Enhancing spatial and temporal utilities in differentially private moving objects database release
INTERNATIONAL JOURNAL OF INFORMATION SECURITY(2020)
摘要
The pervasive use of mobile technologies and GPS-equipped vehicles has resulted in a large number of moving objects databases. Privacy protection is one of the most significant challenges related to moving objects databases because of the legal requirements in many application domains. Over the last few years, several differentially private mechanisms have been proposed for moving objects databases. However, most of them aim to answer statistical queries and do not release a differentially private version of a moving objects database. In this paper, we present DP-MODR, a differentially private (DP) mechanism for synthetic moving objects database release (MODR). DP-MODR tries to efficiently and effectively release synthetic trajectories while preserving spatial and temporal utilities. In this way, the released differentially private moving objects database can be used for different purposes as well, including data analysis tasks. DP-MODR keeps some main spatial and temporal properties of original trajectories and defines a new differentially private tree structure to keep the most probable paths with different lengths and different starting points, which are then iteratively joined to generate synthetic trajectories in a bottom-up way. Also, we present an extension of DP-MODR to support moving objects databases whose locations are time-dependent. Extensive experiments on real moving objects datasets using multiple spatial and temporal evaluation measures show that DP-MODR enhances the utility of query answers and better preserves the main spatial and temporal properties of original trajectories in comparison with recent related work.
更多查看译文
关键词
Differential privacy,Moving objects database release,Noisy cost-sensitive path tree,Spatial utility,Temporal utility,Time-dependent query
AI 理解论文
溯源树
样例
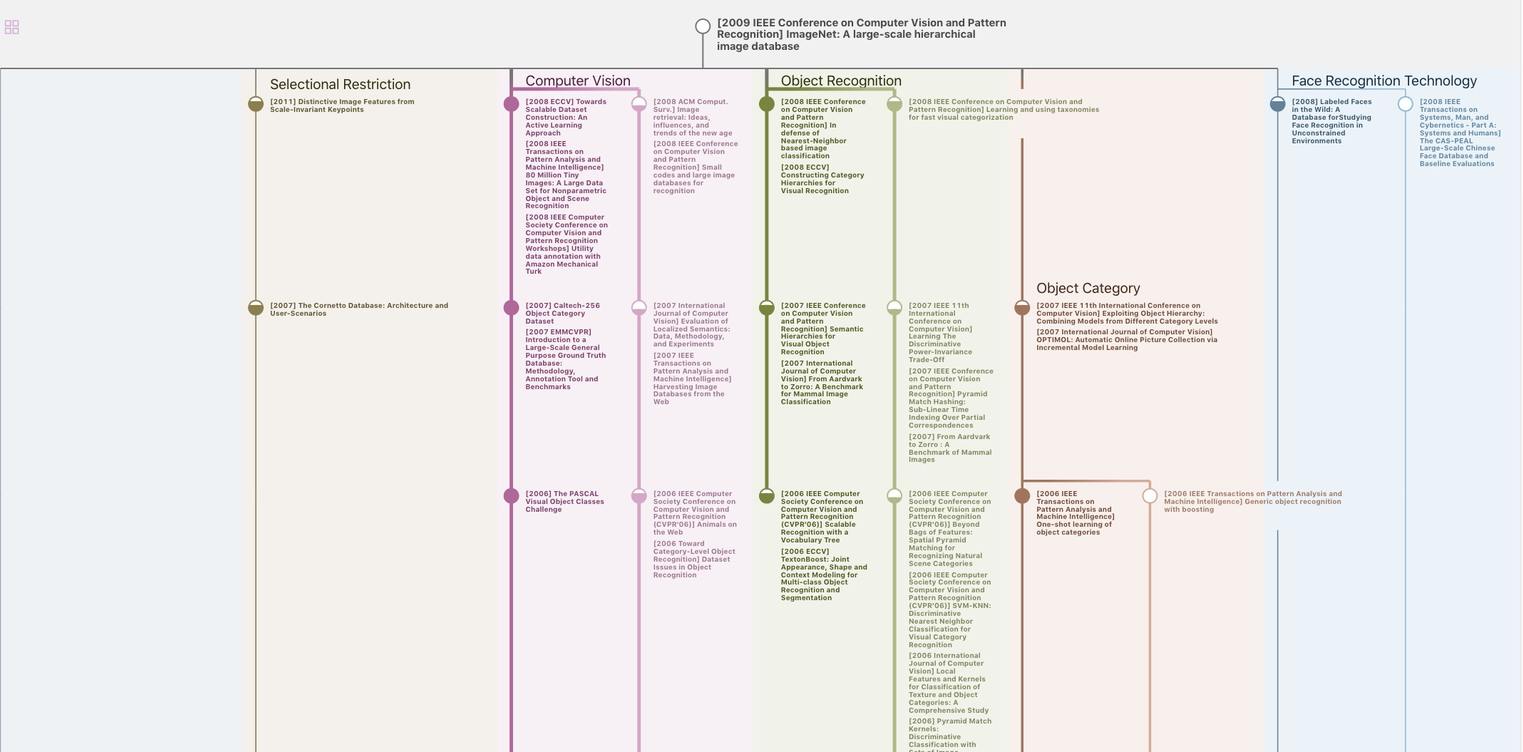
生成溯源树,研究论文发展脉络
Chat Paper
正在生成论文摘要