An Efficient and Effective Approach for Flooding Attack Detection in Optical Burst Switching Networks
SECURITY AND COMMUNICATION NETWORKS(2020)
摘要
Optical burst switching (OBS) networks are frequently compromised by attackers who can flood the networks with burst header packets (BHPs), causing a denial of service (DoS) attack, also known as a BHP flooding attack. Nowadays, a set of machine learning (ML) methods have been embedded into OBS core switches to detect these BHP flooding attacks. However, due to the redundant features of BHP data and the limited capability of OBS core switches, the existing technology still requires major improvements to work effectively and efficiently. In this paper, an efficient and effective ML-based security approach is proposed for detecting BHP flooding attacks. The proposed approach consists of a feature selection phase and a classification phase. The feature selection phase uses the information gain (IG) method to select the most important features, enhancing the efficiency of detection. For the classification phase, a decision tree (DT) classifier is used to build the model based on the selected features of BHPs, reducing the overfitting problem and improving the accuracy of detection. A set of experiments are conducted on a public dataset of OBS networks using 10-fold cross-validation and holdout techniques. Experimental results show that the proposed approach achieved the highest possible classification accuracy of 100% by using only three features.
更多查看译文
AI 理解论文
溯源树
样例
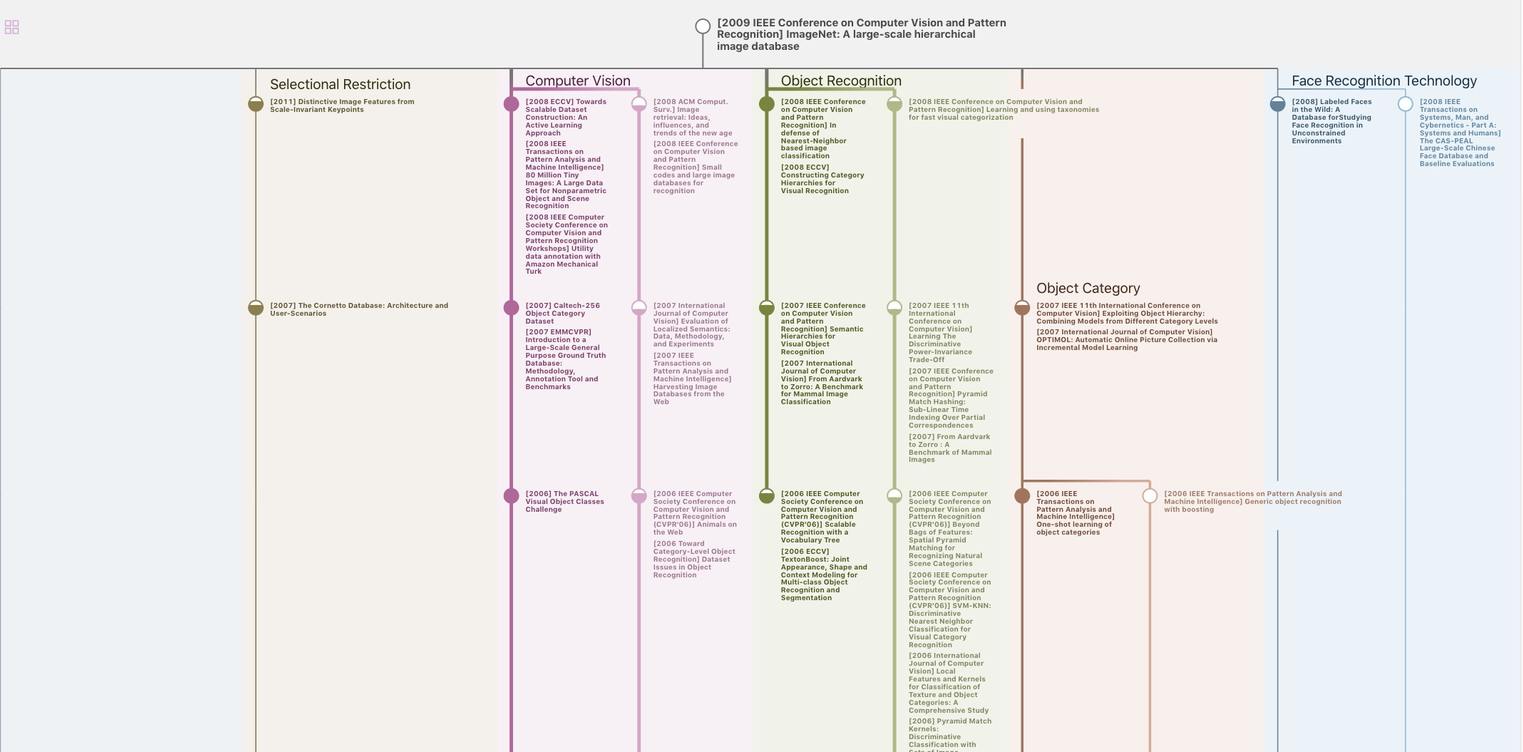
生成溯源树,研究论文发展脉络
Chat Paper
正在生成论文摘要