A review study of functional autoregressive models with application to energy forecasting
Periodicals(2021)
摘要
AbstractAbstractIn this data‐rich era, it is essential to develop advanced techniques to analyze and understand large amounts of data and extract the underlying information in a flexible way. We provide a review study on the state‐of‐the‐art statistical time series models for univariate and multivariate functional data with serial dependence. In particular, we review functional autoregressive (FAR) models and their variations under different scenarios. The models include the classic FAR model under stationarity; the FARX and pFAR model dealing with multiple exogenous functional variables and large‐scale mixed‐type exogenous variables; the vector FAR model and common functional principal component technique to handle multiple dimensional functional time series; and the warping FAR, varying coefficient‐FAR and adaptive FAR models to handle seasonal variations, slow varying effects and the more challenging cases of structural changes or breaks respectively. We present the models’ setup and detail the estimation procedure. We discuss the models’ applicability and illustrate the numerical performance using real‐world data of high‐resolution natural gas flows in the high‐pressure gas pipeline network of Germany. We conduct 1‐day and 14‐days‐ahead out‐of‐sample forecasts of the daily gas flow curves. We observe that the functional time series models generally produce stable out‐of‐sample forecast accuracy.This article is categorized under:Statistical Models > Semiparametric ModelsData: Types and Structure > Time Series, Stochastic Processes, and Functional Data View Figure In this data‐rich era, it is essential to develop advanced techniques to analyze and understand large amounts of data and extract the underlying information in a flexible way. We provide a review study on the state‐of‐the‐art statistical time series models for univariate and multivariate functional data with serial dependence. In particular, we review functional autoregressive (FAR) models and their variations dealing with different scenarios including multivariate functional series, high‐dimensionality, seasonal variations, nonstationarity and causal relation to exogenous variables. We discuss the models' applicability and conduct out‐of‐sample forecasts using real‐world data of high‐resolution natural gas flows in the high‐pressure gas pipeline network of Germany.
更多查看译文
关键词
energy forecast, functional autoregressive modeling, functional time series, sieve estimation
AI 理解论文
溯源树
样例
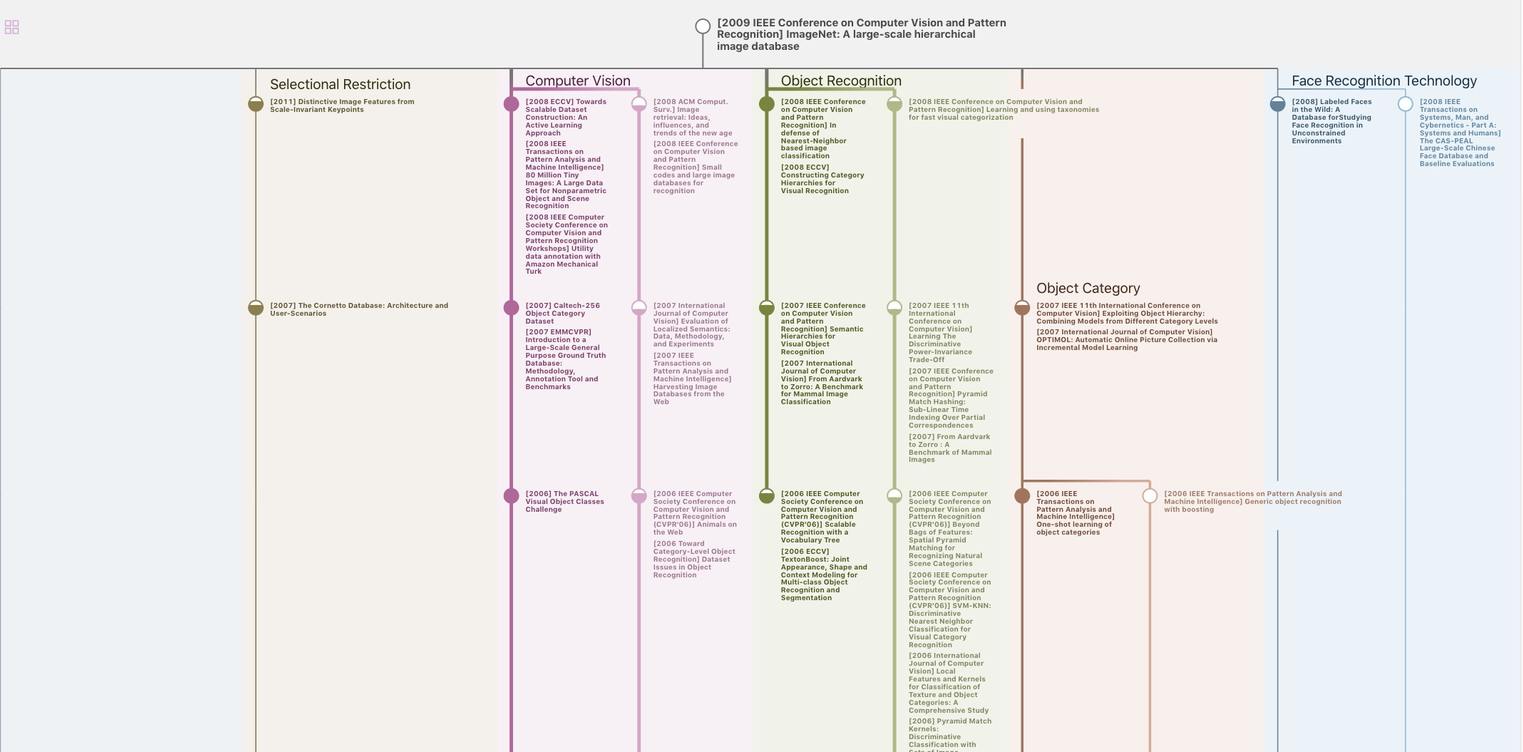
生成溯源树,研究论文发展脉络
Chat Paper
正在生成论文摘要