Short-Term Traffic Flow Prediction Based On Sparse Regression And Spatio-Temporal Data Fusion
IEEE ACCESS(2020)
摘要
Traffic flow forecasting is an important part of intelligent transportation systems. Accurate traffic flow forecasting can not only provide travel advice for people, but also improve traffic management efficiency. In this paper, we propose a novel traffic flow prediction model based on sparse regression, which can effectively and conveniently combine spatio-temporal factor. We analyze the traffic flow data, and design a multi-factor dictionary with spatio-temporal factor. Temporal factor and spatial factor are added to the multi-factor dictionary in the form of basis vectors which make the model easy to expand. The multi-factor coefficient solution based on LASSO guarantees the sparsity of the coefficients, which can quantitatively analyze the influence of spatio-temporal factor. Experimental results in urban and suburban scenarios indicate that the prediction accuracy is improved after adding the temporal factor and spatial factor, and traffic flow on urban roads is more susceptible to spatial factors. We compared our method with other models in three different prediction intervals, and the experimental results show that our method achieved the best prediction performance.
更多查看译文
关键词
Predictive models,Dictionaries,Correlation,Data models,Forecasting,Urban areas,Spatial databases,Traffic flow prediction,multi-factor analysis,sparse regression,dictionary construct
AI 理解论文
溯源树
样例
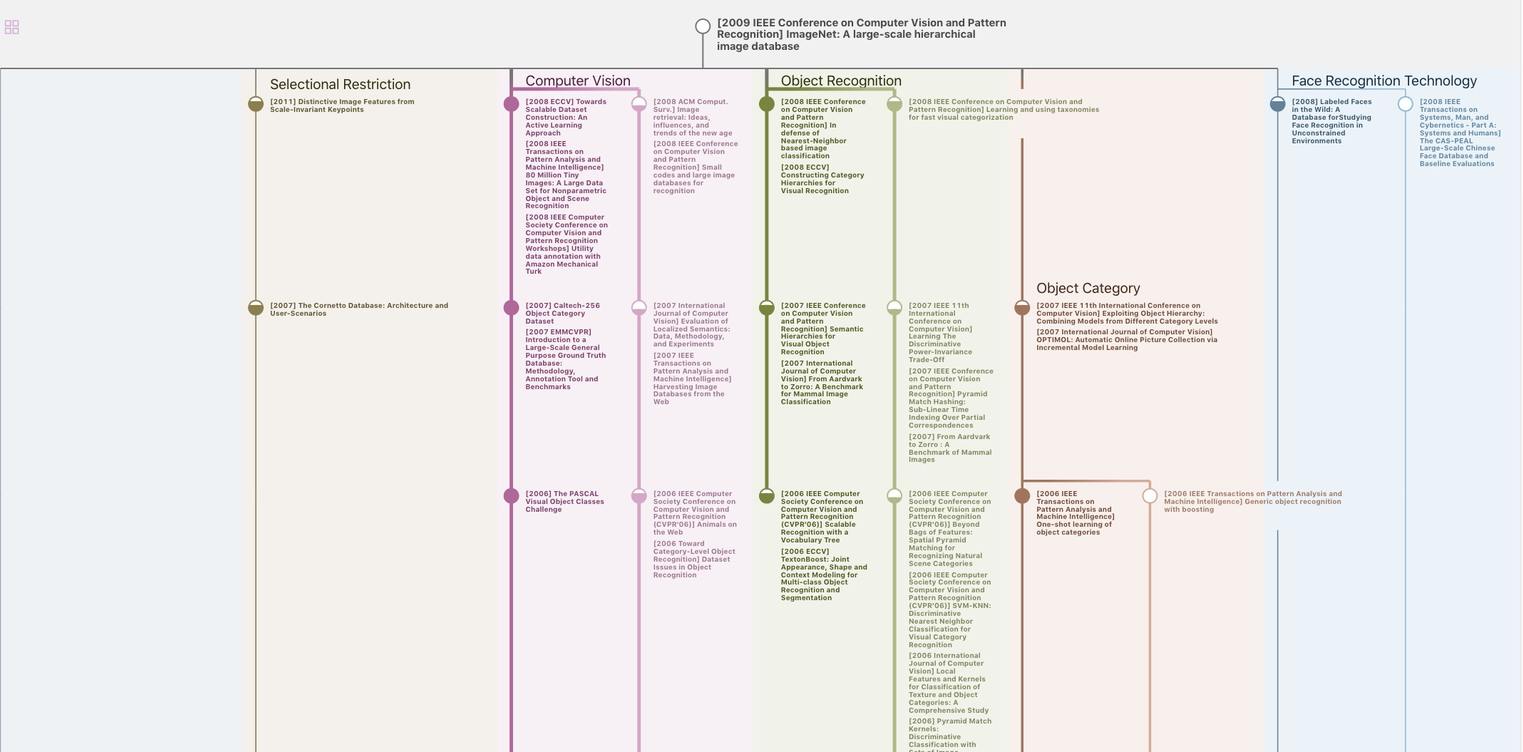
生成溯源树,研究论文发展脉络
Chat Paper
正在生成论文摘要