Log Analytics In Hpc: A Data-Driven Reinforcement Learning Framework
IEEE INFOCOM 2020 - IEEE CONFERENCE ON COMPUTER COMMUNICATIONS WORKSHOPS (INFOCOM WKSHPS)(2020)
摘要
High Performance Computing (HPC) has been employed in many fields such as aerospace, weather forecast, numerical simulation, scientific research etc. Security of HPC, especially anomaly/intrusion detection, has attracted many attentions in recent years. Given the heavily instrumented property of HPC systems, logs become an effective and direct data source that can be utilized to evaluate the system status, further, to detect anomalies or malicious users. In this paper, we offer a novel perspective, treating the anomaly detection in HPC as a sequential decision process, and further applying reinforcement learning techniques to learn the state transition process, based on which we build a framework named as ReLog to detect anomalies or malicious users. Besides, a common challenge of employing machine learning techniques is lacking sufficient data, we provide a generative adversarial network (GAN)-based solution to generate sufficient training data in HPC. The experimental validations are conducted based on real-world collected MPI logs, and our results demonstrate a 93% of detection accuracy on the collected dataset.
更多查看译文
关键词
High performance computing, security, reinforcement learning, defenses and attacks, log analytics
AI 理解论文
溯源树
样例
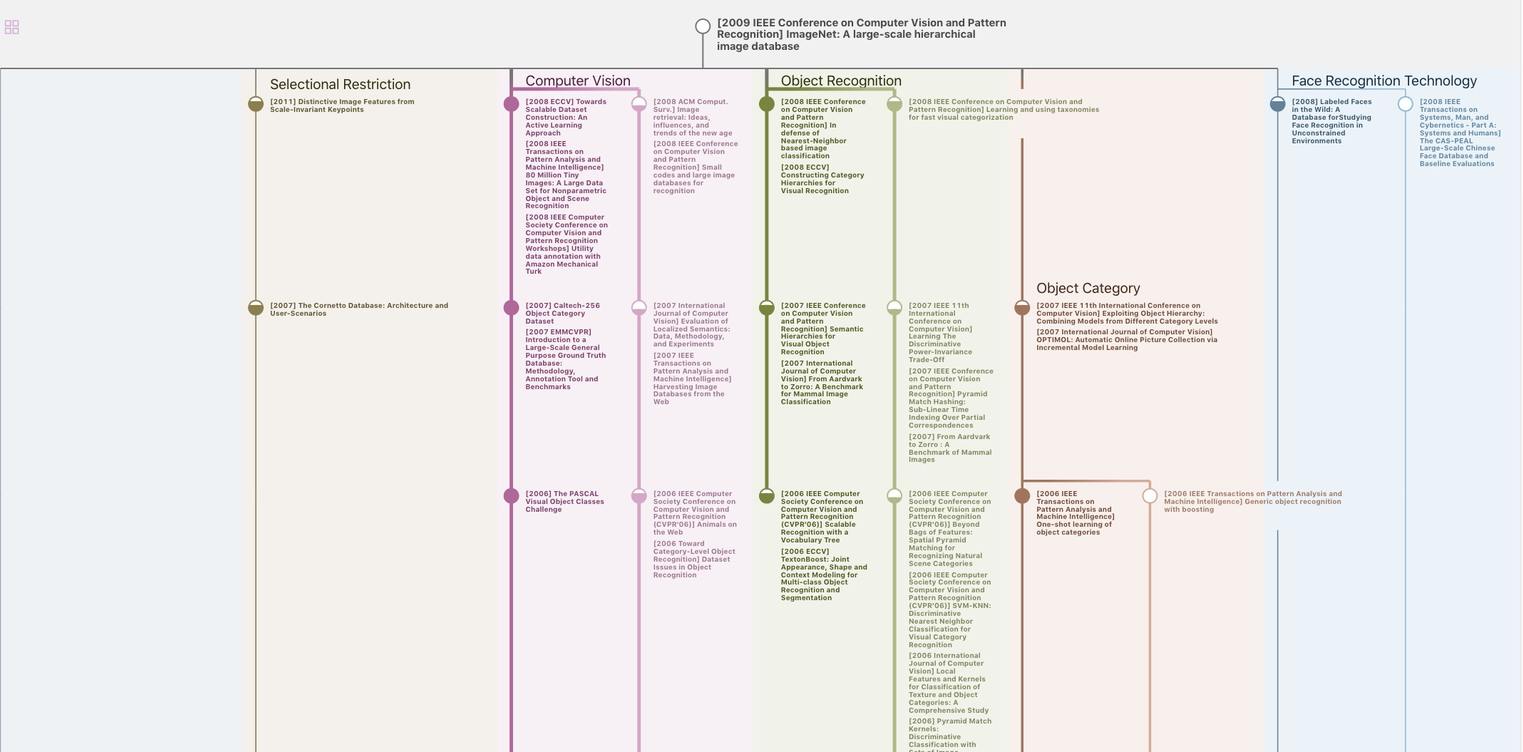
生成溯源树,研究论文发展脉络
Chat Paper
正在生成论文摘要