Discrete-Time Recurrent Neural Network for Solving Bound-Constrained Time-Varying Underdetermined Linear System
IEEE Transactions on Industrial Informatics(2021)
摘要
A typical recurrent neural network (RNN) has been developed for the online solution of a time-varying underdetermined linear system (TVULS) with bound constraint. This article provides a complete investigation by proposing a new discrete-time RNN (DTRNN) model to solve the bound-constrained TVULS. In particular, the continuous-time RNN (CTRNN) model in the existing literature for solving the bound-constrained TVULS is presented. The new DTRNN model is established and investigated by utilizing the Taylor difference formula to discretize the CTRNN model for determining the solution of the TVULS with bound constraint. Theoretical analysis and numerical results are provided to validate the effectiveness and superiority of the proposed DTRNN model. The model applicability is substantiated through the simulations on a PUMA560 robotic manipulator using the proposed DTRNN model.
更多查看译文
关键词
Bound constraint,discrete-time recurrent neural network (DTRNN),robotic manipulator,Taylor difference formula,time-varying underdetermined linear system (TVULS)
AI 理解论文
溯源树
样例
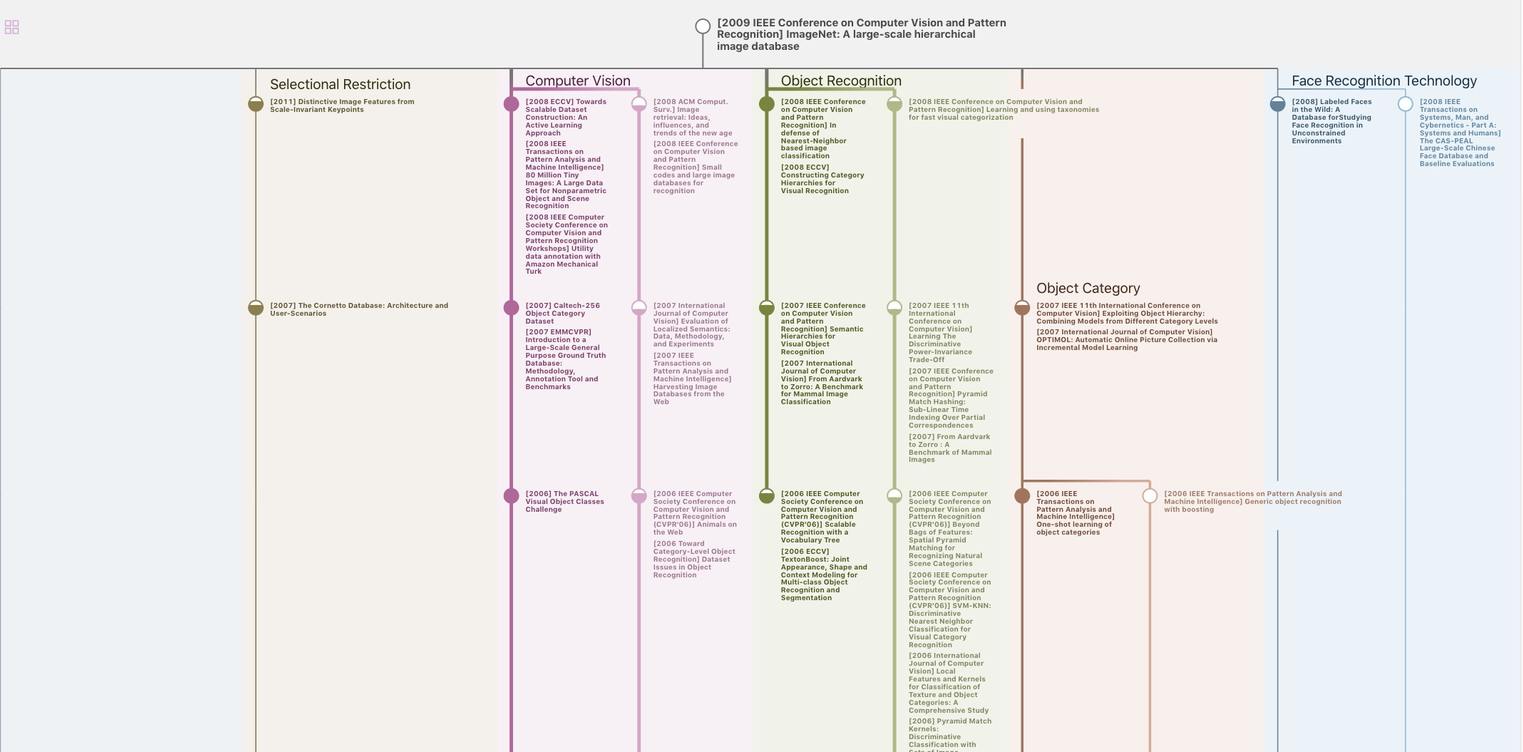
生成溯源树,研究论文发展脉络
Chat Paper
正在生成论文摘要