Inversion of well logs into lithology classes accounting for spatial dependencies by using hidden markov models and recurrent neural networks
Journal of Petroleum Science and Engineering(2021)
摘要
Lithology is a crucial factor in reservoir characterization. Due to the limited availability of cores, the classes of the subsurface lithologies in boreholes need to be predicted from indirect measurements like well logs. However, the spatial interdependence between sediments and the spatial coupling between the well logs data pose challenges in this lithology classification. Numerous proposed classifiers are based on spatial element-wise independence and these classifiers usually fail to provide accurate predictions. In this study, we focus on two classification models from the Bayesian and the deep learning framework, which both take spatial context into account. We discuss a kernel-based hidden Markov (HM) model and a kind of recurrent neural network model named gated recurrent unit (GRU). Cross-validation results from these two models of three partially cored real wells are compared to result from a simple non-spatial deep neural network (DNN) model. The cross-validation results indicate that the lithology classifiers from models taking vertical spatial dependency into account are much more reliable in terms of classification accuracy and geological interpretation. The probabilistically defined HM model performs better than the neural network GRU model.
更多查看译文
关键词
Hidden markov model,Recurrent neural network,Lithology classification,Well logs inversion
AI 理解论文
溯源树
样例
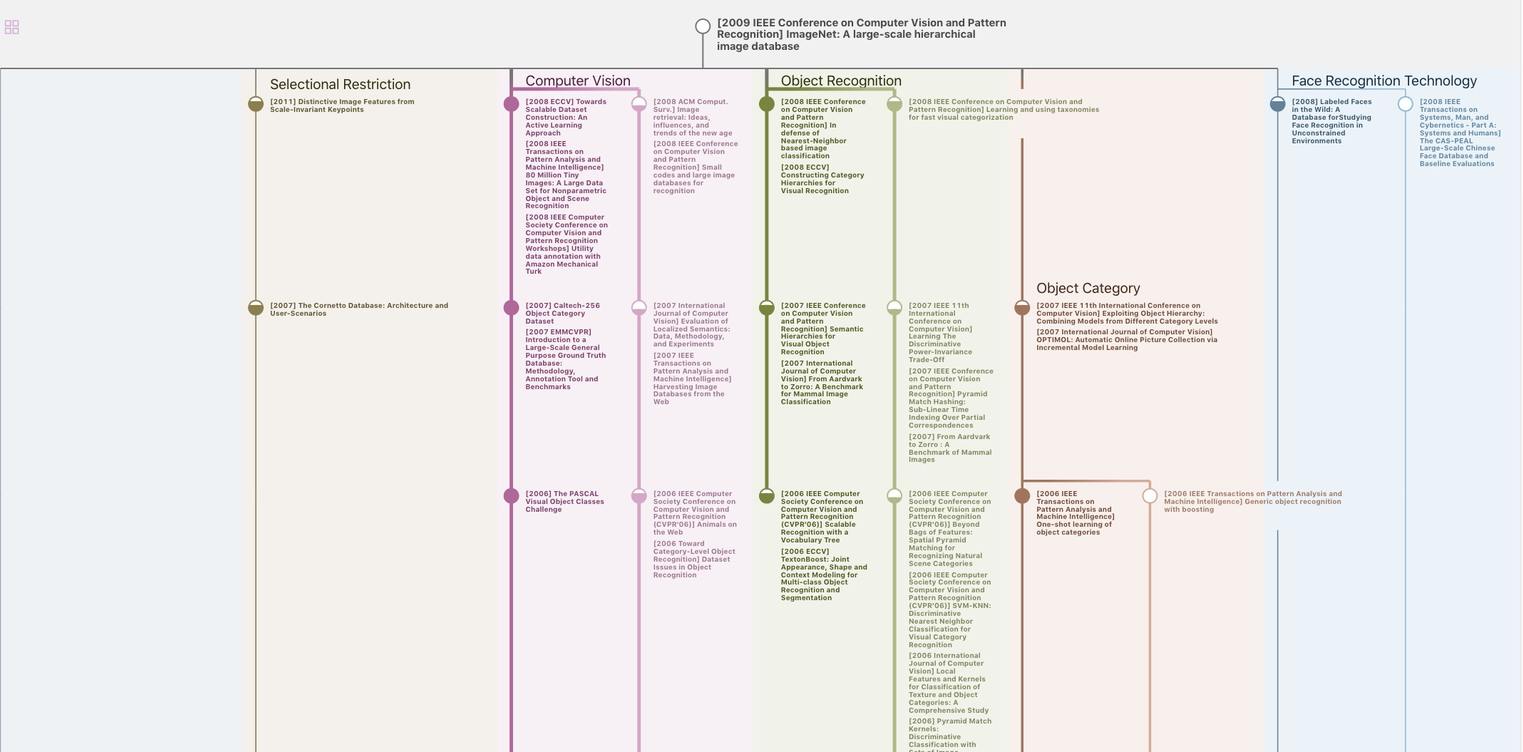
生成溯源树,研究论文发展脉络
Chat Paper
正在生成论文摘要