Combining deep learning and model-based method using Bayesian Inference for walking speed estimation
BIOMEDICAL SIGNAL PROCESSING AND CONTROL(2020)
摘要
In this paper, deep learning and model-based method were combined using Bayesian Inference to realize high accuracy and good generalization capability stride-by-stride walking speed estimation by low cost inertial measurement unit (IMU) sensor. Long Short-Term Memory (LSTM) network was applied to train the prediction model because of its ability to consider the temporal correlation of multi-dimensional kinematic parameters during one stride. To improve the performance with unseen subjects, a model-based method was introduced for its relatively good generalization capability. Fusion strategy based on Bayesian Inference was applied to take advantage of the two methods which considered the estimation derived from different methods as abstract sensors. Six healthy young adults performed treadmill walking with shank-mounted IMU and the range of the walking speed was 2.5 km/h to 5 km/h at an increment of 0.5 km/h. Leave-one-subject-out (LOSO) crossvalidation was performed to analyze the generalization capability. For deep learning method, the root mean square error (RMSE) of the model trained by all available subjects was 0.026 m/s and the RMSE of LOSO cross-validation was 0.066 m/s which indicated a low generalization capability. After the fusion strategy was applied, RMSE of the model trained by all available subjects was 0.023 m/s which was slightly improved, while the RMSE of LOSO cross-validation was reduced to 0.036 m/s which indicated that accuracy and the generalization capability was greatly improved. In addition, this accurate estimation can be easily realized online which is essential for locomotion interactive systems (e.g. self-paced treadmill).
更多查看译文
关键词
Walking speed estimation,Deep learning,Bayesian Inference,Self-paced treadmill
AI 理解论文
溯源树
样例
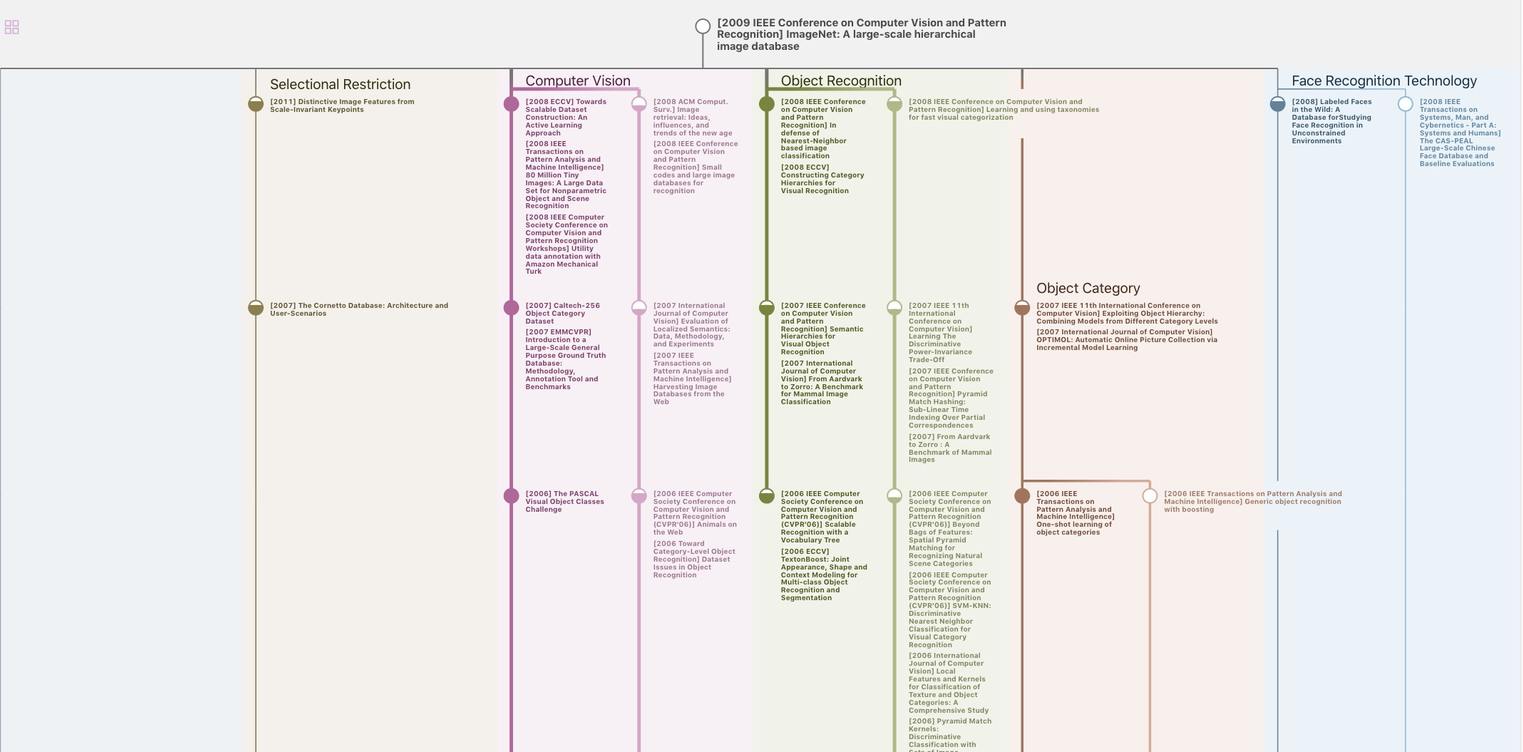
生成溯源树,研究论文发展脉络
Chat Paper
正在生成论文摘要