DI-Mondrian: Distributed improved Mondrian for satisfaction of the L-diversity privacy model using Apache Spark
Information Sciences(2021)
摘要
For the extraction of useful patterns, the collected data should be distributed to and shared with analyzers. This, however, creates problems and challenges for the individual with respect to their privacy and identity. In this paper, the Mondrian multidimensional anonymization method was developed and improved for satisfaction of the l-diversity privacy model, and it has been presented in a distributed fashion within the Apache Spark framework. Since one of the major challenges in data privacy is the tradeoff between privacy and data utility, the presented method focuses on information loss and classifier evaluation criteria. Therefore, the cut dimension was selected using the coefficient of variation and information gain criteria, and the cut points were chosen dynamically, which led to a decrease in the information loss parameter and an improvement in the classifier performance evaluation criteria such as accuracy and FMeasure compared to the previous algorithms in the literature. The processing speed is 100 times higher in Spark than in the Hadoop framework. Consequently, the proposed method was presented in a distributed fashion based on RDDs programming within Apache Spark framework. This will resolve the problem of speed in large-scale data anonymization as it exists in the previous Hadoop-based algorithms. The results of the experiments performed on the numerical datasets demonstrate the improvements made by the proposed method.
更多查看译文
关键词
Anonymization,PPDP,K-anonymity,L-diversity,Information loss,Apache Spark,RDD
AI 理解论文
溯源树
样例
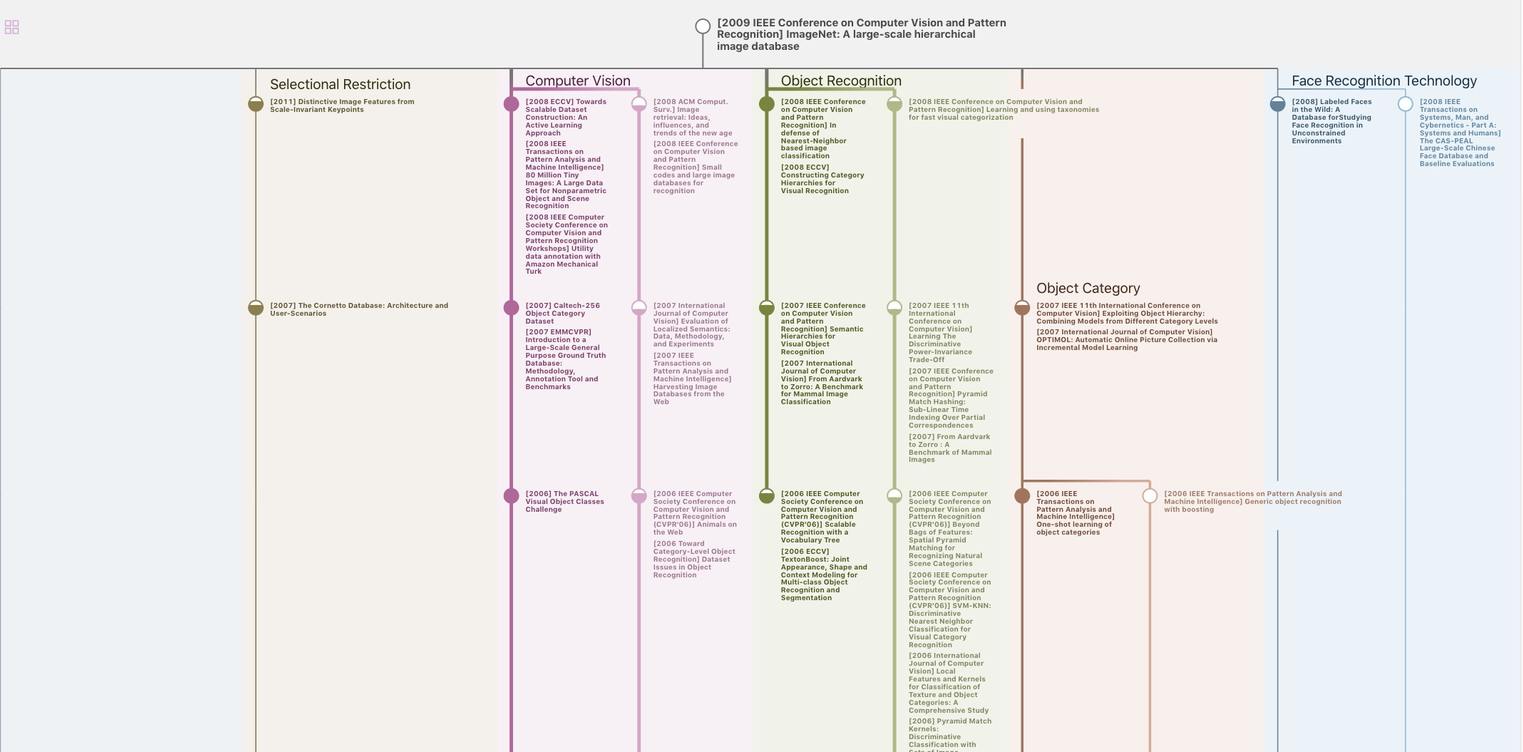
生成溯源树,研究论文发展脉络
Chat Paper
正在生成论文摘要