Vector-Based Feature Representations For Speech Signals: From Supervector To Latent Vector
IEEE TRANSACTIONS ON MULTIMEDIA(2021)
Abstract
There are two basic types of feature representations for speech signals. The first type refers to probabilistic models, such as the Gaussian mixture model (GMM). The second type refers to vector-based feature representations, such as the Gaussian supervector (GSV). Since vector-based feature representations are easier to use and process, they are more popular than probabilistic model-based feature representations. In this paper, we begin by explaining the rationale behind two widely used vector-based feature representations, viz. GSV and the i-vector, and then make extensions. GSV is a supervector (SV) based on maximum a posteriori (MAP) adaptation. Its computation is simple and fast, but its dimensionality is high and fixed. While the i-vector is a latent vector (LV) based on factor analysis (FA). Although the computation can be time-consuming because of additional model parameters, its dimensionality is changeable. To generalize GSV, we propose the MAP SV, which is also based on MAP adaptation but can have an even higher dimensionality and thus carry more information. To boost the computational efficiency of the i-vector, we adopt the concept of the mixture of factor analyzers (MFA) and propose the MFA LV, which exhibits a similar flexibility in dimensionality but is faster in computation. The experimental results for speaker identification and verification tasks demonstrate that, MAP SV can be more robust than GSV, and MFALV is comparable to or even better than the i-vector in effectiveness and meanwhile maintains a higher computational efficiency. With a powerful backend, GSV and MAP SV are comparable to the i-vector and MFALV, but the latter two are more flexible in dimensionality.
MoreTranslated text
Key words
Acoustics, Probabilistic logic, Computational modeling, Adaptation models, Computational efficiency, Task analysis, Speaker recognition, Acoustic and speech signal processing, gaussian supervector, i-vector, supervector and latent vector, vector-based feature representation
AI Read Science
Must-Reading Tree
Example
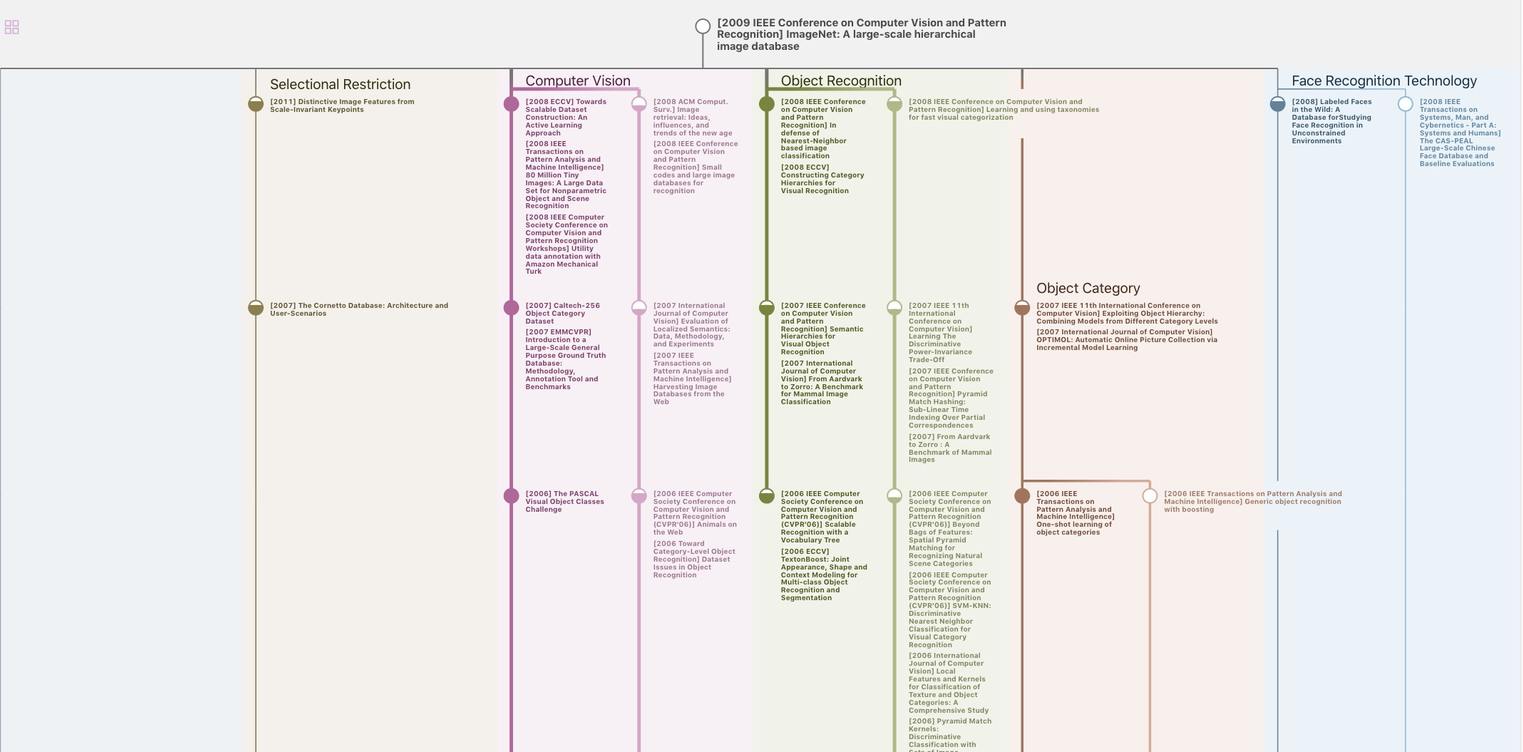
Generate MRT to find the research sequence of this paper
Chat Paper
Summary is being generated by the instructions you defined