Pedestrian Detection with Cascaded Part Model for Occlusion Handling
International journal on advances in intelligent systems(2015)
Abstract
Pedestrian detection in a crowded environment under occlusion constraint is a challenging task. We have addressed this task by exploiting the properties of a rich feature set, which gives almost all cues necessary for recognizing pedestrians. Such rich feature set results in higher dimensional feature space. We have used partial least square regression to map these higher dimensional features to a lower dimensional yet discriminative feature space. Part model is further applied to deal with occlusions. The proposed method gives the best reported results on INRIA pedestrian dataset with detection accuracy of 98% at 10-4 False Positives Per Window (FPPW) and a miss rate of 31.62% at 10-1 False Positives Per Image (FPPI). We have also demonstrated the effectiveness of our part model under partial and heavily occluded conditions. Our proposed system outperforms several state of the art techniques under various evaluation conditions of INRIA pedestrian database. Keywords-Pedestrian detection; occlusion handling.
MoreTranslated text
AI Read Science
Must-Reading Tree
Example
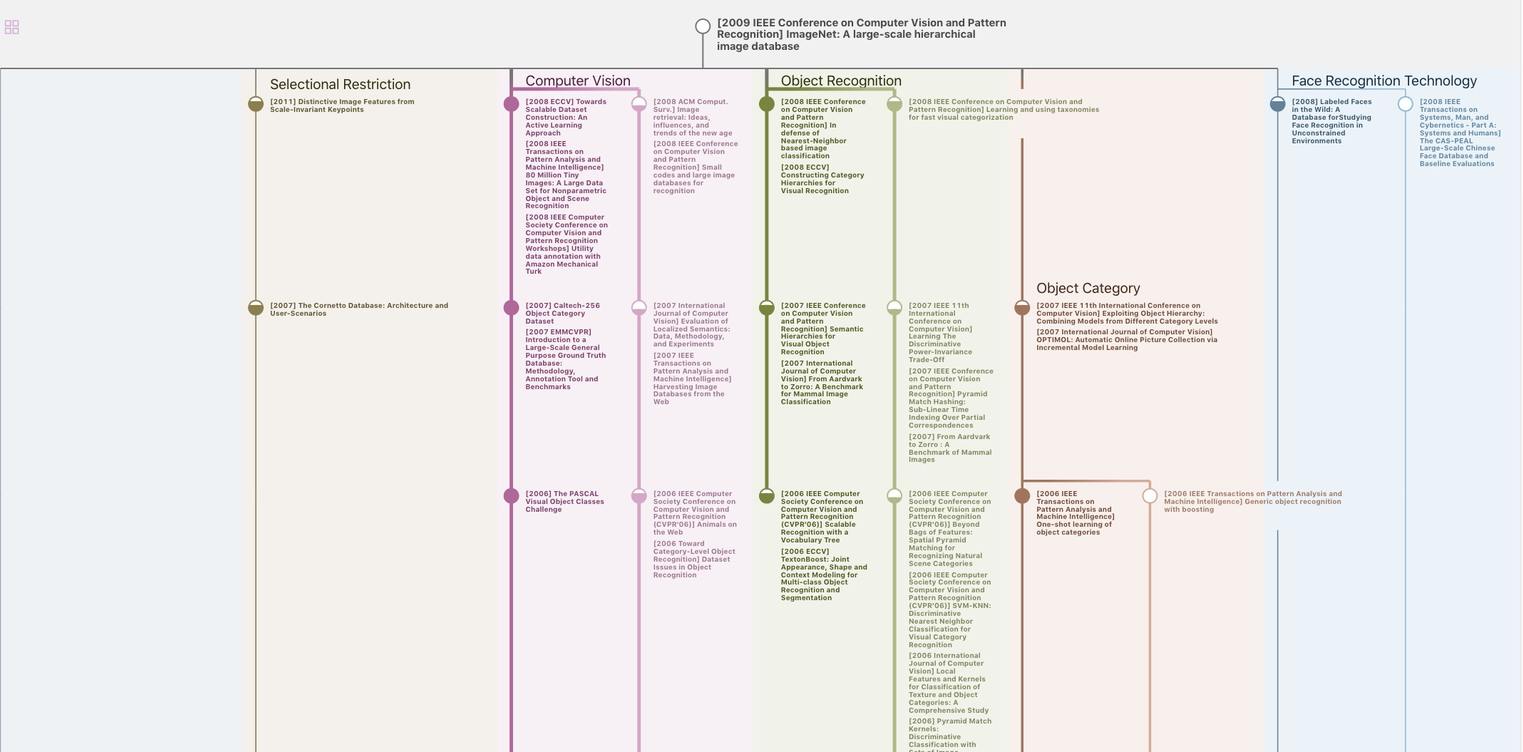
Generate MRT to find the research sequence of this paper
Chat Paper
Summary is being generated by the instructions you defined