Classification-based fraud detection for payment marketing and promotion.
COMPUTER SYSTEMS SCIENCE AND ENGINEERING(2020)
摘要
Nowadays, many payment service providers use the discounts and other marketing strategies to promote their products. This also raises the issue of people who deliberately take advantage of such promotions to reap financial benefits. These people are known as 'scalper parties' or 'econnoisseurs' which can constitute an underground industry. In this paper, we show how to use machine learning to assist in identifying abnormal scalper transactions. Moreover, we introduce the basic methods of Decision Tree and Boosting Tree, and show how these classification methods can be applied in the detection of abnormal transactions. In addition, we introduce a graph computing method, which implicitly describes the characteristics of people and merchants through node correlation, in order to mine deep features. Because of the volume of large data, we carried out reasonable block calculation, and succeeded in reducing a large amount of data to a series of segments, thereby decreasing the computational resources and memory requirements. Compared with other work on abnormal transaction detection, we pay more attention to creating and using the portraits of merchants or individuals to assist in decision-making. After data analysis and model building, we find that focusing on only one transaction or one day does not yield a comprehensive number of characteristics, and many characteristics can be obtained by examining the transactions of a person or a merchant over a period of time. Furthermore, a large number of characteristics can be obtained from transactions in a period of time. After GBDT (Gradient Boosting Decision Tree) based classification prediction and analysis, we can conclude that there is a clear distinction between abnormal trading shops and conventional shops, facilitating the clustering of abnormal merchants. By filtering transaction data from multiple dimensions, multiple sub-graphs can be obtained. After hierarchical clustering, the abnormal trading group is mined and classified according to its features. Finally, we build a scoring model and apply it to the big data platform of one of China's largest payment service providers to help enterprises identify abnormal trading groups and specific marketing strategies.
更多查看译文
关键词
Payment fraud detection,Outlier detection,Gradient boosting decision tree,Machine learning
AI 理解论文
溯源树
样例
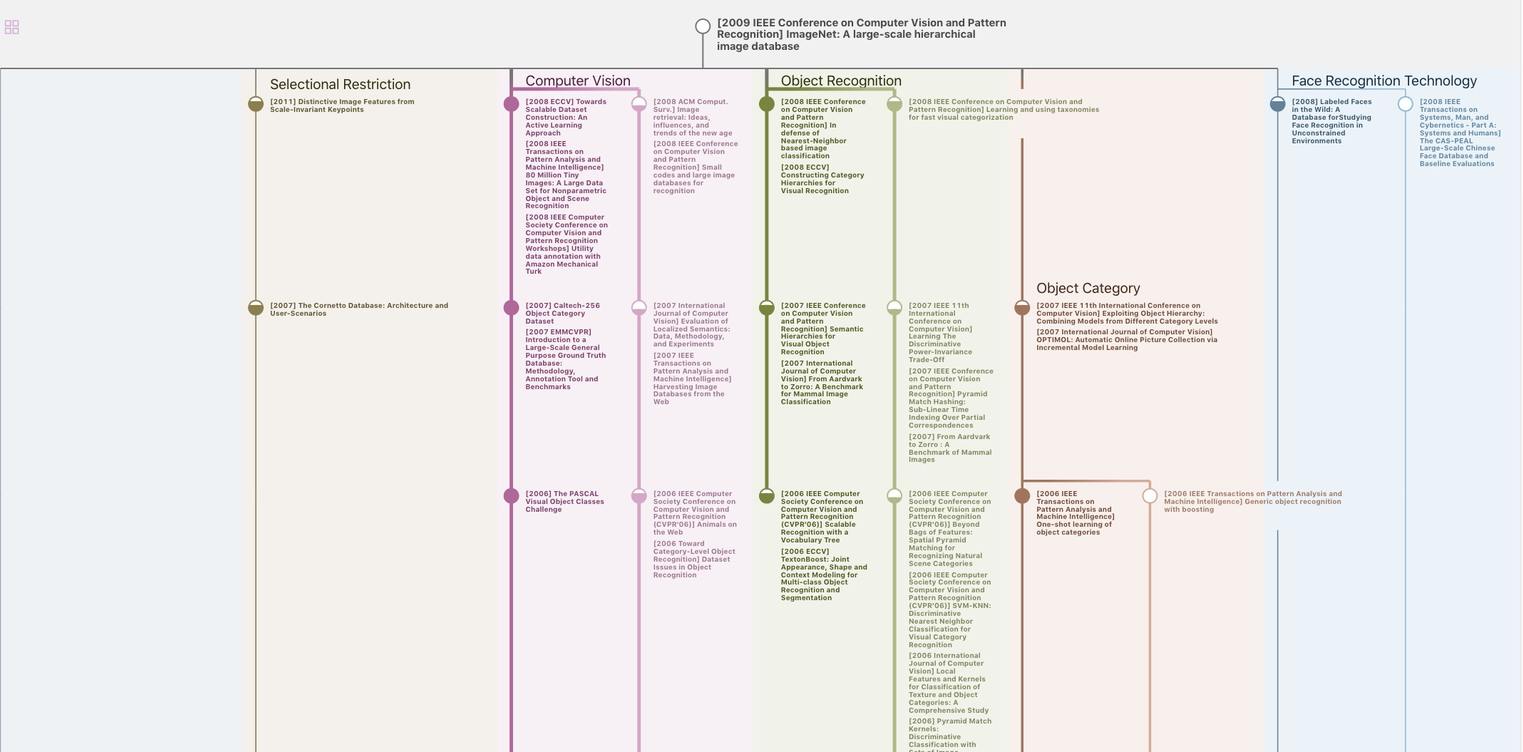
生成溯源树,研究论文发展脉络
Chat Paper
正在生成论文摘要