Privacy-preserving and Utility-aware Participant Selection for Mobile Crowd Sensing
Mobile Networks and Applications (MONET)(2020)
摘要
Mobile Crowd Sensing (MCS) is an emerging paradigm, it engages ordinary mobile device users to effectively contribute sensed data using mobile applications. The unique characteristics of MCS bring a lot of new challenges. First, contributing valuable data quality utilizes users’ mobile resources, and private information; making it essential to adequately reward the participants however the Service Provider (SP) usually has a limited budget. Second, sensitive information is vulnerable to information leaks (such as sensed data reports and sensing locations) as the sensing tasks in MCS are location-based; demanding a proper privacy protection scheme. Last, the SP targets to maximize the data utility, which implies not only the requirements of high-quality data but also large geographical coverage of sensing data, with a limited budget. To address the participants’ privacy and selection issues, this paper proposes a novel Privacy-preserving and utility-aware participant selection scheme PUPS. Firstly, we claim that the total data utility of a set of participants within a certain area should be calculated according to the data quality of each participant and the location coverage of the sensing data. Secondly, a participant selection scheme has been proposed, which determines a set of participants with maximum total data utility under the budget constraint, and shows that it is a Quadratic Integer Programming problem. We show that our optimization problem is a quadratic integer programming problem. Further to preserve the data qualities and location privacy of participants, homomorphic encryption-based euclidean distance and one-time padding schemes are integrated. Extensive simulations have been conducted to solve the selection problem. Through performance evaluation, we demonstrate the accuracy, efficacy, and scalability of PUPS by comparing it with three other selection schemes; Data Quality First Selection (DQFS), Lowest Bid Price First Selection (LBFS), and Random Selection (RS), respectively. The simulation results further show that our proposed scheme is effective and offers a higher level of privacy protection and maximum data utility.
更多查看译文
关键词
Mobile crowd sensing,Privacy,Utility,Budget,Homomorphic encryption,One-time padding
AI 理解论文
溯源树
样例
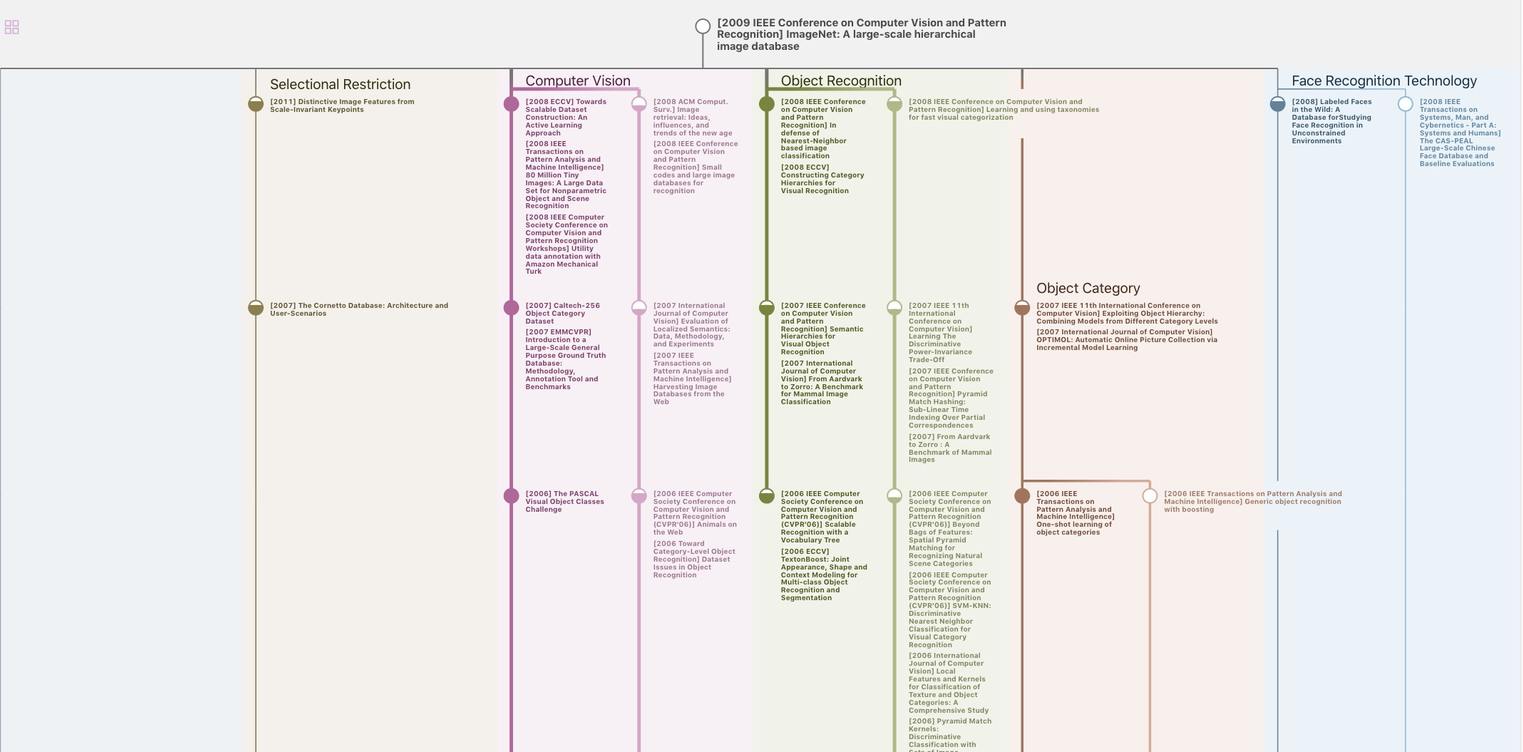
生成溯源树,研究论文发展脉络
Chat Paper
正在生成论文摘要