Mitigating sentimental bias via a polar attention mechanism
INTERNATIONAL JOURNAL OF DATA SCIENCE AND ANALYTICS(2020)
Abstract
Fairness in machine learning has received increasing attention in recent years. This study focuses on a particular type of machine learning fairness, namely sentimental bias, in text sentiment analysis. Sentimental bias occurs on words (or phrases) when they are distributed distinctly in positive and negative corpora. It results in that an excessively proportion of words carry negative/positive sentiment in learned models. This study proposed a new attention mechanism, called polar attention, to mitigate sentimental biases. It consists of two modules, namely polar flipping and distance measurement. The first module explicitly models word sentimental polarity and can prevent that neutral words flip positively or negatively. The second module is used to attend negative/positive words. In the experiments, three benchmark data sets are used, and supplementary testing sets are compiled. Experimental results verify the effectiveness of the proposed method.
MoreTranslated text
Key words
Sentiment analysis, Sentimental bias, Attention, Polar flipping
AI Read Science
Must-Reading Tree
Example
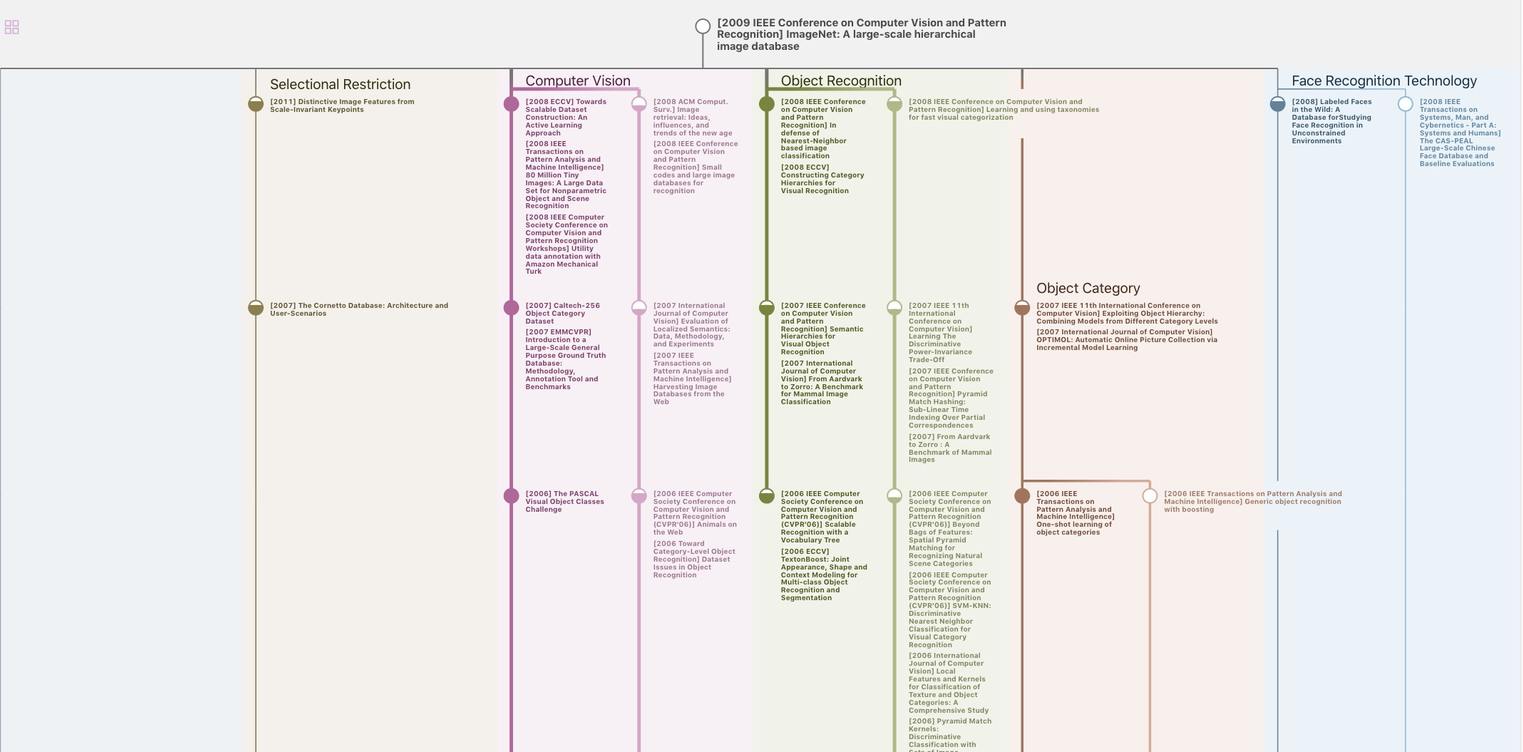
Generate MRT to find the research sequence of this paper
Chat Paper
Summary is being generated by the instructions you defined