Gauging human visual interest using multiscale entropy analysis of EEG signals
JOURNAL OF AMBIENT INTELLIGENCE AND HUMANIZED COMPUTING(2020)
摘要
Gauging human emotion can be of great benefit in many applications, such as marketing, gaming, and medicine. In this paper, we build a machine learning model that estimates the enjoyment and visual interest level of individuals experiencing museum content. The input to the model is comprised of 8-channel electroencephalogram signals, which we processed using multiscale entropy analysis to extract three features: the mean, slope of the curve, and complexity index (i.e., the area under the curve). Then, the number of features was drastically reduced using principle component analysis without a notable loss of accuracy. Multivariate analysis of variance showed that there exists a statistically significant correlation (i.e., p < 0.05 ) between the extracted features and the enjoyment level. Moreover, the classification model was able to predict the enjoyment level with a mean squared error of 0.1474 and an accuracy of 98.0
更多查看译文
关键词
Human–computer interaction,Electroencephalogram,Artificial neural networks,Emotion,Enjoyment,Multiscale entropy
AI 理解论文
溯源树
样例
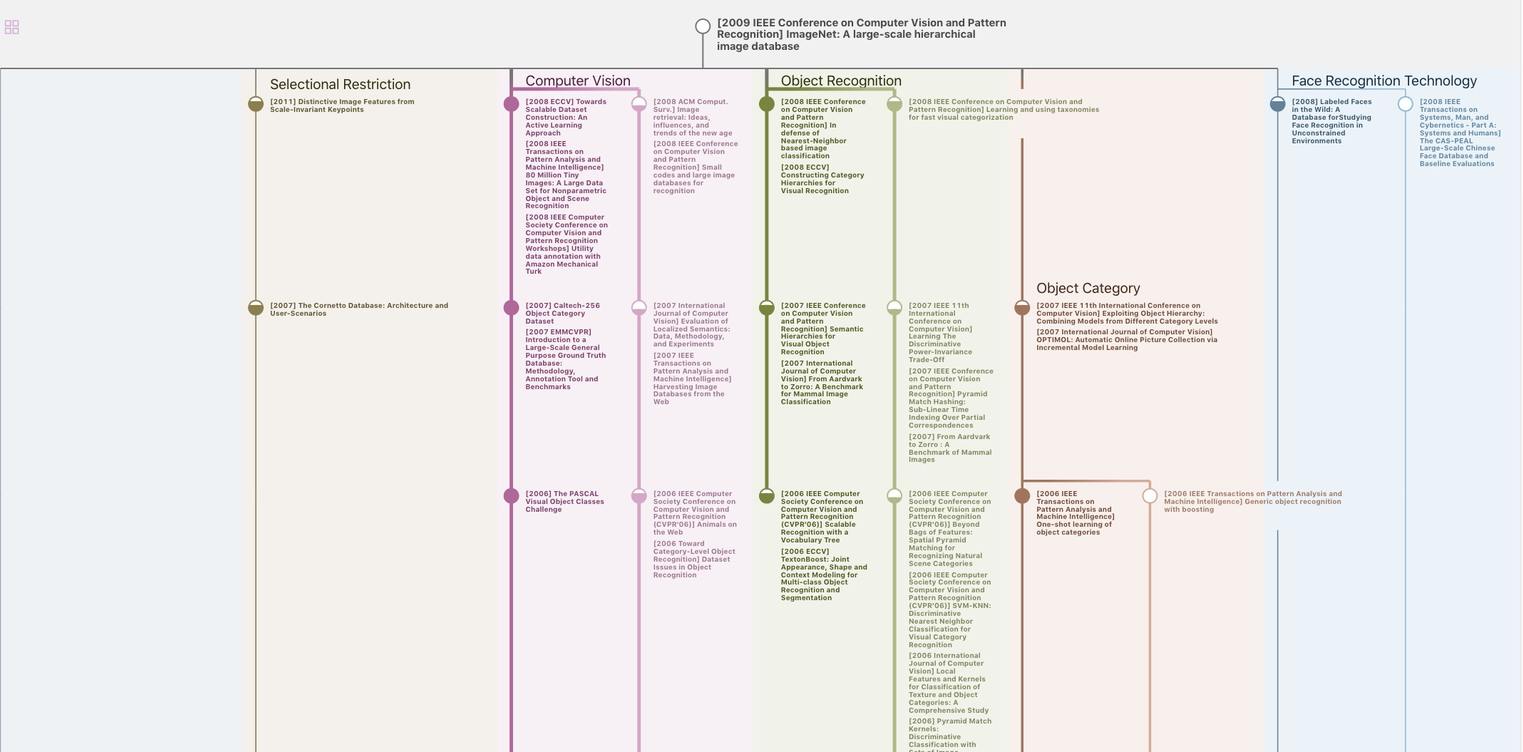
生成溯源树,研究论文发展脉络
Chat Paper
正在生成论文摘要