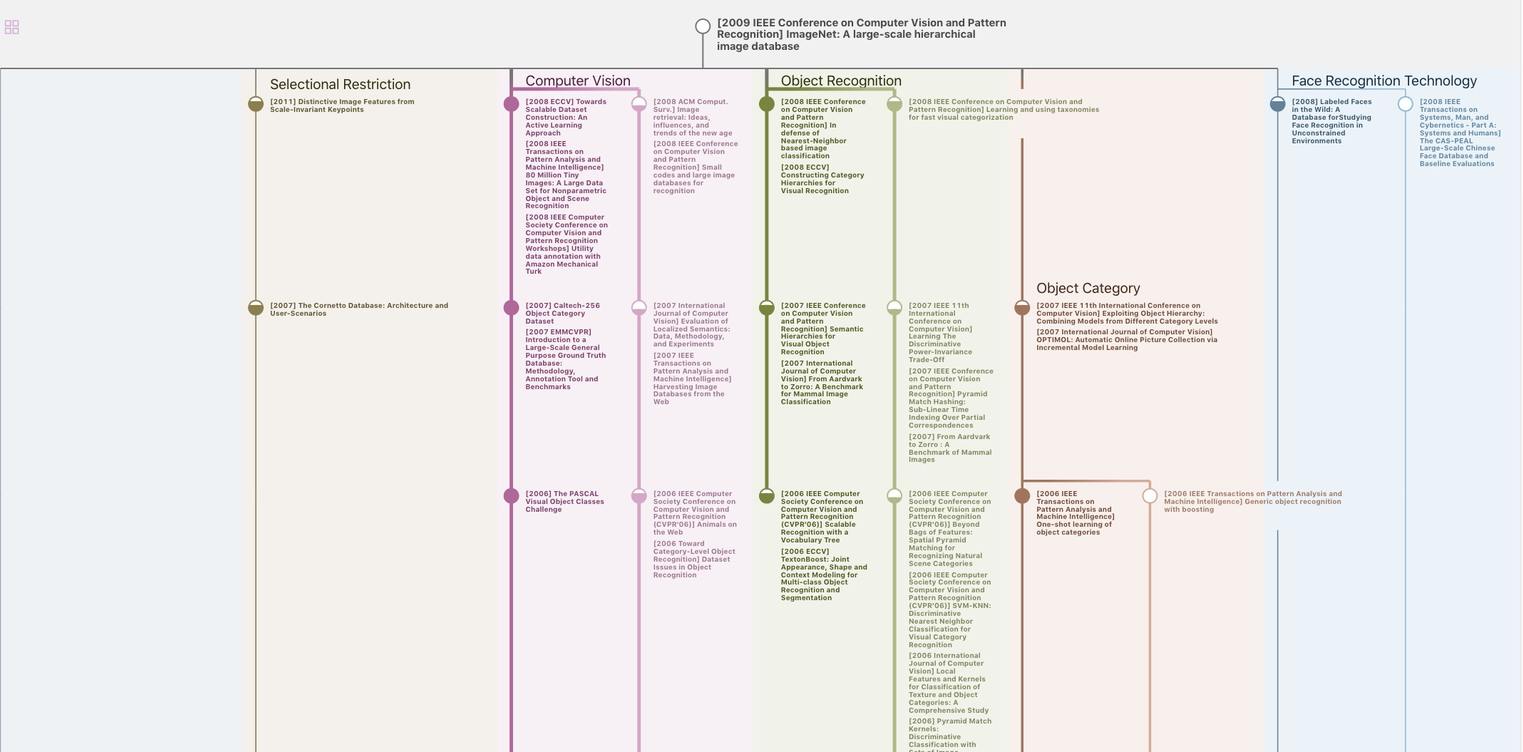
Generate MRT to find the research sequence of this paper
Chat Paper
Summary is being generated by the instructions you defined