STABILIZATION OF SENTINEL-1 SAR TIME-SERIES USING CLIMATE AND FOREST STRUCTURE DATA FOR EARLY TROPICAL DEFORESTATION DETECTION
ISPRS Annals of the Photogrammetry, Remote Sensing and Spatial Information Sciences(2020)
摘要
Abstract. In this study we analyse the factors of variability of Sentinel-1 C-band radar backscattering over tropical rainforests, and propose a method to reduce the effects of this variability on deforestation detection algorithms. To do so, we developed a random forest regression model that relates Sentinel-1 gamma nought values with local climatological data and forest structure information. The model was trained using long time-series of 26 relevant variables, sampled over 6 undisturbed tropical forests areas. The resulting model explained 71.64% and 73.28% of the SAR signal variability for VV and VH polarizations, respectively. Once the best model for every polarization was selected, it was used to stabilize extracted pixel-level data of forested and non-deforested areas, which resulted on a 10 to 14% reduction of time-series variability, in terms of standard deviation. Then a statistically robust deforestation detection algorithm was applied to the stabilized time-series. The results show that the proposed method reduced the rate of false positives on both polarizations, especially on VV (from 21% to 2%, α=0.01). Meanwhile, the omission errors increased on both polarizations (from 27% to 37% in VV and from 27% to 33% on VV, α=0.01). The proposed method yielded slightly better results when compared with an alternative state-of-the-art approach (spatial normalization).
更多查看译文
关键词
early tropical deforestation detection,forest structure data,sar,time-series
AI 理解论文
溯源树
样例
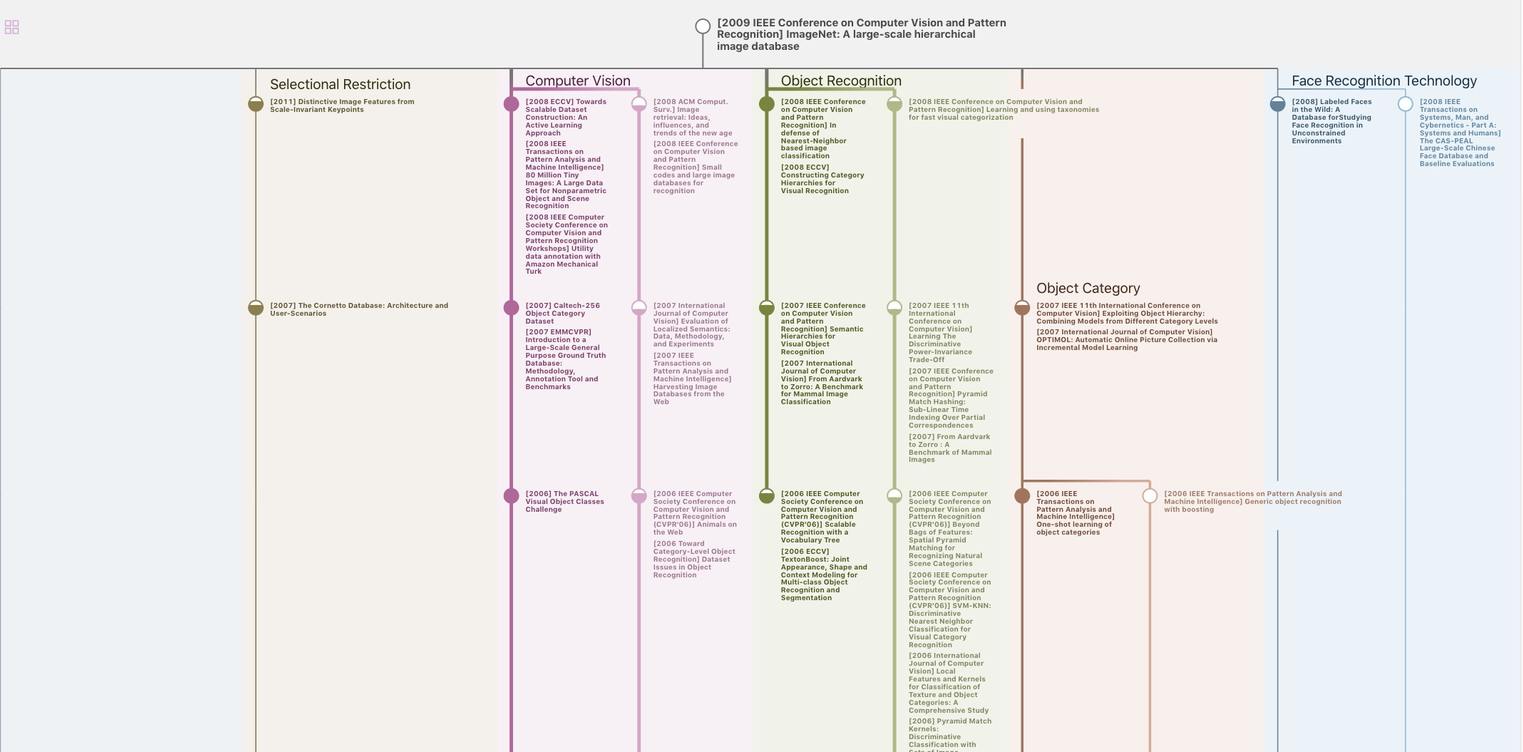
生成溯源树,研究论文发展脉络
Chat Paper
正在生成论文摘要