A Mask-Pooling Model With Local-Level Triplet Loss For Person Re-Identification
IEEE ACCESS(2020)
Abstract
Person Re-Identification (ReID) is an important yet challenging task in computer vision. Background clutter is one of the greatest challenges to overcome. In this paper, we propose a Mask-pooling model with local-level triplet loss (MPM-LTL) to tackle this problem and improve person ReID performance. Specifically, we present a novel pooling method, called mask pooling (MP), to gradually remove background features in feature maps through deep convolutional network. With mask pooling, the network can learn the most crucial person features. Moreover, we raise a novel local-level triplet loss for discriminative feature training. Furthermore, we propose a new hard triplets selection algorithm named Mask-guided TriHard. The method is based on human outline information, which is, to our best knowledge, employed for the first time for hard triplets selection. We achieve the state-of-the-art results on three benchmark person datasets Market-1501 [1], CUHK03 [2] and DukeMTMC-reID [3], [4].
MoreTranslated text
Key words
Feature extraction,Training,Image segmentation,Computational modeling,Cameras,Image resolution,Body regions,Person re-identification,mask-pooling,hard triplets selection,local-level triplet loss
AI Read Science
Must-Reading Tree
Example
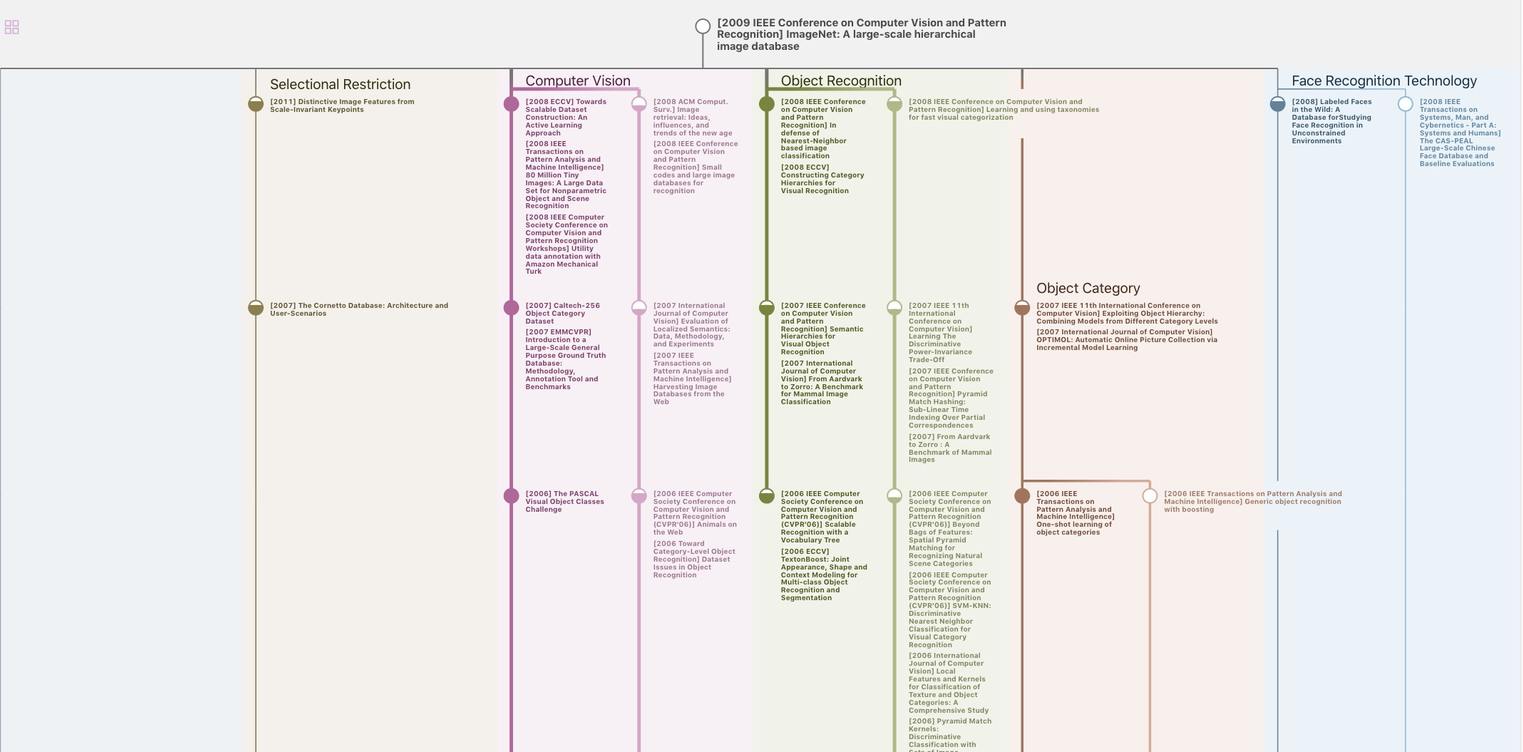
Generate MRT to find the research sequence of this paper
Chat Paper
Summary is being generated by the instructions you defined