A Bayesian Deep Learning Rul Framework Integrating Epistemic And Aleatoric Uncertainties
IEEE TRANSACTIONS ON INDUSTRIAL ELECTRONICS(2021)
Abstract
Recent years have witnessed the prominent advancements of deep learning (DL) in the arsenal of prognostics and health management. However, the prognostic uncertainty problem extensively existed in industrial devices is not addressed by most DL approaches. This article formulates a novel Bayesian Deep Learning (BDL) framework to characterize the prognostic uncertainties. A distinguished advantage of the framework is its capacity of capturing the comprehensive effects of two critical uncertainties: 1) epistemic uncertainty, accounting for the uncertainty in the model, and 2) aleatoric uncertainty, representing the impact of random disturbance, such as measurement errors. The former arises from the variability of the model weights, and the latter is characterized by selected lifetime distributions. We integrate both uncertainties by defining BDL as priors of lifetime parameters. A sequential Bayesian boosting algorithm is executed to improve the estimation accuracy and compress the credible intervals. The superior prediction performance of our framework is validated by a real-world dataset collected from hydraulic mechanisms of circuit breakers.
MoreTranslated text
Key words
Uncertainty, Bayes methods, Prognostics and health management, Probabilistic logic, Machine learning, Modeling, Prediction algorithms, Aleatoric uncertainty, Bayesian neural network, epistemic uncertainty, remaining useful life
AI Read Science
Must-Reading Tree
Example
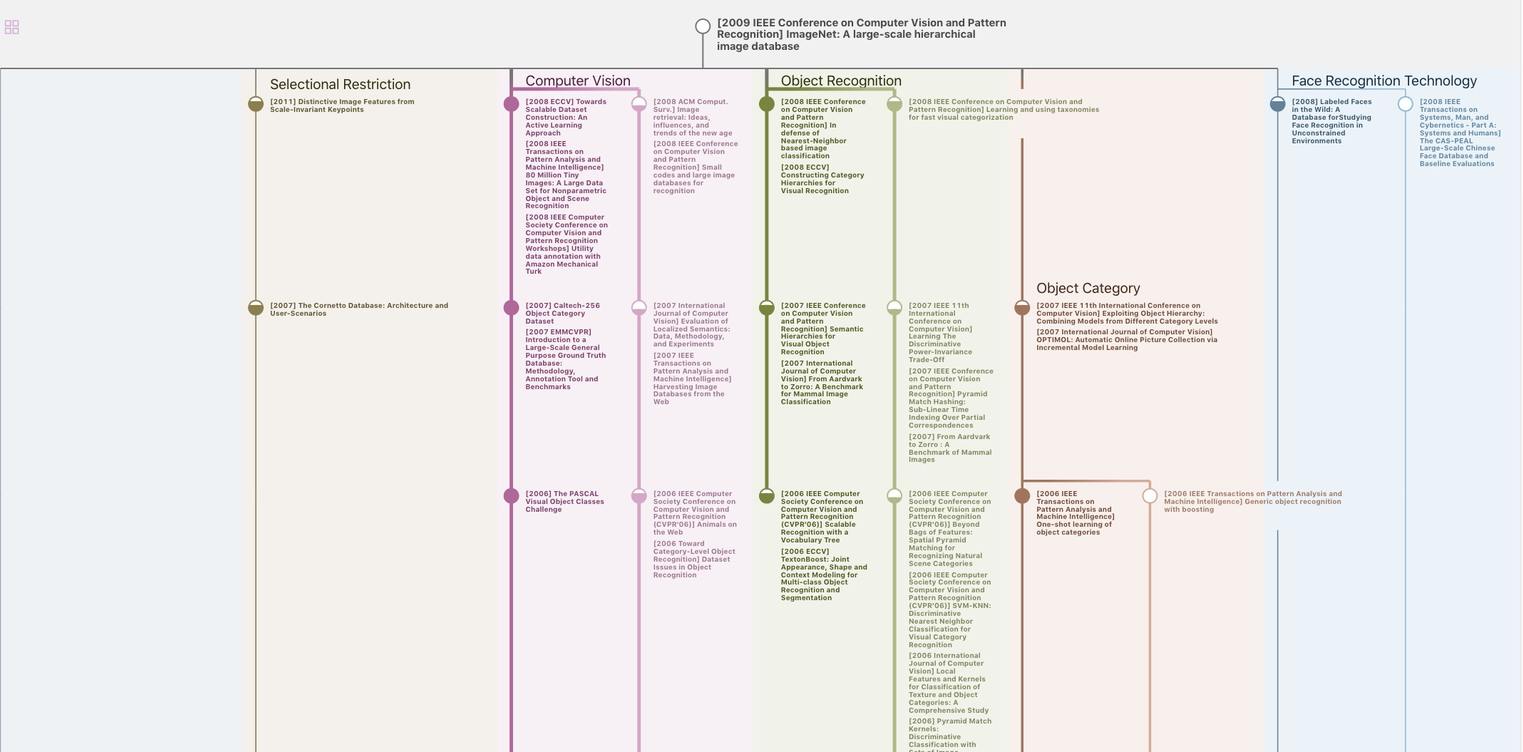
Generate MRT to find the research sequence of this paper
Chat Paper
Summary is being generated by the instructions you defined